Conservative Data Reduction for Covariance Matrices Using Elementwise Event Triggers.
FUSION(2023)
摘要
Decentralized data fusion algorithms are fundamentally built on the exchange of estimates and covariance matrices between the individual components. This leads to a high volume of data, mainly caused by the covariance matrices, which can be problematic, especially in environments with limited bandwidth. In order to guarantee the proper functioning of decentralized estimation algorithms, data reduction methods for covariance matrices must ensure that the reduced matrices are conservative, i.e., do not underestimate the actual uncertainty. Motivated by these considerations, this paper presents an elementwise event-triggered method for the data-reduced transmission of covariance matrices that takes into account the aforementioned condition concerning uncertainty. For this purpose, several event triggers are proposed and, based on the event data and diagonal dominance, upper bounds for the actual covariance matrices are derived. An investigation of the data reduction and its influence on the estimation results is performed in a decentralized tracking scenario. The results show that substantial data reduction is possible with only minor losses in estimation quality.
更多查看译文
关键词
Decentralized Data Fusion,Data Reduction,Conservative Data Fusion
AI 理解论文
溯源树
样例
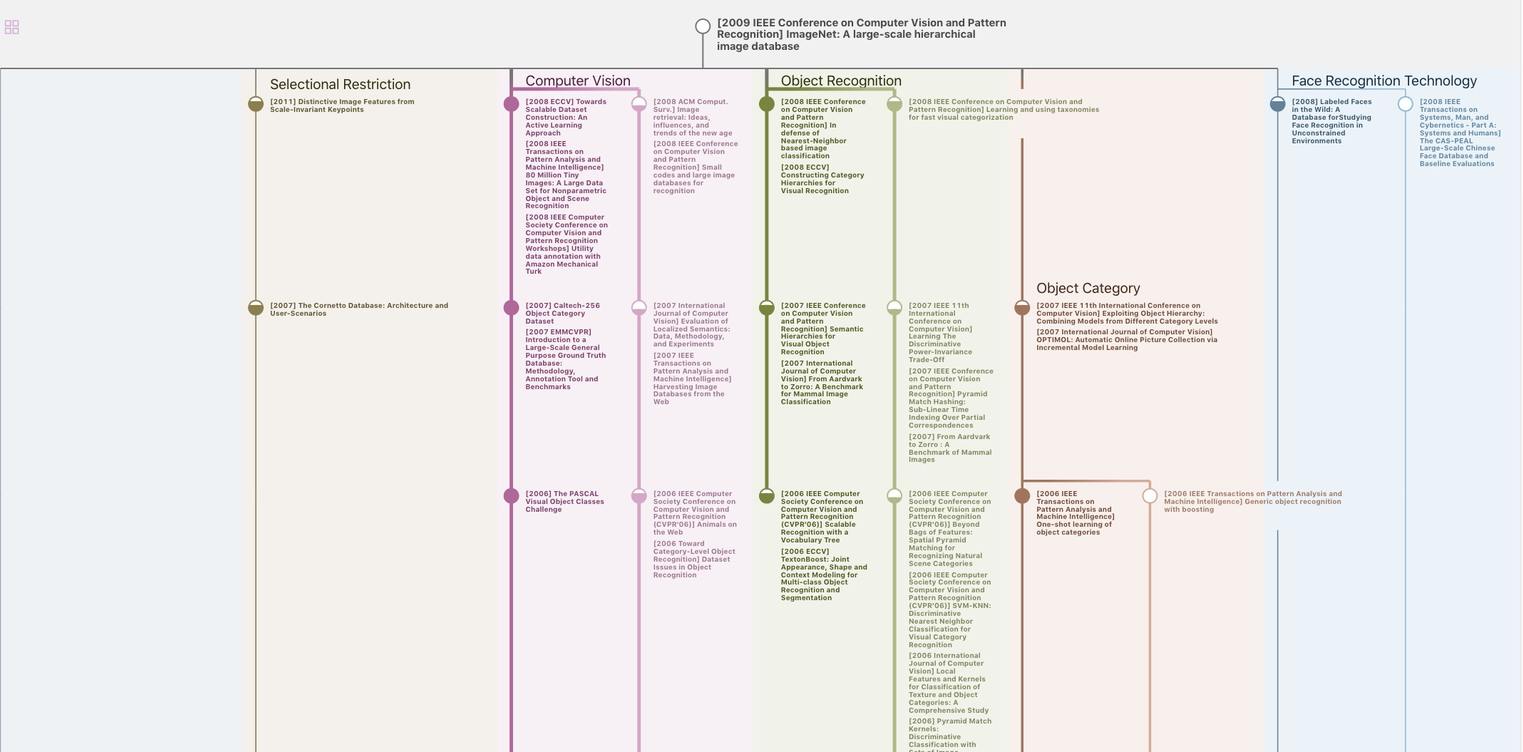
生成溯源树,研究论文发展脉络
Chat Paper
正在生成论文摘要