GNSS-R Global Sea Surface Wind Speed Retrieval Based on Deep Learning
IEEE Trans. Geosci. Remote. Sens.(2023)
摘要
Global navigation satellite system reflectometry (GNSS-R) is a burgeoning remote sensing observation technology that can retrieve global sea surface wind speeds using satellite signals reflected from the sea surface. The improvement of data quality and the accumulation of data volume of this technology provides data support for constructing interdisciplinary-based retrieval models. This article constructs a hybrid deep neural network model based on deep learning for wind speed retrieval, which can receive and perform feature mining on the entire delay waveform while simultaneously supporting multiple auxiliary features input and achieving joint fitting. Then a bias correction method based on cumulative distribution function (CDF) matching is introduced to mitigate bias, especially at high wind speeds. We verify the contribution of different attribute features in wind speed retrieval by designing a feature ablation analysis. The fluctuation variation of the retrieval accuracy in the time dimension and the retrieval results distribution in space are compared and analyzed. The root mean square error (RMSE) of retrieval results is 1.486 m/s and can reach 1.399 m/s under the 94.25% wind speed condition. After bias correction based on CDF matching, the retrieval accuracy at high wind speed is improved by 7.19%. Besides, this model also has good temporal stability and can reproduce large-scale wind fields while effectively mitigating retrieval bias on a global scale, showing great potential for operational applications.
更多查看译文
关键词
Cyclone global navigation satellite system (CYGNSS),deep learning,global navigation satellite system reflectometry (GNSS-R),sea surface wind speed
AI 理解论文
溯源树
样例
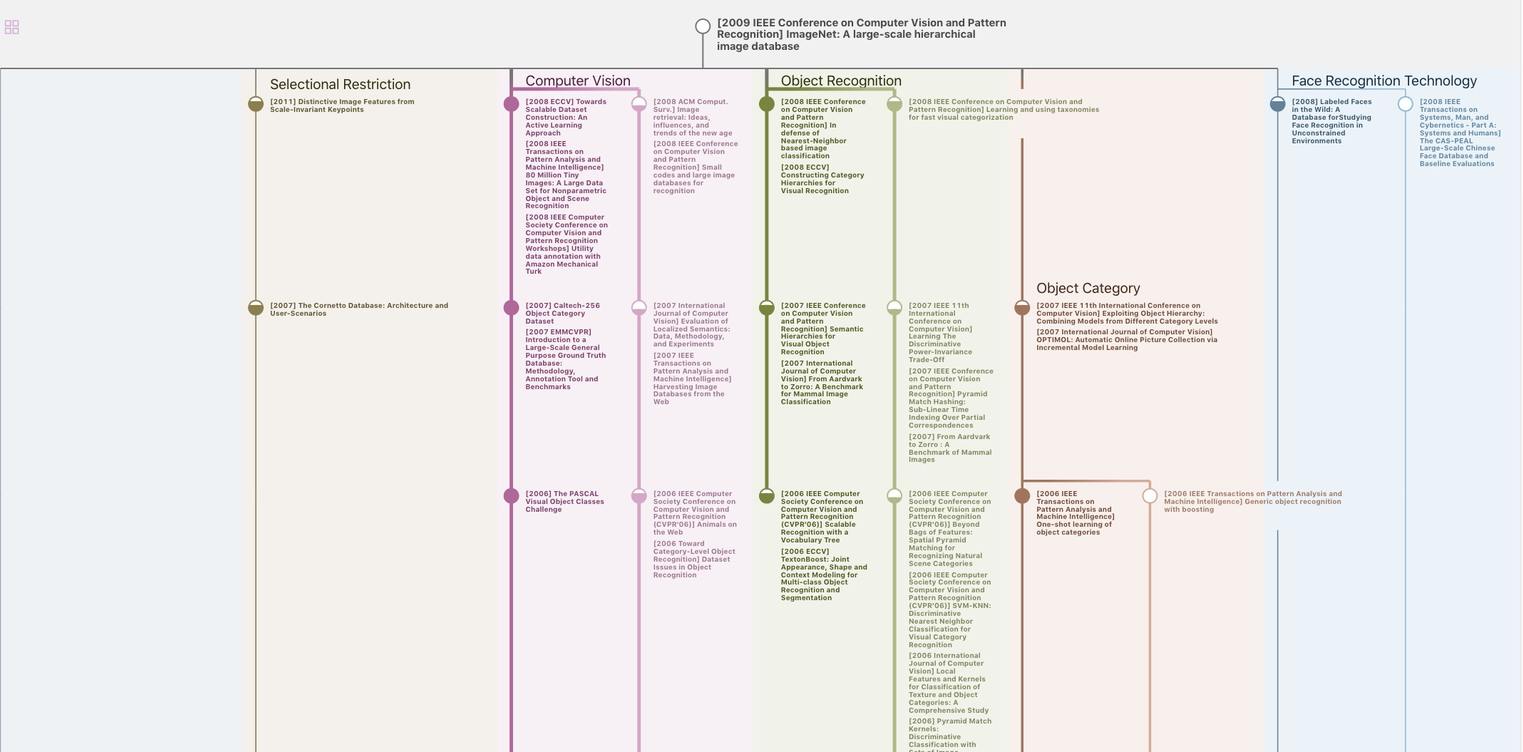
生成溯源树,研究论文发展脉络
Chat Paper
正在生成论文摘要