Multitask Learning for SAR Ship Detection With Gaussian-Mask Joint Segmentation.
IEEE Trans. Geosci. Remote. Sens.(2023)
摘要
Detecting ships from synthetic aperture radar (SAR) images is inherently subject to the limitations of SAR's imaging mechanism. SAR object detection technology has rapidly advanced in recent years due to deep-learning-based techniques for detecting objects from optical images. SAR ship detection still faces some challenges due to the strong speckle noise, complex surroundings, and a variety of scales. This article proposes a multitask learning framework for object detection (MLDet) detect ships in SAR images. The proposed end-to-end framework consists of the object detection task, speckle suppression task, and target segmentation task. First, an angle classification loss with aspect ratio weighting (ARW) is explored during object detection to improve the accuracy by making the detector sensitive to the periodicity of angular and the aspect ratio of objects. Second, the speckle suppression task uses a dual-feature fusion attention (DFA) mechanism to suppress noisy background information and fuse shallow features and denoising features, which helps MLDet be more robust to speckle noise. Third, the target segmentation task with rotated Gaussian mask is explored to further help the detection network to extract the regions of intersect from the cluttered background, as well as improving the detection efficiency through pixel-by-pixel prediction. The rotated Gaussian mask for ship modeling ensures that the center of a ship has the highest probabilities to be labeled as an object, and the probabilities of the remaining regions are gradually reduced under a Gaussian distribution. Assisted by these two subtasks, the shallow-level features are robust to speckle noise and reliably support deep-level feature learning. In addition, the weighted rotated boxes' fusion (WRBF) strategy is adopted to combine the predictions of multidirection anchors for rotated objects and eliminate the anchors beyond the boundary and the anchors with high overlap rates but low scores. A large number of experiments and comprehensive evaluations on the SAR ship detection datasets SSDD+ and HRSID have shown the effectiveness and superiority of the proposed method.
更多查看译文
关键词
Multitask learning,ship detection,synthetic aperture radar (SAR)
AI 理解论文
溯源树
样例
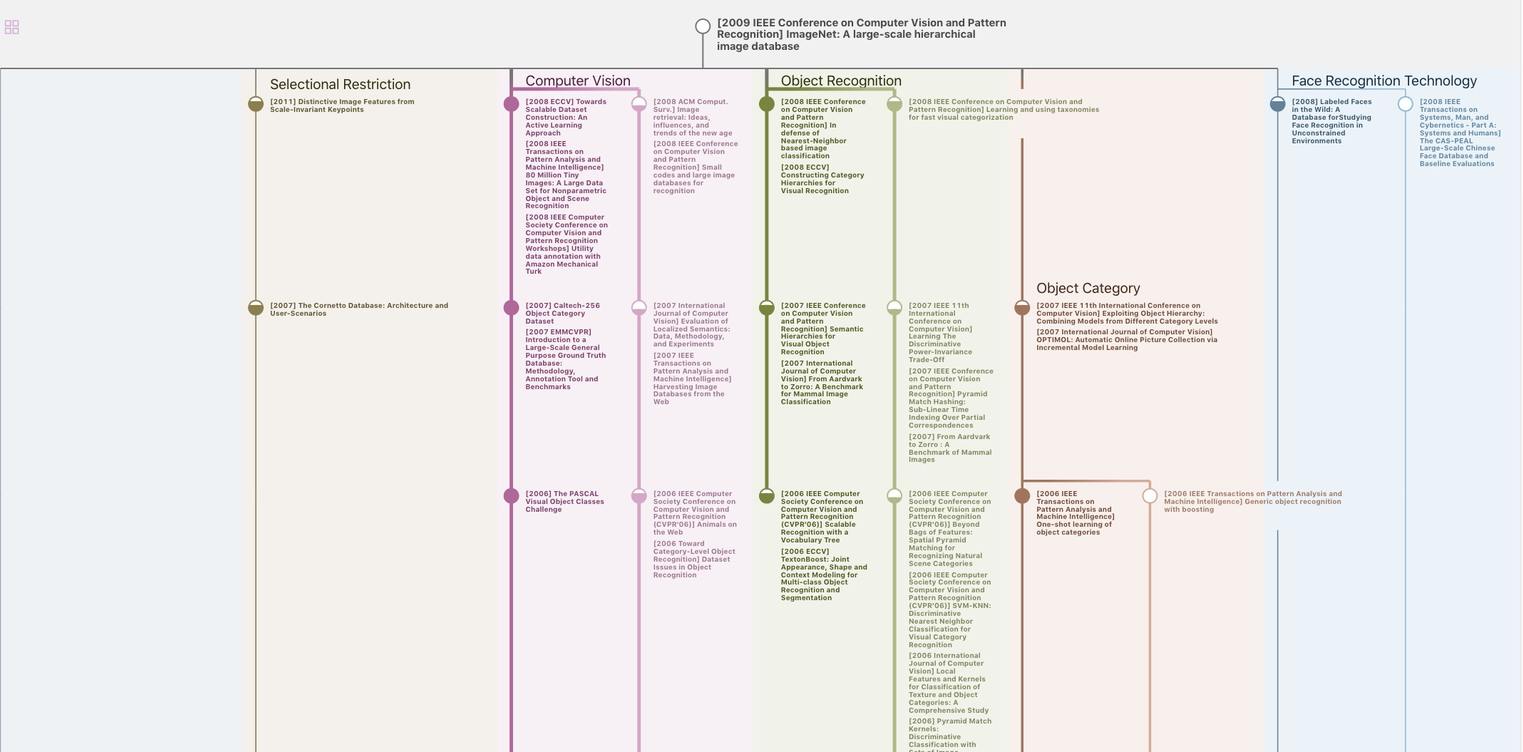
生成溯源树,研究论文发展脉络
Chat Paper
正在生成论文摘要