A novel sample selection approach based universal unsupervised domain adaptation for fault diagnosis of rotating machinery.
Reliab. Eng. Syst. Saf.(2023)
摘要
Transfer learning-based fault diagnosis methods, especially unsupervised domain adaptation (UDA), have demonstrated significant potential in addressing insufficiently labeled signal problems. However, the assumption that the label spaces of two domains are identical may only be valid in some real-world scenarios. A priori information about the target domain's failure modes is usually unavailable in natural industries, limiting UDA's applicability. In this paper, a more common UDA scenario, called universal UDA (UUDA), is designed to handle domain and label space shift issues better, where no explicit assumption is made on the target label set. Furthermore, we propose a novel sample selection method to address the UUDA problem. Firstly, the outlier threshold learning aims to minimize the distance between known classes in the source domain while preserving the discrepancy between known and outlier classes. Subsequently, the domain-invariant sampler performs domain-invariant feature sampling while accommodating label space shifts. Lastly, an adversarial classifier training method is incorporated to enhance transferability by recognizing label space variability across domains. Extensive experiments have demonstrated exceptional performance in addressing domain and label space inconsistencies.
更多查看译文
关键词
Machinery fault diagnosis,Transfer learning,Deep learning,Unsupervised domain adaptation,Universal unsupervised domain adaptation
AI 理解论文
溯源树
样例
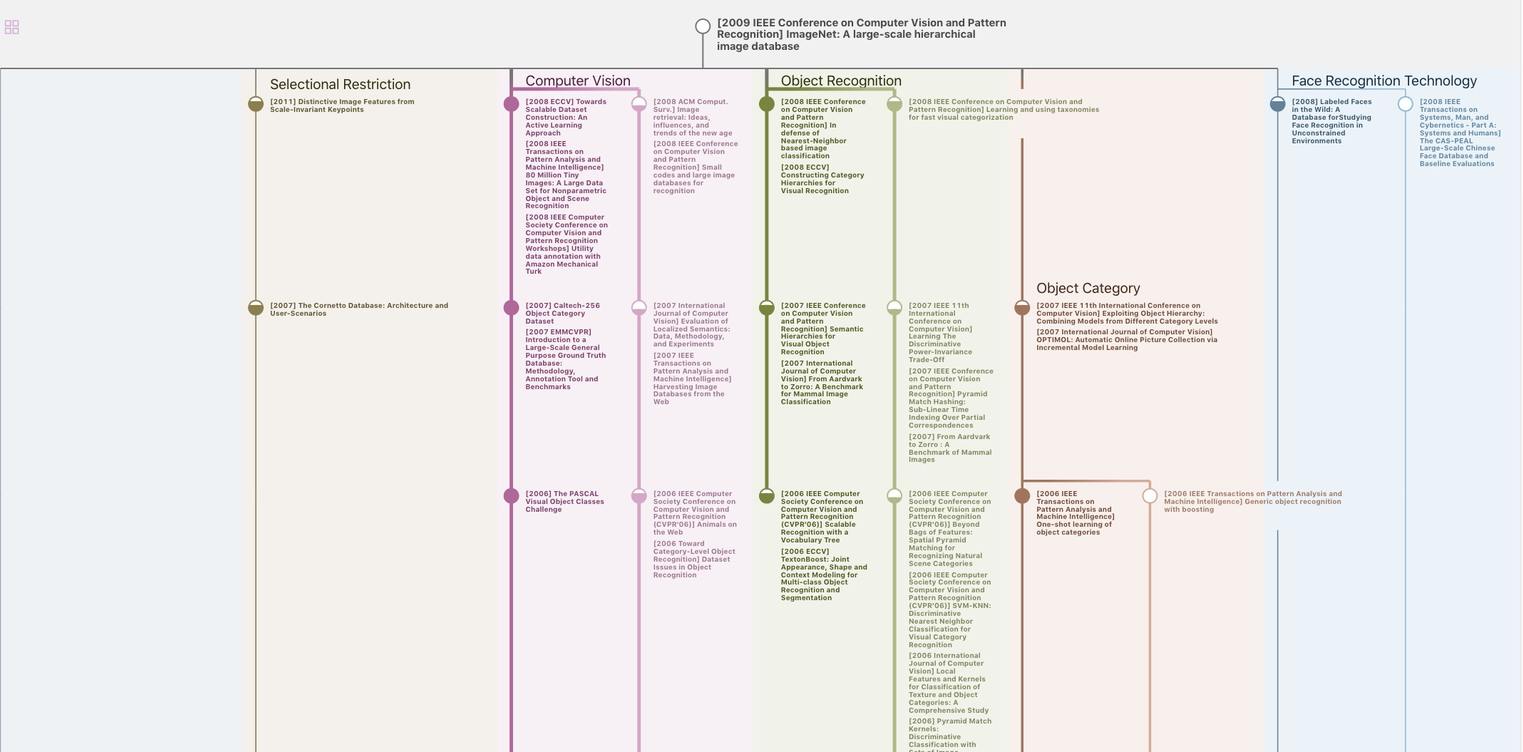
生成溯源树,研究论文发展脉络
Chat Paper
正在生成论文摘要