Software-Friendly Subjective Bayesian Networks: Reasoning within a Software-Centric Mission Impact Assessment Framework
FUSION(2023)
摘要
Subjective Bayesian networks (SBN) combine Bayesian Networks (BN) with Subjective Logic in order to express the second-order uncertainty (i.e., the uncertainty about a probability distribution of an event – as opposed to the uncertainty about the event itself). While SBNs provide a strong formalism for treating the uncertainty in a higher level, the literature lacks support for extensive software implementations focused on compatibility with current software solutions or standards. Our work explores the structural congruence between BN and SBN (in terms of software data structure) and a semantic bijection between Subjective Logic opinions to Dirichlet distributions to introduce a SBN reasoning framework that targets on effectively reusing the existing BN solutions. Particularly, we developed two inference algorithms that apply a Monte Carlo method to existing BN inference algorithms (we chose the Junction Tree algorithm, for test), respectively for batch and interactive SBN reasoning. A method for translating an evidence in SBN to uncertain (virtual) evidence in BN is also presented. The main contribution of this paper is the introduction of a simple, yet flexible empirical estimation method and a software architecture that virtually adapts any BN inference algorithm to an approximate computational inference framework for SBN. We also developed a Java component to demonstrate the reusability, and we developed a case study of Mission Impact Assessment of Unmanned Aerial Vehicles transporting critical items between hospitals in order to illustrate the applicability in a knowledge engineering process.
更多查看译文
关键词
Subjective Bayesian Networks,Second Order Uncertainty,Virtual Evidence,Monte Carlo method,Mission Impact Assessment,Unmanned Aerial Vehicle
AI 理解论文
溯源树
样例
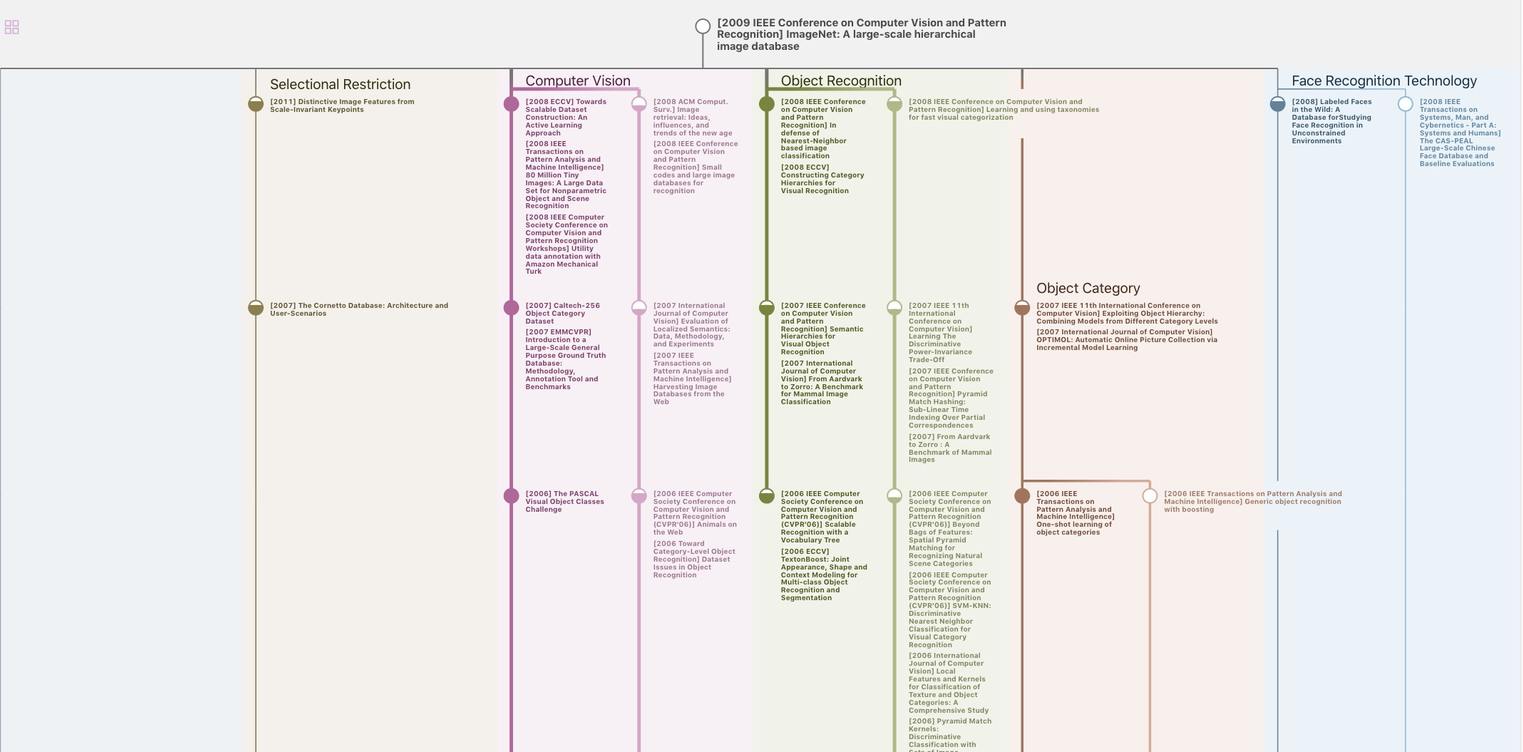
生成溯源树,研究论文发展脉络
Chat Paper
正在生成论文摘要