InSAR Spatial-Heterogeneity Tropospheric Delay Correction in Steep Mountainous Areas Based on Deep Learning for Landslides Monitoring.
IEEE Trans. Geosci. Remote. Sens.(2023)
摘要
Synthetic aperture radar interferometry (InSAR) technology has been widely used for landslide monitoring in mountainous areas. The troposphere in steep mountainous areas is affected by the variable topography, temperature, and humidity, which differs from that in plain areas and thus exhibits large spatial heterogeneity. Traditional InSAR troposphere correction methods are limited in this area, and the accuracy of InSAR measurements will be significantly affected. In this article, we proposed a tropospheric delay correction method based on deep learning (AtmNet) without external data considering the spatial heterogeneity in each individual interferogram. The tropospheric correction and landslide monitoring based on Sentinel-1 SAR data was carried out in Mao County, a high landslide-prone area in southwest Sichuan Province (China). A simulation experiment was conducted to analyze the adaptability of the model and evaluate the effectiveness of the AtmNet method. Furthermore, we demonstrated the good performance of the AtmNet method through a comparison with the linear model (LM) and generic atmospheric correction model (GACOS), revealing that the proposed method could effectively model the spatial heterogeneity of tropospheric delay in steep mountains. The slope displacements that cannot be seen in the interferogram were very clear after the tropospheric delay correction. This method provides important technical support for the accurate DInSAR and time-series InSAR for landslide monitoring in steep mountainous areas in the future.
更多查看译文
关键词
Deep learning, InSAR, landslides, mountainous areas, spatial heterogeneity, tropospheric delay
AI 理解论文
溯源树
样例
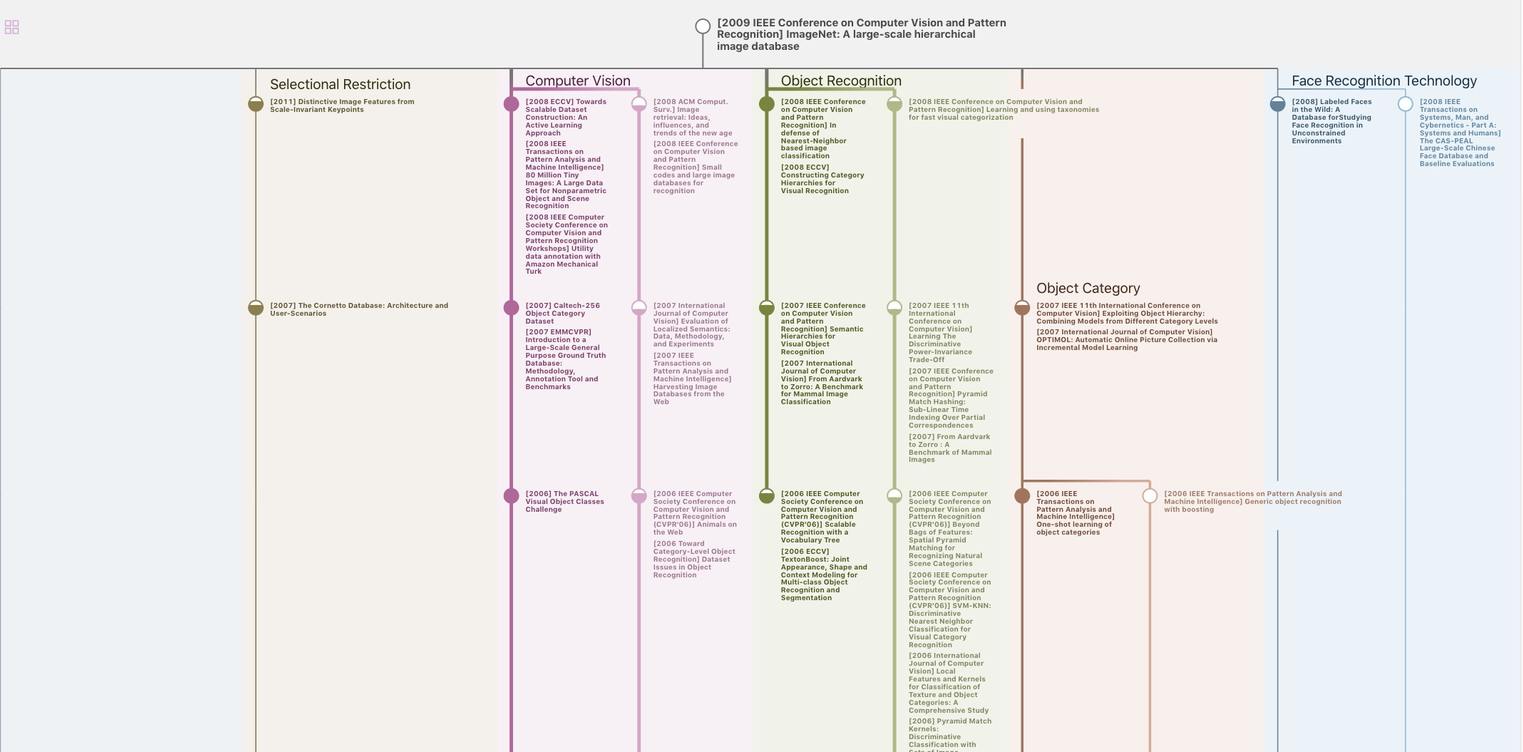
生成溯源树,研究论文发展脉络
Chat Paper
正在生成论文摘要