A Novel Iterative Algorithm for Outlier Robust Phase Retrieval via Majorization Minimization Technique.
IEEE Trans. Instrum. Meas.(2023)
摘要
In this article, we present a novel iterative primal-dual algorithm based on the majorization minimization (MM) principle to solve the problem of robust phase retrieval. The algorithm minimizes the reverse Kullback-Leibler divergence (RKLD) loss function, which is known to be not dependent on the statistical distribution of the noise in the measurements and also robust to presence of additive outliers. As the RKLD objective function is nonconvex, we reformulate the RKLD minimization problem to a minimax optimization problem by using the Fenchel dual representation of some term in the objective function. We then solve this minimax problem using the MM technique over the primal and dual variables, resulting in an iterative algorithm, referred to as RKLD-MM. The primal problem admits a closed-form solution, and we obtain the solution to the dual problem by solving the associated Karush-Kuhn-Tucker (KKT) condition, which requires the use of the Lambert function. We also extend the proposed algorithm to solve the problem with the l(1) -regularization that promotes sparsity, which we refer to as RKLD-MMR. The proposed algorithm does not require tuning of any hyperparameter and it is guaranteed to converge to a stationary point of the problem. We present various numerical simulations under different noise distributions on synthetic and experimental datasets. The RKLD-MM algorithm consistently performs better than the other state-of-the-art algorithms in terms of normalized root-mean-square error (NRMSE) in recovering the unknown signal and also effectively handles the outliers in the measurements.
更多查看译文
关键词
Majorization minimization (MM),phase retrieval,reverse Kullback–Leibler divergence (RKLD),robust estimation
AI 理解论文
溯源树
样例
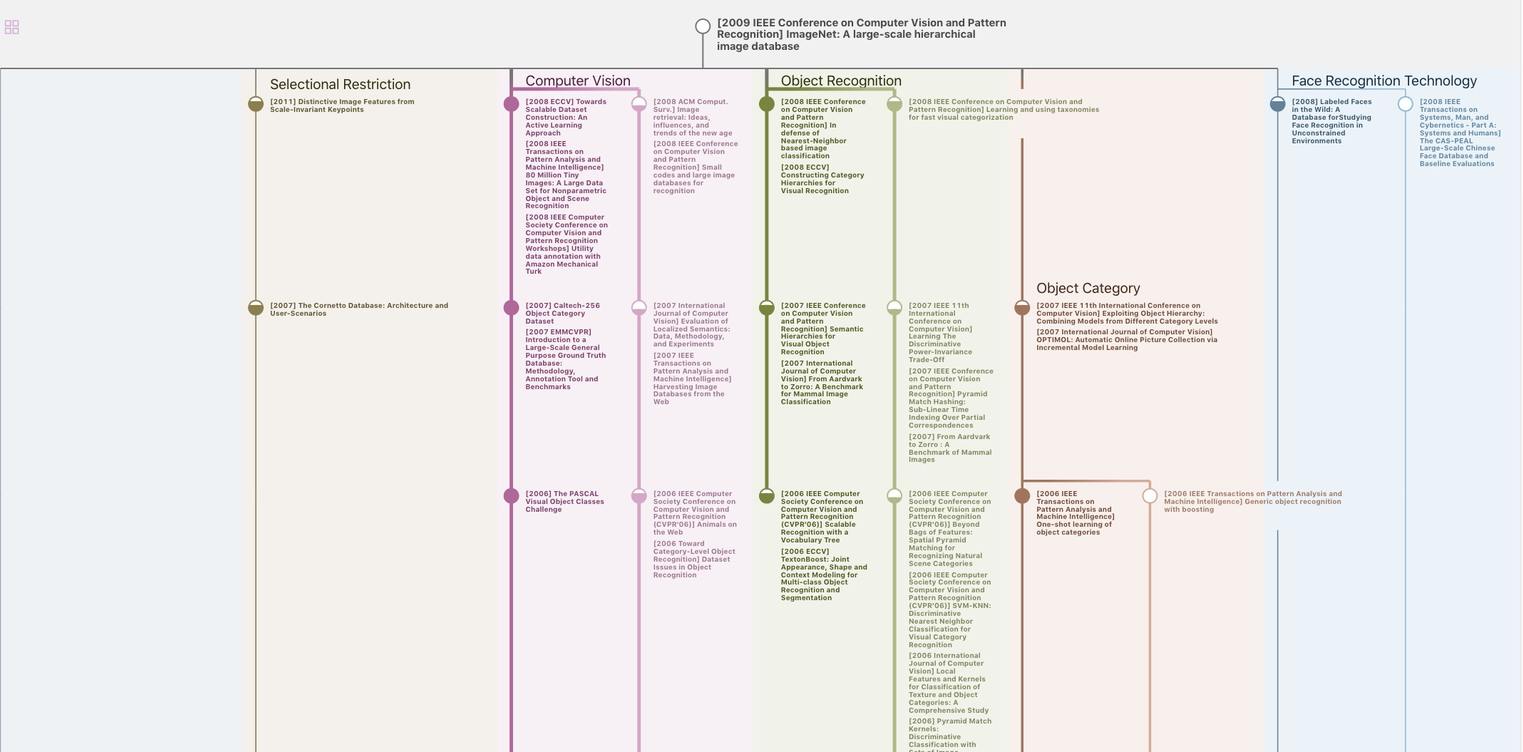
生成溯源树,研究论文发展脉络
Chat Paper
正在生成论文摘要