Instance-dimension dual contrastive learning of visual representations
Mach. Vis. Appl.(2023)
摘要
Existing contrastive methods usually learn visual representations either by maximizing instance contrast or by minimizing dimension redundancy separately, and fail to make full use of data information. In this paper, we propose an instance-dimension dual contrastive method named IDDCLR to thoroughly mine the intrinsic knowledge underlying data. It jointly optimizes the instance contrast and the dimension redundancy to learn better visual representations. Specifically, we employ the normalized temperature scaled cross entropy (NT-Xent) to formulate the instance contrast loss, and propose a dimension contrast loss function that also takes the form of NT-Xent, resulting in symmetric form of the whole loss. The significance of minimizing the loss is twofold: On the one hand, it learns effective visual representations in the latent space, where the agreement between differently augmented views of the same instance is maximized. On the other hand, it minimizes the redundancy among feature dimensions, consequently being capable of avoiding trivial embeddings. Experimental results show that IDDCLR outperforms state-of-the-art self-supervised methods on classification tasks, and performs comparably on transfer learning tasks.
更多查看译文
关键词
Contrastive learning, Self-supervised learning, Dual contrastive, Visual representation
AI 理解论文
溯源树
样例
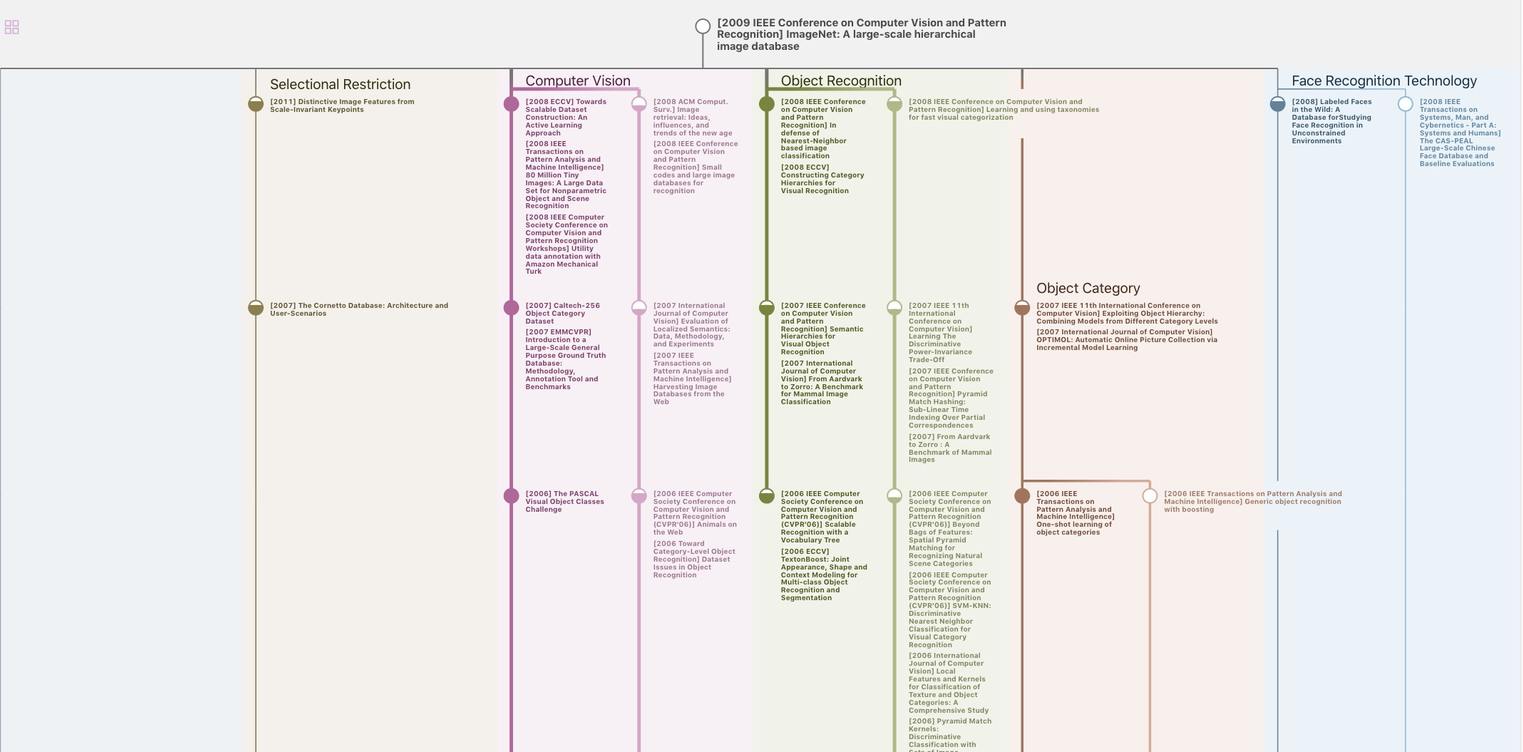
生成溯源树,研究论文发展脉络
Chat Paper
正在生成论文摘要