Scale-Aware Frequency Attention network for super-resolution
Neurocomputing(2023)
摘要
Scale-arbitrary super-resolution (SASR) has recently gained widespread attention in the community, which aims to super-resolve an image to arbitrary desired resolutions. Existing SASR methods always use a fixed convolution neural network (CNN) as the backbone to share the same image features for different resolutions, leading to limited reconstruction performance due to the lack of distinctive feature extraction for individual resolutions. Meanwhile, these CNN-based methods always tend to overfit low-frequency components while losing high-frequency ones. This frequency gap leads to blurred structures in the reconstructed image. To address these issues, we propose a Scale Aware Frequency Attention network (SAFANet), which consists of Scale-Aware Frequency Attention (SAFA) modules and a Scale-Guided Continuous Reconstruction (SGCR) module. Additionally, frequency-domain attention learning is introduced to optimize the scale-adapted features to explicitly focus on high-frequency components, thereby overcoming the frequency restoration gap. Finally, the SGCR module combines the input scale as guidance with the optimized features to reconstruct images with arbitrary resolutions by using an implicit reconstruction function, thus adaptively achieving continuous image restoration. Our proposed SAFANet can be easily incorporated into existing CNN-based SR networks to achieve interactive SR at arbitrary scales. Extensive experiments demonstrate that SAFANet achieves superior performance, which is competitive with or even better than the state-of-the-art methods across a vast scale range (×1∼×30) via a single model.
更多查看译文
关键词
Scale-arbitrary image super-resolution,Frequency domain learning
AI 理解论文
溯源树
样例
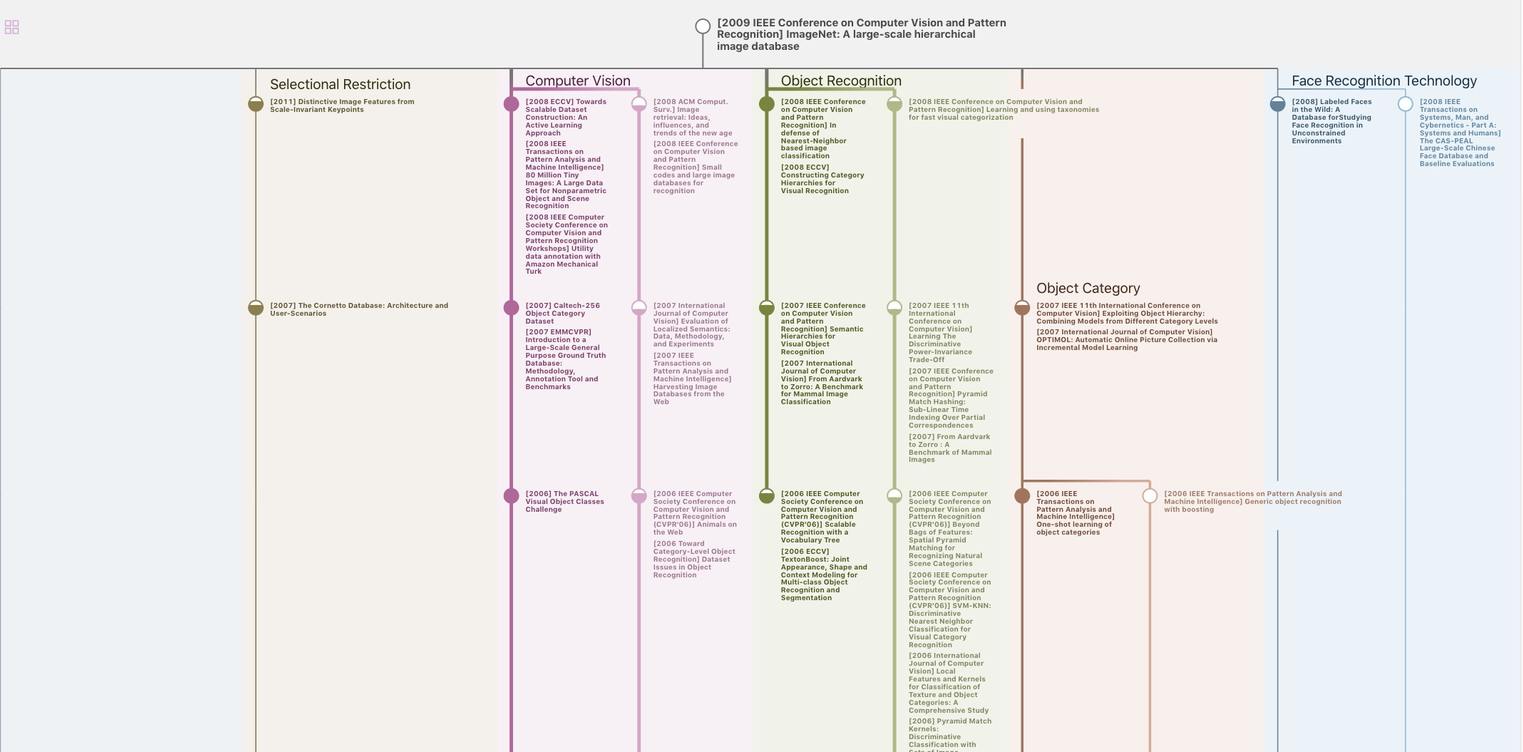
生成溯源树,研究论文发展脉络
Chat Paper
正在生成论文摘要