Coupling Effect of Exploration Rate and Learning Rate for Optimized Scaled Reinforcement Learning
SN Comput. Sci.(2023)
摘要
Reinforcement learning (RL) is making paradigm transformations in artificial intelligence frameworks in the field of autonomous robotics. This learning methodology primarily depends on three fundamental parameters namely—(i) learning rate, (ii) exploration rate, and (iii) discount factor. Out of these parameters, the learning rate and exploration rate play a pivotal role in optimizing the training process for the RL agent which is yet to be explored under an integrated environment. This work proposes a methodology to extract a coupled framework incorporating both the exploration and learning rates for the optimization of scaled reinforcement learning. Additionally, it also explores learning curves w.r.t the episode length and with reference to the cumulative reward for different maze architecture. Further, it also attributes the determination of both execution time and the number of iterations for an RL agent functioning under optimal test conditions ( ϵ = 0 . 9 , α = 0 . 9 , γ = 0 . 9). This led to an investigation that comprised extracting the inflection point for a detailed understanding of this coupled framework using differentials.
更多查看译文
关键词
exploration rate,learning rate
AI 理解论文
溯源树
样例
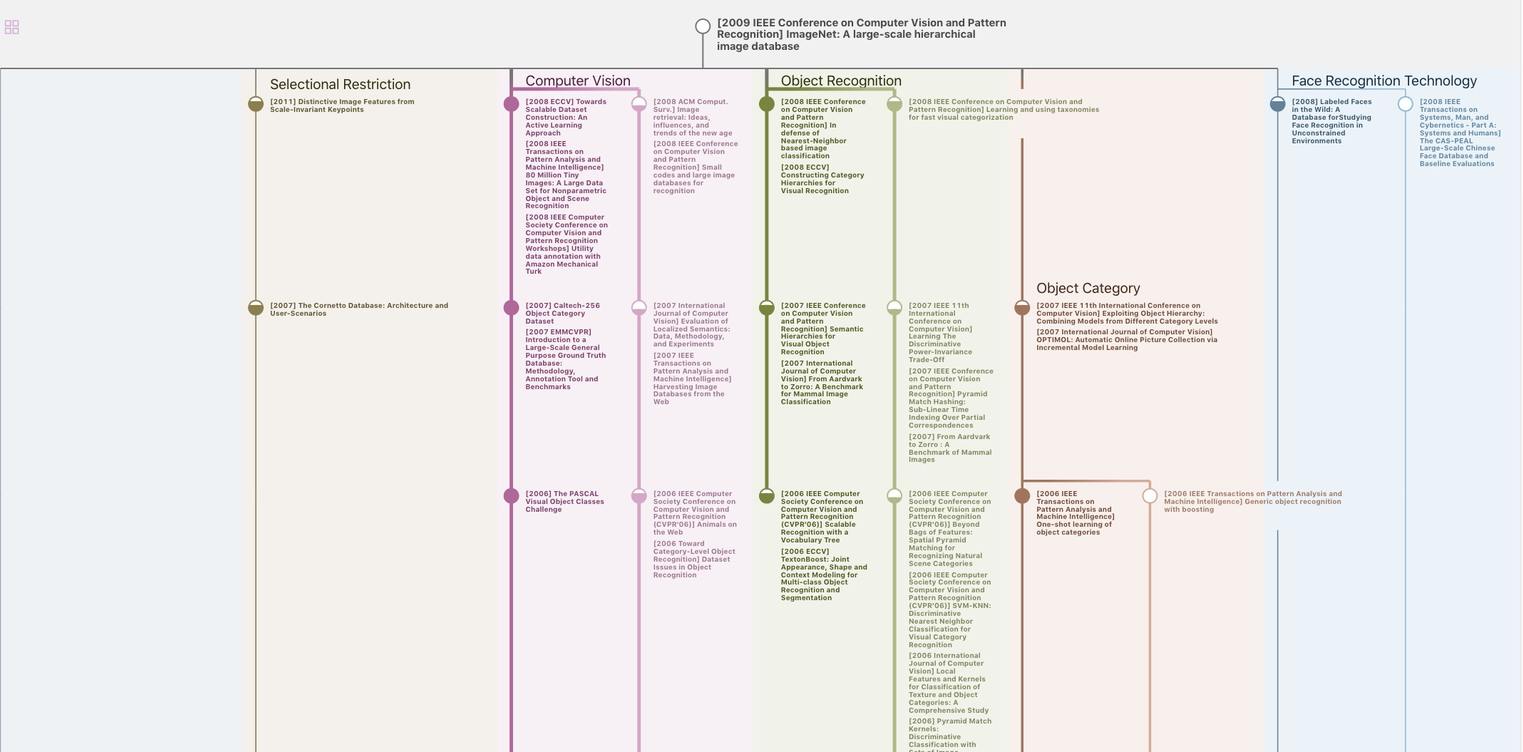
生成溯源树,研究论文发展脉络
Chat Paper
正在生成论文摘要