Multi-dataset fusion for multi-task learning on face attribute recognition
Pattern Recognit. Lett.(2023)
摘要
The goal of face attribute recognition(FAR) is to recognize the attributes of face images, such as gender, race, etc. Multi-dataset fusion aims to train a network with multiple datasets simultaneously, which has significant advantages in deep learning. For example, in FAR, the attribute labels differ with the datasets. When using multi-dataset fusion on FAR, training data and attribute labels can be increased so that the network performance can be improved and more attributes can be recognized simultaneously. Currently, most related researchers take the multi-task learning approach on FAR, where each attribute is treated as a recognition task. However, the existing multi-task learning framework cannot be applied when using multi-dataset fusion on FAR. That is because multi-task learning requires the training data to contain the labels of all tasks, but no data contains all the attribute labels of multiple datasets. A Multi-Dataset and Multi-Task Framework(MDMTF) that takes knowledge distillation as the core is proposed in this paper to deal with the problem above. Three distillation strategies are designed in the MDMTF according to the characteristics of multi-dataset fusion on FAR. Experimental results on CelebA and MAAD-Face datasets demonstrate the effectiveness of our framework and strategies. Compared to training two networks on both datasets, respectively, that is, single dataset training, our method has significant advantages in accuracy, parameters, and computational complexity.
更多查看译文
关键词
Face attribute recognition,Multi-dataset fusion,Multi-task learning,Knowledge distillation,Deep learning
AI 理解论文
溯源树
样例
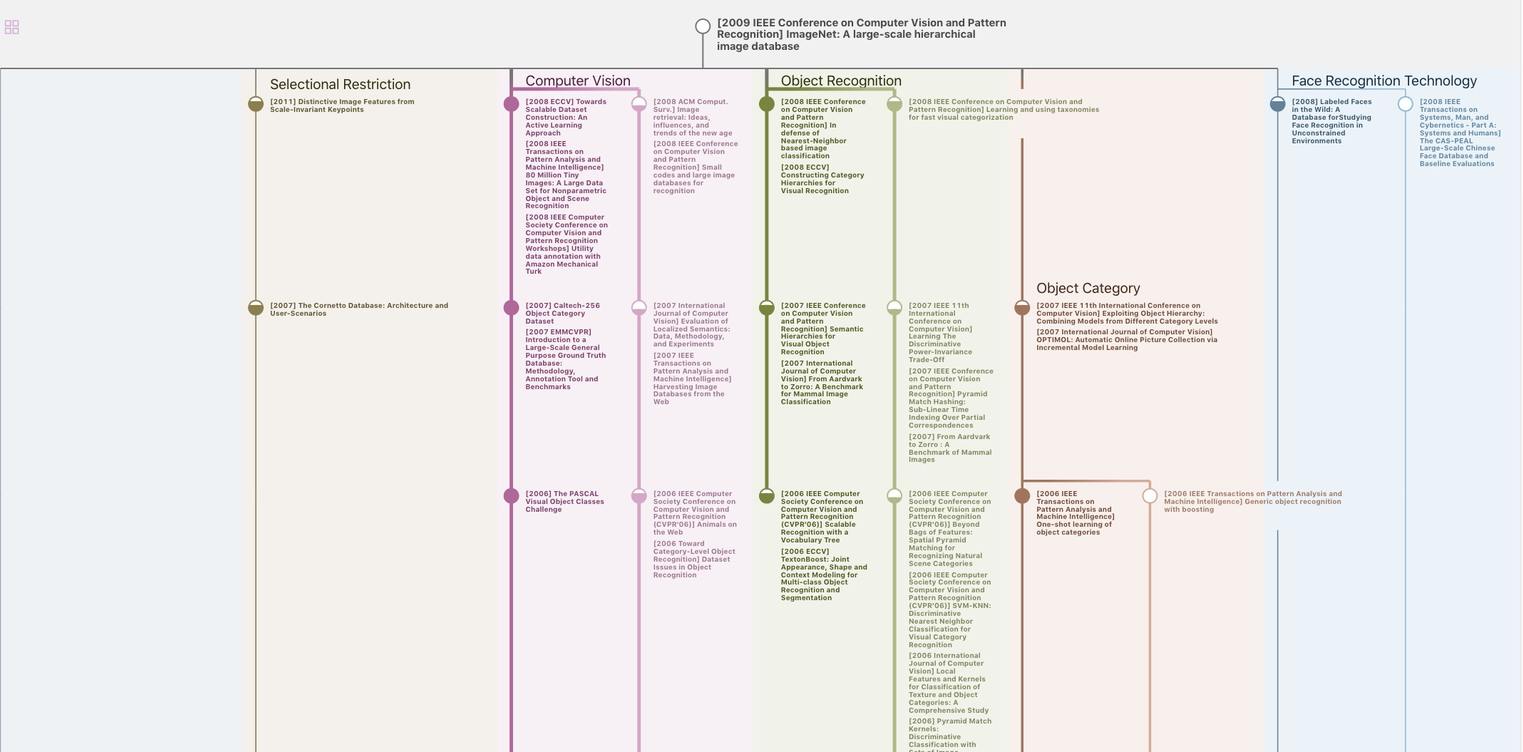
生成溯源树,研究论文发展脉络
Chat Paper
正在生成论文摘要