Class-Prompting Transformer for Incremental Semantic Segmentation.
IEEE Access(2023)
摘要
Class-incremental Semantic Segmentation (CISS) aims to learn new tasks sequentially that assign a specific category to each pixel of a given image while preserving the original capability to segment the old classes even if the labels of old tasks are absent. Most existing CISS methods suppress catastrophic forgetting by directly distilling on specific layers, which ignores the semantic gap between training data from the old and new classes with different distributions and leads to distillation errors, thus affecting segmentation performance. In this paper, we propose a Class-prompting Transformer (CPT) to introduce external prior knowledge provided by a pre-trained vision-language encoder into CISS pipelines for bridging the old and new classes and performing more generalized initialization and distillation. Specifically, we proposed a Prompt-guided Initialization Module (PIM), which measures the relationships between the class prompts and old query parameters to initialize the new query parameters for relocating the previous knowledge to the learning of new tasks. Then, a Semantic-aligned Distillation Module (SDM) is proposed to incorporate class prompt information with the class-aware embeddings extracted from the decoder to prevent the semantic gap problem between distinct class data and conduct adaptive knowledge transfer to suppress catastrophic forgetting. Extensive experiments on Pascal VOC and ADE20K datasets for the CISS task demonstrate the superiority of the proposed method, which achieves state-of-the-art performance.
更多查看译文
关键词
incremental semantic segmentation,semantic segmentation,transformer,class-prompting
AI 理解论文
溯源树
样例
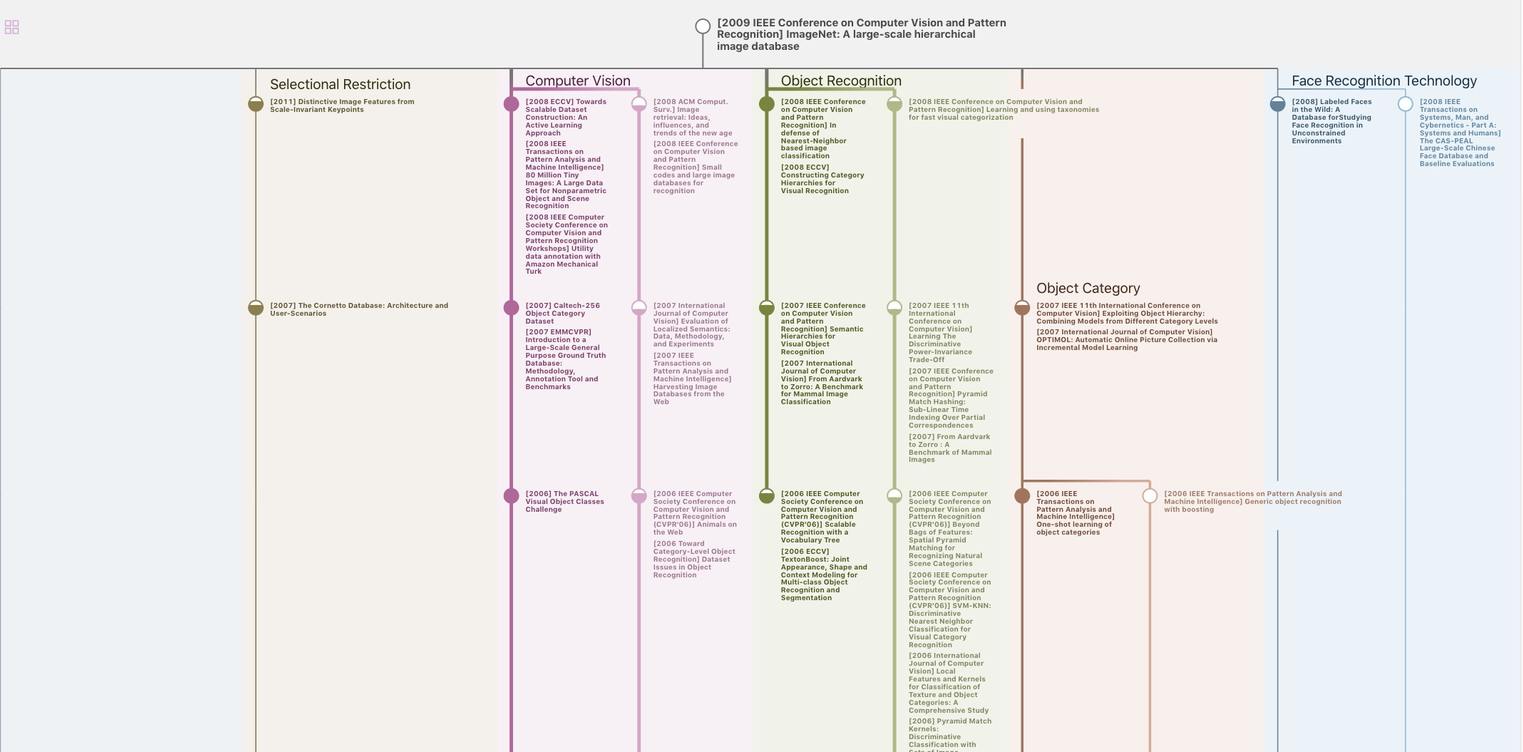
生成溯源树,研究论文发展脉络
Chat Paper
正在生成论文摘要