Urban Building Classification (UBC) V2 - A Benchmark for Global Building Detection and Fine-Grained Classification From Satellite Imagery.
IEEE Trans. Geosci. Remote. Sens.(2023)
摘要
Datasets play a key role in developing superior building detection approaches. However, most of the previous work focuses on accurate building masks and scale expansion, while the categories are always missing, which hinders the further analysis of urban development and cultures. Therefore, we propose a benchmark for building detection and fine-grained classification from very high-resolution (VHR) satellite imagery. An extensive annotation is performed for about 0.5 million building instances with 12 fine-grained roof types and individual polygons. The annotation of building functions of two cities in the previous version (UBCv1) (Huang et al., 2022) is also integrated. To ensure the building variety, it consists of VHR optical images of 20 unique cities worldwide with various landforms and styles of architecture. Its variety and fine-grained categories pose great challenges and meanwhile provide a foundation for the building extraction and fine-grained classification on a global scale. Besides, 17 cities are provided with finely aligned synthetic aperture radar (SAR) images, which can be used for the development and evaluation of approaches optionally based on optical, SAR, or multimodal images. Significantly, the proposed benchmark is used as the base of the 2023 IEEE GRSS Data Fusion Contest (Persello et al., 2023). The dataset and codes of the baseline methods are available at: https://github.com/AICyberTeam/UBC-dataset/tree/UBCv2.
更多查看译文
关键词
Building detection,fine-grained classification,instance segmentation,multimodal,optical imagery,synthetic aperture radar (SAR)
AI 理解论文
溯源树
样例
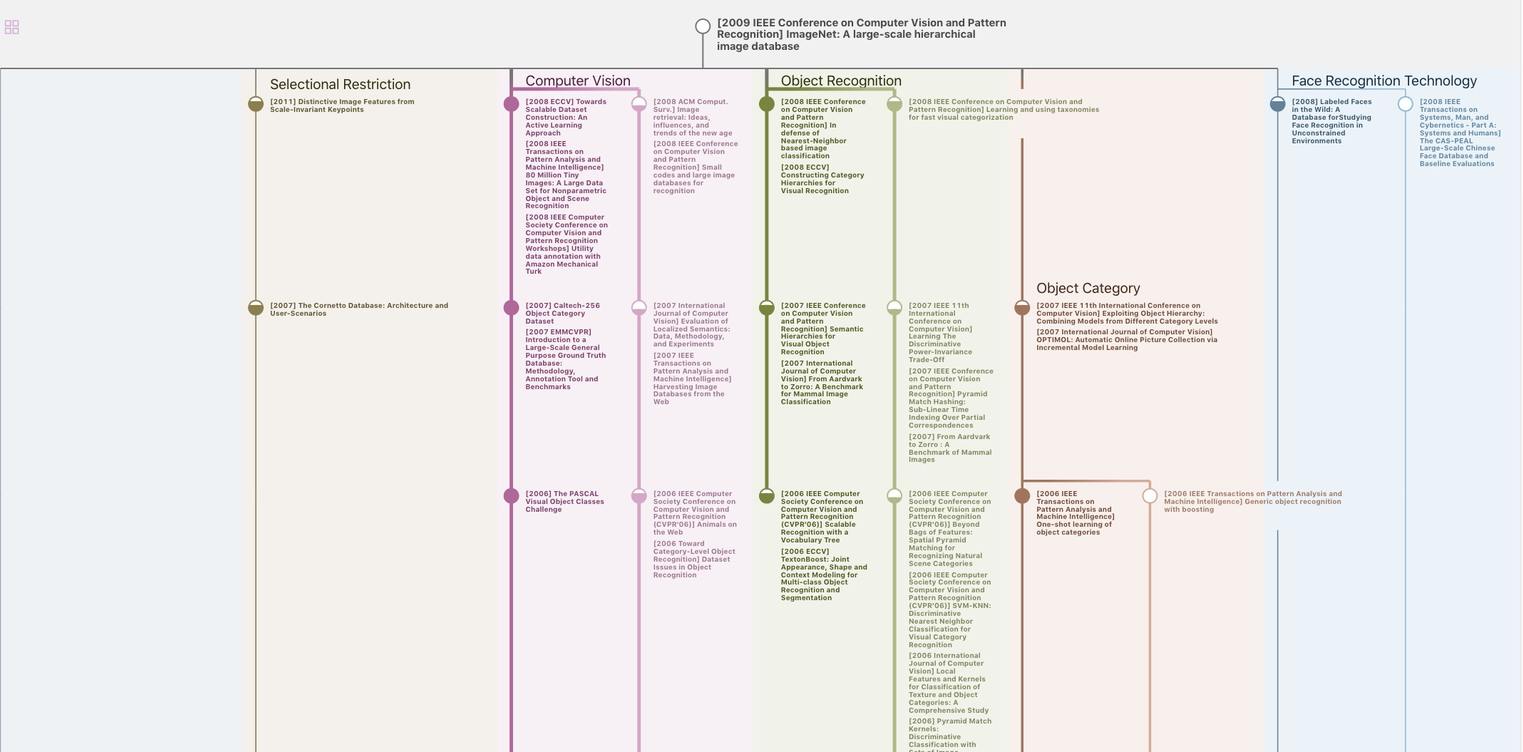
生成溯源树,研究论文发展脉络
Chat Paper
正在生成论文摘要