Policy Fusion Transfer: The Knowledge Transfer for Different Robot Peg-in-Hole Insertion Assemblies.
IEEE Trans. Instrum. Meas.(2023)
摘要
Given the problem of exploration and utilization, the high interaction cost of deep reinforcement learning (DRL) is sometimes unacceptable. Moreover, the model trained by DRL cannot adapt to the complex and changeable assembly environment quickly owing to the weak generalization ability. Thus, the knowledge transfer framework is established for different robot peg-in-hole assemblies in this article to reinforce the generalization ability of the assembly model and the utilization efficiency of data. In the framework, the source domain model is trained through the proximal policy optimization (PPO) algorithm. The model accurately predicts environmental information and dynamically adjusts the robot’s movements based on the prediction. Combined with the PI controller, the flexible peg-in-hole assembly can be quickly achieved by the robot. The policy fusion between the source and target domain MDP is realized by the policy fusion transfer (PFT) algorithm designed in this article. The effectiveness of the algorithm is verified and discussed based on the transfer experiments, involving different assembly objects and different assembly environments. The training results demonstrate that the environment is explored by the PFT algorithm in a safe range at the initial stage of training by reusing the assembly policy of the source domain and that the faster model convergence speed is achieved. Furthermore, the testing results suggest that the assembly success rate is improved by 26% and the assembly force is reduced by 30 N. Moreover, the knowledge transfer between different peg-in-hole assemblies is realized successfully.
更多查看译文
关键词
Assembly policy,industrial robot,peg-in-hole assembly,transfer reinforcement learning
AI 理解论文
溯源树
样例
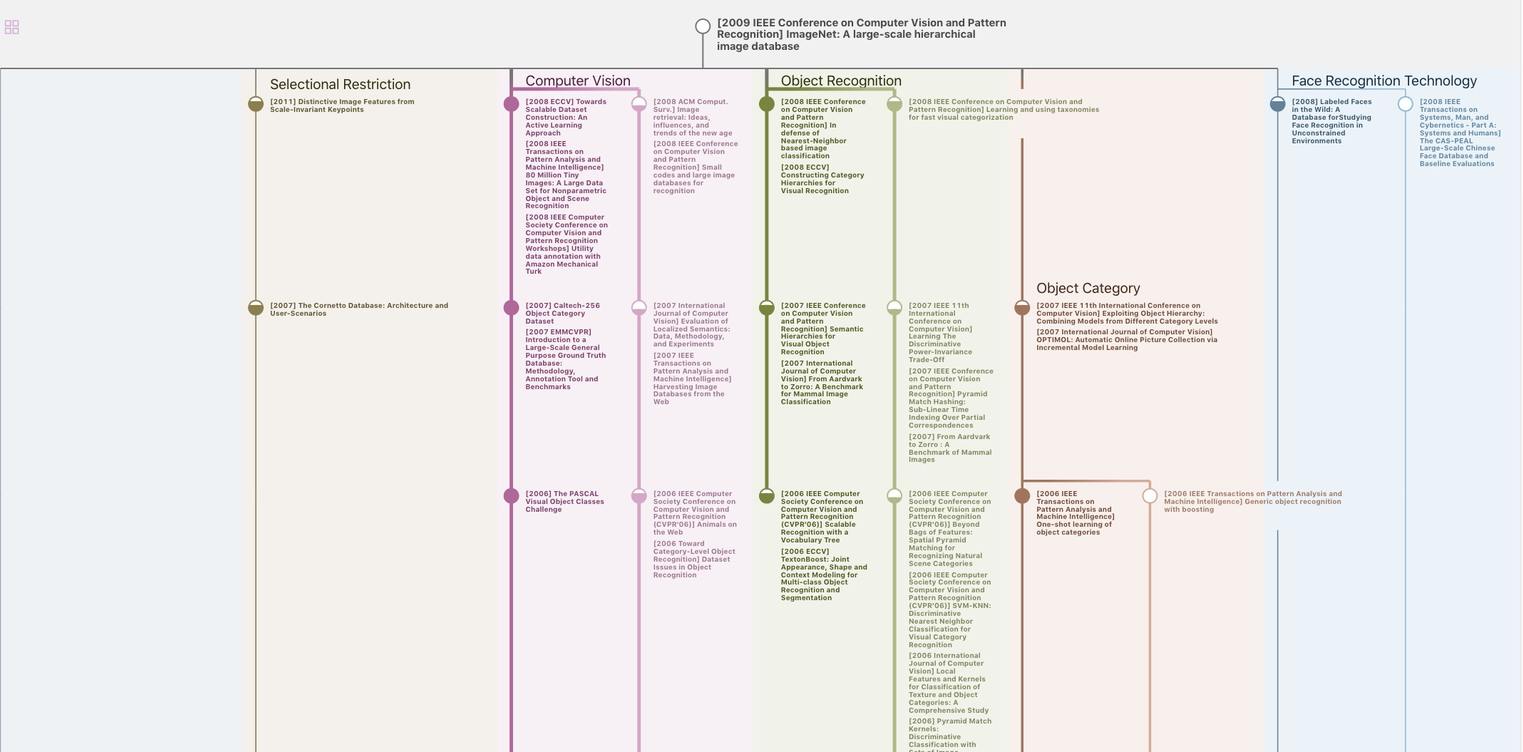
生成溯源树,研究论文发展脉络
Chat Paper
正在生成论文摘要