Large Kernel Spectral and Spatial Attention Networks for Hyperspectral Image Classification.
IEEE Trans. Geosci. Remote. Sens.(2023)
摘要
Currently, long-range spectral and spatial dependencies have been widely demonstrated to be essential for hyperspectral image (HSI) classification. Due to the transformer superior ability to exploit long-range representations, the transformer-based methods have exhibited enormous potential. However, existing transformer-based approaches still face two crucial issues that hinder the further performance promotion of HSI classification: 1) treating HSI as 1-D sequences neglects spatial properties of HSI and 2) the dependence between spectral and spatial information is not fully considered. To tackle the above problems, a large kernel spectral-spatial attention network (LKSSAN) is proposed to capture the long-range 3-D properties of HSI, which is inspired by the visual attention network (VAN). Specifically, a spectral-spatial attention module (SSAM) is first proposed to effectively exploit discriminative 3-D spectral-spatial features while keeping the 3-D structure of HSI. This module introduces the large kernel attention (LKA) and convolutional feed-forward (CFF) to flexibly emphasize, model, and exploit the long-range 3-D feature dependencies with lower computational pressure. Finally, the features from the SSAM are fed into the classification module for the optimization of 3-D spectral-spatial representation. To verify the effectiveness of the proposed classification method, experiments are executed on four widely used HSI datasets. The experiments demonstrate that LKSSAN is indeed an effective way for long-range 3-D feature extraction of HSI.
更多查看译文
关键词
Feature extraction, Three-dimensional displays, Transformers, Kernel, Data mining, Correlation, Convolution, Convolutional feed-forward (CFF), deep learning (DL), hyperspectral image (HSI) classification, large kernel attention (LKA), long-range 3-D spectral-spatial feature extraction, spectral-spatial attention
AI 理解论文
溯源树
样例
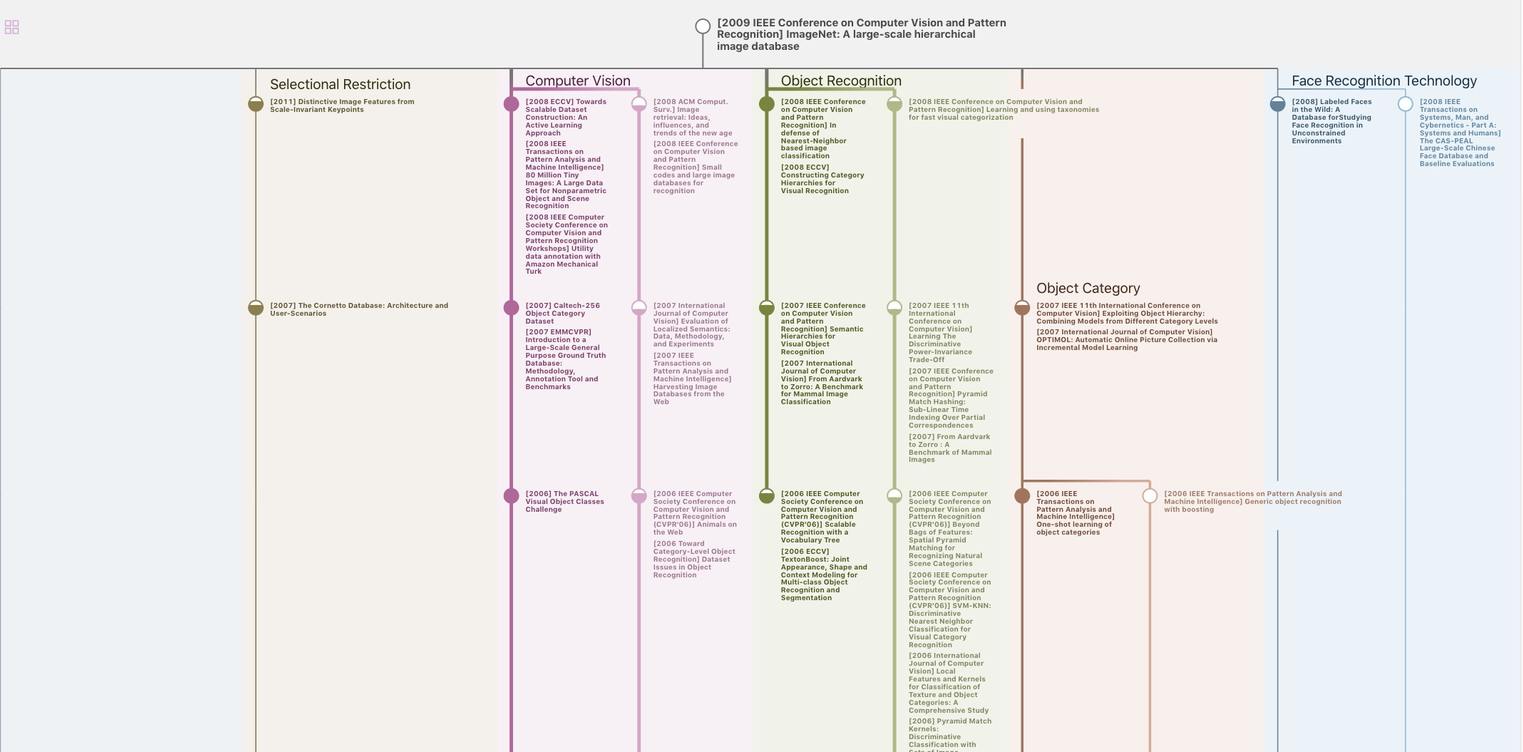
生成溯源树,研究论文发展脉络
Chat Paper
正在生成论文摘要