TraceNet: Tracing and Locating the Key Elements in Sentiment Analysis.
Knowledge-based systems(2023)
摘要
We study sentiment analysis task where the outcomes are mainly contributed by a few key elements of the inputs. Motivated by the two-streams hypothesis, we explore processing input items and their weights separately by developing a neural architecture, named TraceNet, to address this type of task. It not only learns discriminative representations for the target task via its encoders, but also traces key elements at the same time via its locators. In TraceNet, both encoders and locators are organized in a layer-wise manner, and a smoothness regularization is employed between adjacent encoder-locator combinations. Moreover, a sparsity constraint is enforced on locators for tracing purposes and items are proactively masked according to the item weights output by locators. A major advantage of TraceNet is that the outcomes are easier to understand, as it identifies the key components responsible for the outcomes, making them easier to understand. The experimental results demonstrate its effectiveness in sentiment classification. Furthermore, we present case studies to showcase the interpretability of the model and conduct comprehensive analyses to highlight the impacts of each component. (c) 2023 Elsevier B.V. All rights reserved.
更多查看译文
关键词
Language models,Sentiment analysis,Key items,Interpretability
AI 理解论文
溯源树
样例
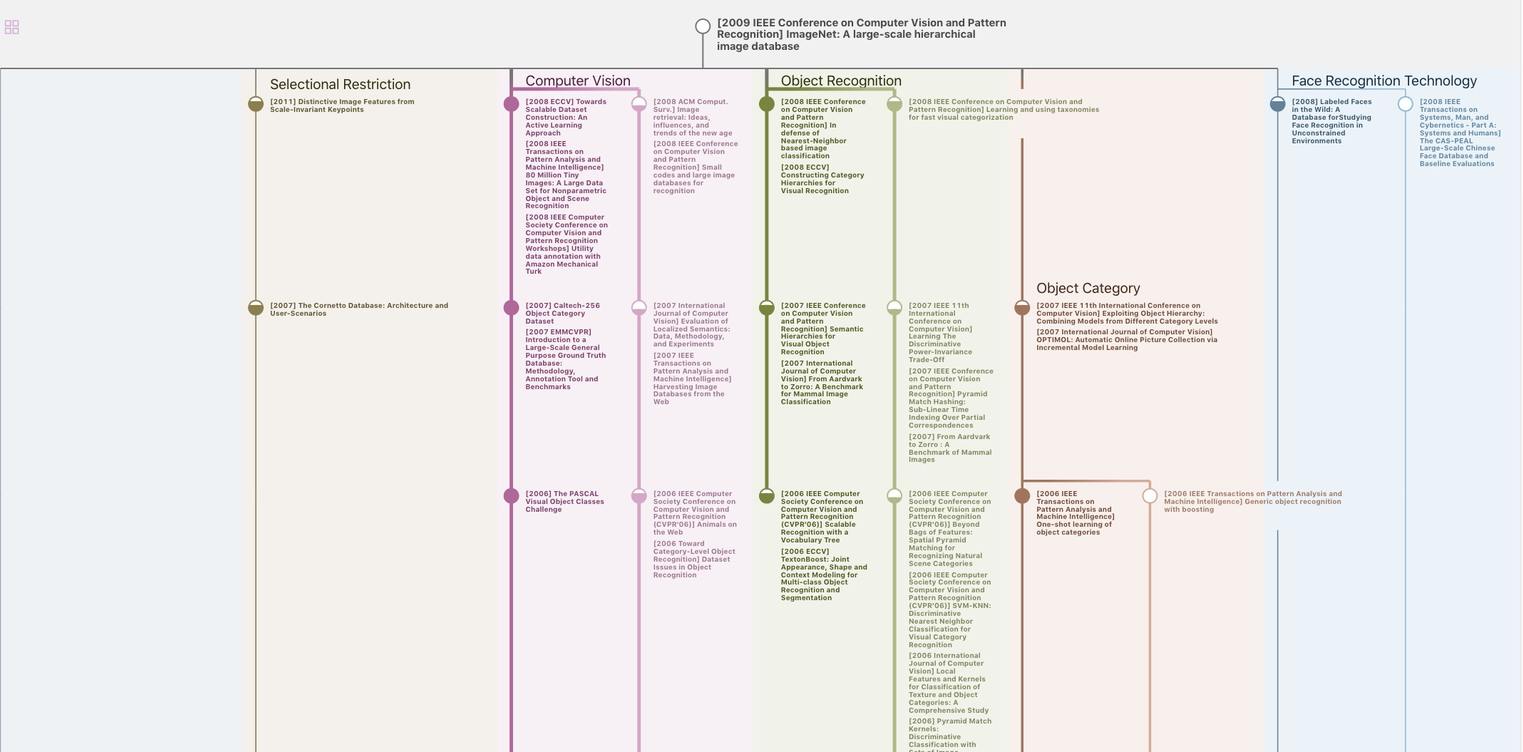
生成溯源树,研究论文发展脉络
Chat Paper
正在生成论文摘要