Enhancing Prognosis Accuracy for Ischemic Cardiovascular Disease Using K Nearest Neighbor Algorithm: A Robust Approach.
IEEE Access(2023)
摘要
Ischemic Cardiovascular diseases are one of the deadliest diseases in the world. However, the mortality rate can be significantly reduced if we can detect the disease precisely and effectively. Machine Learning (ML) models offer substantial assistance to individuals requiring early treatment and disease detection in the realm of cardiovascular health. In response to this critical need, this study developed a robust system to predict ischemic disease accurately using ML-based algorithms. The dataset obtained from Kaggle encompasses a comprehensive collection of over 918 observations, encompassing 12 essential features crucial for predicting ischemic disease. In contrast, much-existing research relies primarily on datasets comprising only 303 instances from the UCI repository. Six ML-based algorithms, including K Nearest Neighbors (KNN), Random Forest (RF), Logistic Regression (LR), Support Vector Machine (SVM), Gaussian Naive Bayes (GNB), and Decision Trees (DT), are trained on the ischemic heart data. The effectiveness of the proposed methodologies is meticulously evaluated and benchmarked against cutting-edge techniques, employing a range of performance criteria. The empirical findings manifest that the KNN classifier produced optimized results with 91.8% accuracy, 91.4% recall, 91.9% F1 score, 92.5% precision, and AUC of 90.27%.
更多查看译文
关键词
Diseases, Classification tree analysis, Predictive models, Support vector machines, Prediction algorithms, Heart, Radio frequency, Cardiovascular diseases, Risk management, Ischemic cardiovascular diseases, healthcare and health risks, SVM, RF, GNB, KNN
AI 理解论文
溯源树
样例
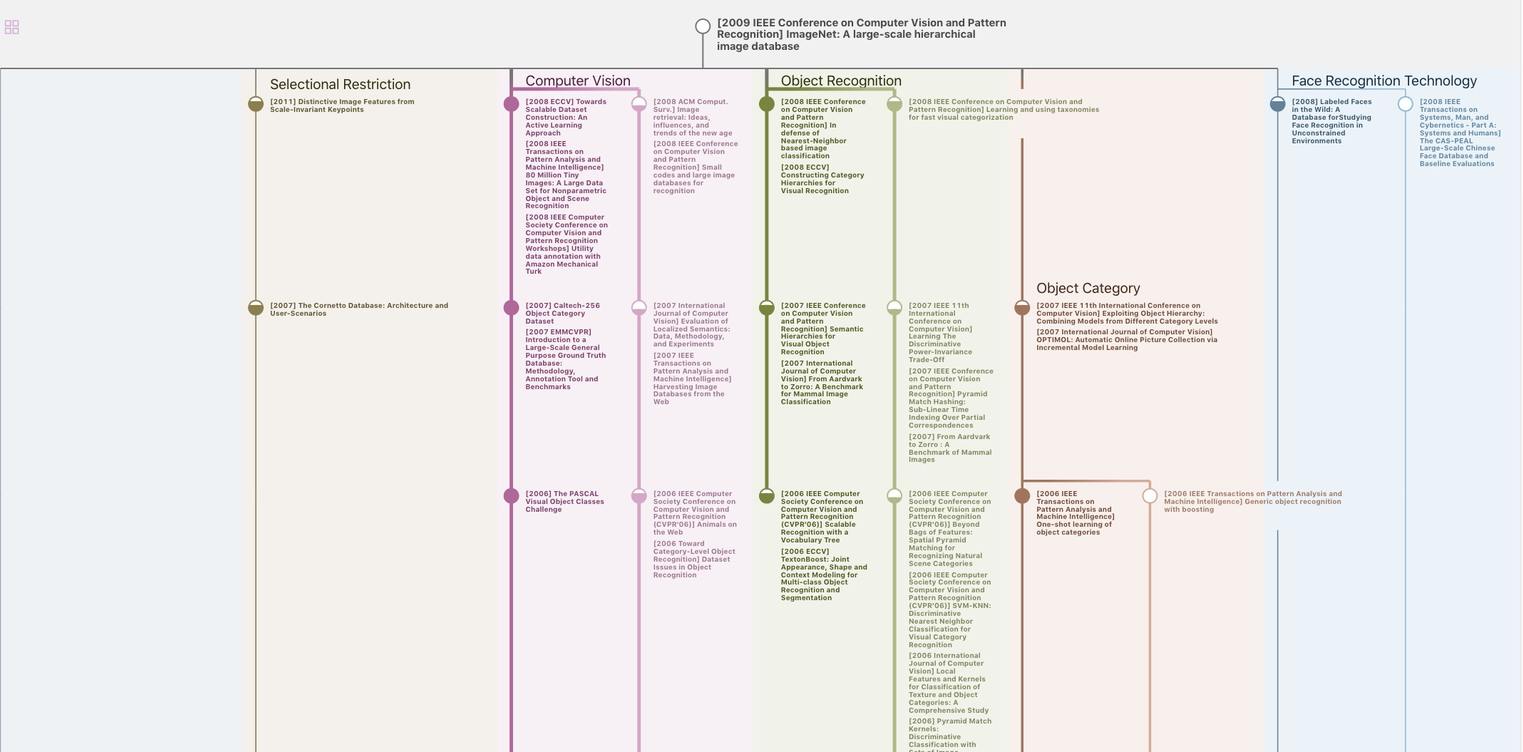
生成溯源树,研究论文发展脉络
Chat Paper
正在生成论文摘要