Improving Recommender Systems by a Further Factorization of the Factor Matrices
IEEE Access(2023)
摘要
Due to the availability of massive numbers of items for any product on theWeb, the burden of selecting an item is borne by the user. A Recommender System (RS) is a useful tool that has been employed to save the user's time by recommending preferred items for him/her efficiently. Collaborative-based RS's use the latent factor or/and the neighbourhood techniques including matrix factorization (MF), which is an efficient approach utilizing latent factors. The idea of the approach is based on calculating similarities through between users and items simultaneously, to predict appropriate recommendations. MF can be seen as mathematical model capable to split an entity into multiple smaller entries through an ordered rectangular array of functions, to discover the features or information underlying the interactions between users and items. The technique factorizes the user-item rating matrix (R) (first-level factorization) into users (P) and items (Q) matrices. A recent RS system, named NLM, combines the neighbourhood and the latent factor techniques to produce attractive results. This paper proposes to improve the NLM method by proposing a more effective technique, named NLM+, by further factorizing P and Q using MF (second-level factorization). To the best of our knowledge, current studies in this field have not considered the use of the aforementioned two levels of factorizing. To validate our method, CiaoDVD, MovieLens, and FilmTrust datasets have been used and incorporated to demonstrate that NLM+ improves NLM by 48% and 42% in the recall and the precision values, respectively.
更多查看译文
关键词
~Recommender system,matrix factorization,second-level factorization,latent factor techniques
AI 理解论文
溯源树
样例
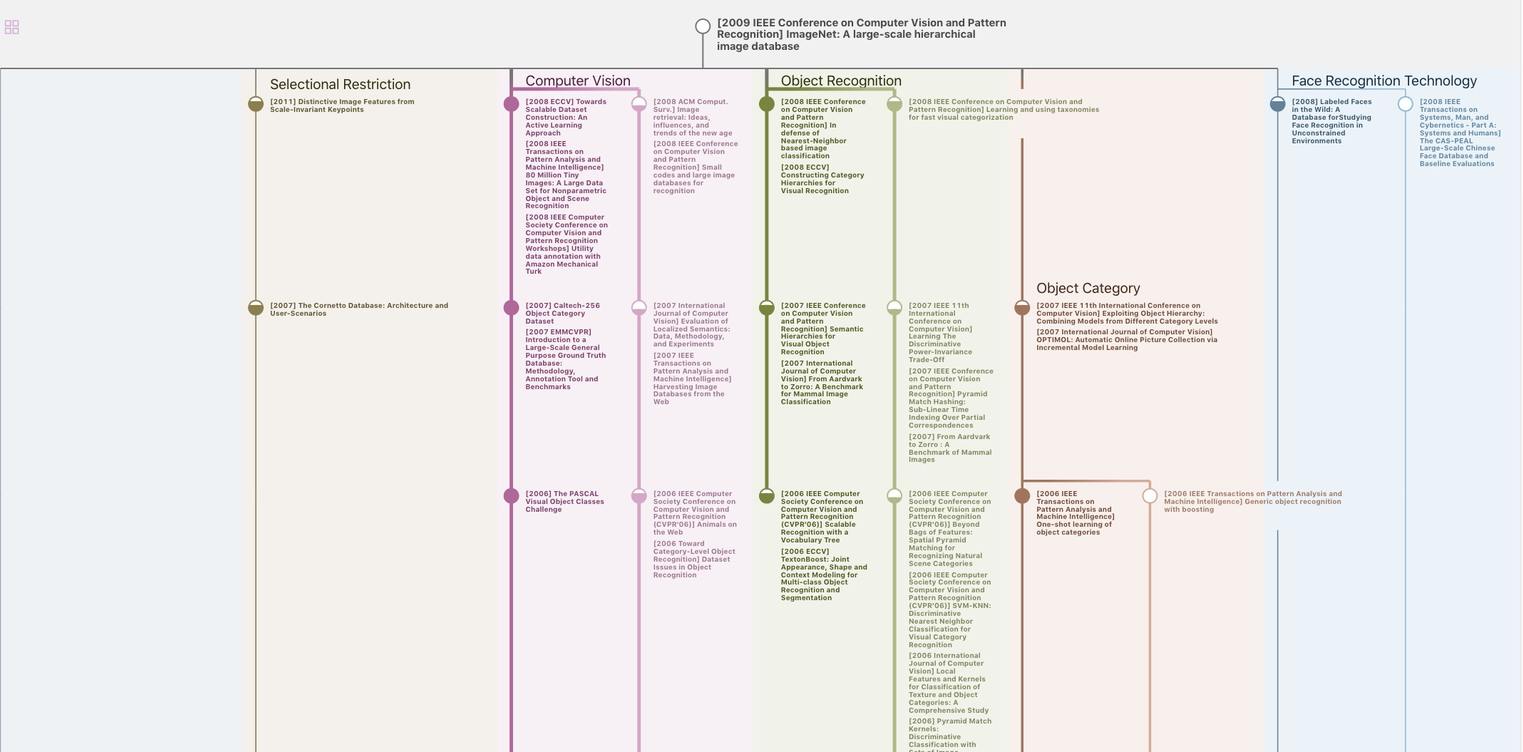
生成溯源树,研究论文发展脉络
Chat Paper
正在生成论文摘要