Data Driven Energy Economy Prediction for Electric City Buses Using Machine Learning
IEEE Access(2023)
摘要
Electrification of transportation systems is increasing, in particular city buses raise enormous potential. Deep understanding of real-world driving data is essential for vehicle design and fleet operation. Various technological aspects must be considered to run alternative powertrains efficiently. Uncertainty about energy demand results in conservative design which implies inefficiency and high costs. Both, industry, and academia miss analytical solutions to solve this problem due to complexity and interrelation of parameters. Precise energy demand prediction enables significant cost reduction by optimized operations. This paper aims at increased transparency of battery electric buses' (BEB) energy economy. We introduce novel sets of explanatory variables to characterize speed profiles, which we utilize in powerful machine learning methods. We develop and comprehensively assess 5 different algorithms regarding prediction accuracy, robustness, and overall applicability. Achieving a prediction accuracy of more than 94%, our models performed excellent in combination with the sophisticated selection of features. The presented methodology bears enormous potential for manufacturers, fleet operators and communities to transform mobility and thus pave the way for sustainable, public transportation.
更多查看译文
关键词
Battery electric buses,energy demand prediction,feature extraction,machine learning,meta modeling
AI 理解论文
溯源树
样例
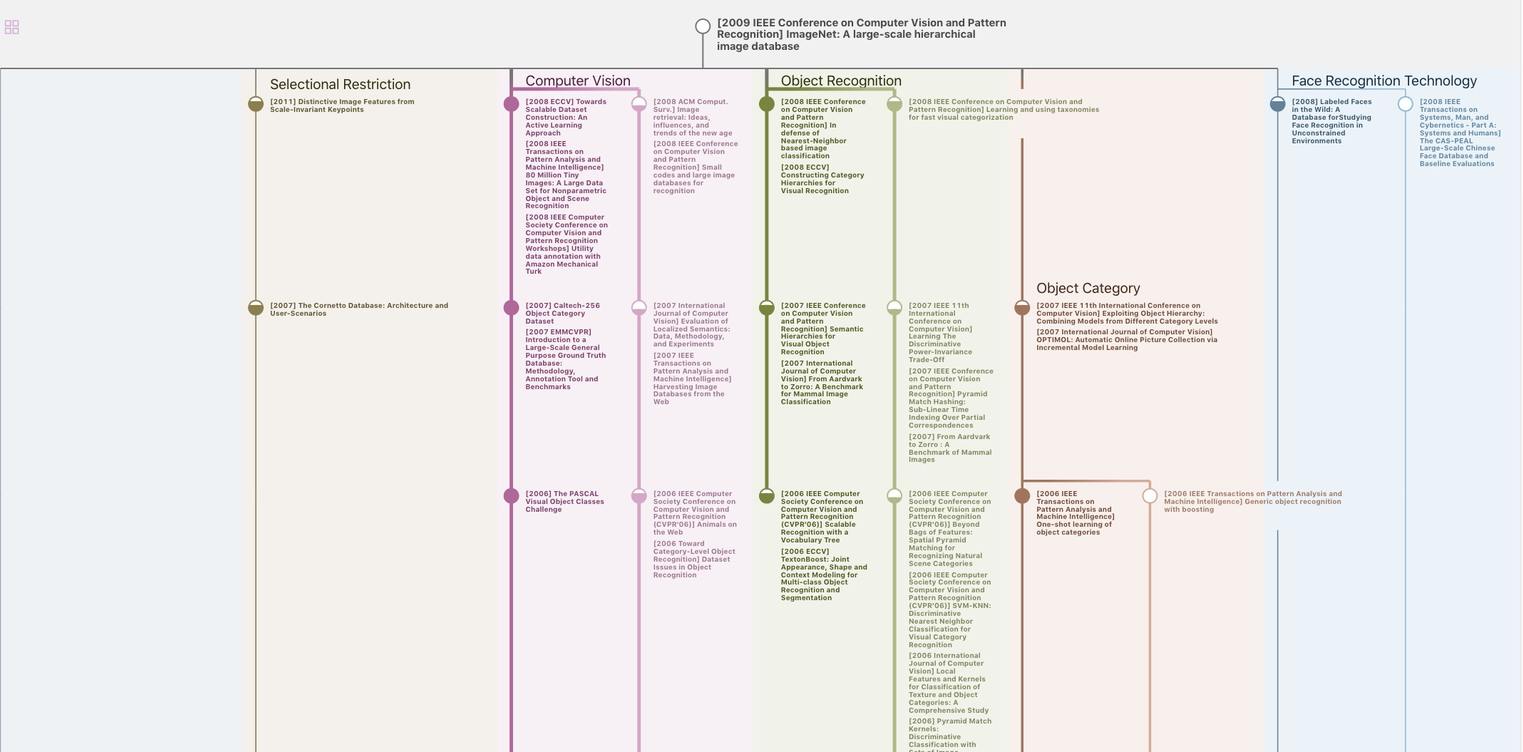
生成溯源树,研究论文发展脉络
Chat Paper
正在生成论文摘要