Closed-Loop Learning for Accuracy Improvement of Nonintrusive Load Monitoring in Smart Homes.
IEEE Trans. Instrum. Meas.(2023)
摘要
Nonintrusive load monitoring (NILM) is a technique that estimates the powers of all loads within a smart home, using only the aggregated power at the main power bus. Up to today, some NILM methods have been well developed. However, their estimation accuracy can be further improved, especially when dealing with the loads that own more than two operating states. In this article, we propose a closed-loop learning method that explores feedback mechanism to reduce the estimation error of NILM. Specifically, the closed-loop learning consists of a feedforward network and a feedback network. The former aims to transform the aggregated power into load powers, as accurately as possible. The latter balances the operating states and exploits the temporal-spatial correlation of different loads, generating a feedback loss that helps improve the former's accuracy. The proposed method is evaluated on the UK-DALE and REDD datasets and is compared with the state-of-the-art methods. Results show that the proposed method indeed improves the accuracy of NILM and can be deployed for practical applications.
更多查看译文
关键词
Accuracy improvement, closed-loop learning, deep neural network, nonintrusive load monitoring (NILM), smart home
AI 理解论文
溯源树
样例
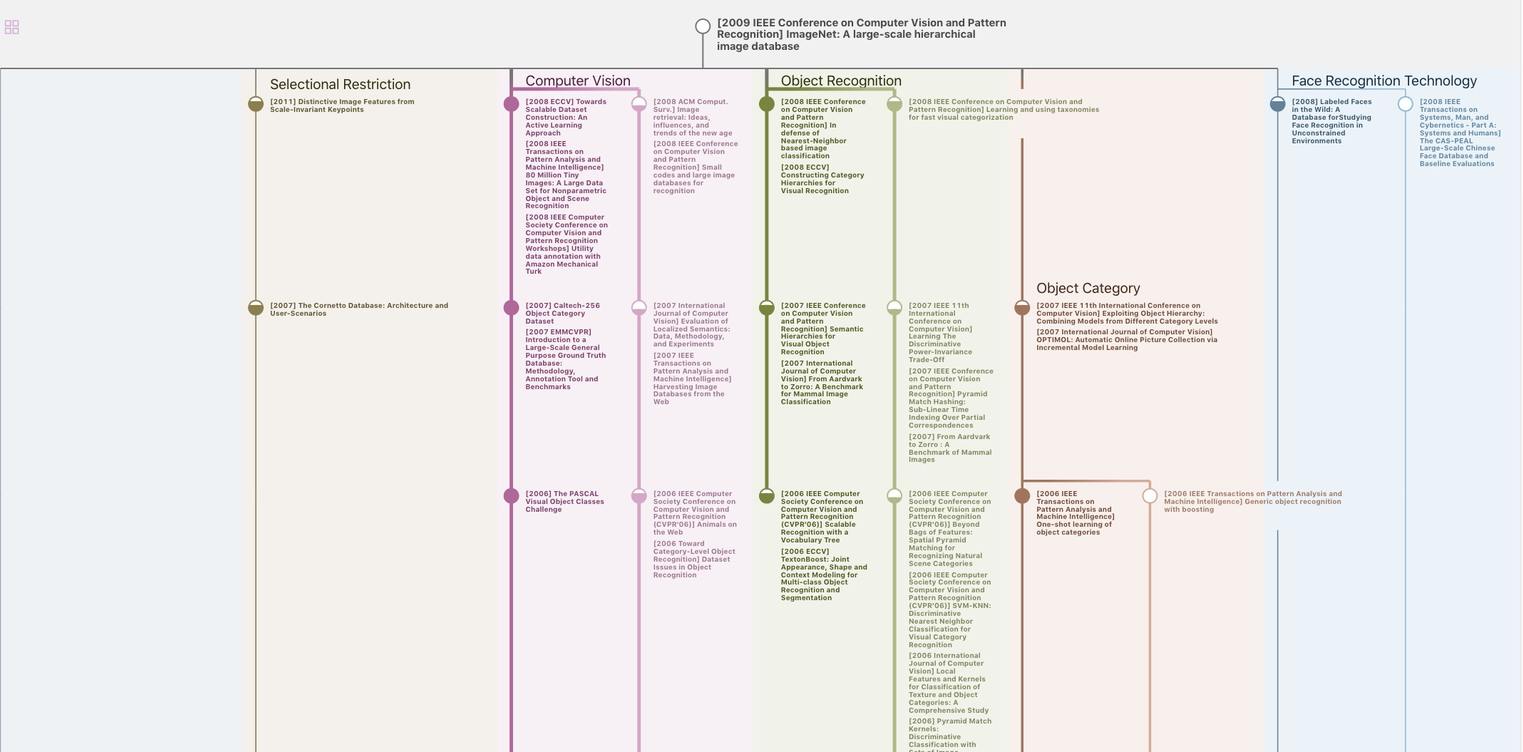
生成溯源树,研究论文发展脉络
Chat Paper
正在生成论文摘要