Multi-proximity based embedding scheme for learning vector quantization-based classification of biochemical structured data.
Neurocomputing(2023)
摘要
In this paper, we propose a data embedding technique for structured data that allows for the direct application of standard vector-based machine learning models without the need for explicit feature extraction. Our approach relies on multiple notions of data proximity, making it suitable for handling mixed data types or incorporating domain knowledge. Our method also reduces the computational costs of pairwise proximity calculations, resulting in improved efficiency and scalability. We demonstrate the effectiveness of our technique on graph and sequence datasets from the biochemical domain. In particular, we show that the method can efficiently be combined with interpretable machine learning approaches like relevance-based learning vector quantization for sophisticated classification learning.
更多查看译文
关键词
Structured data,Mixed data,Data transformation,Sensoric response principle,Dissimilarity representation,Classification,Relevance learning
AI 理解论文
溯源树
样例
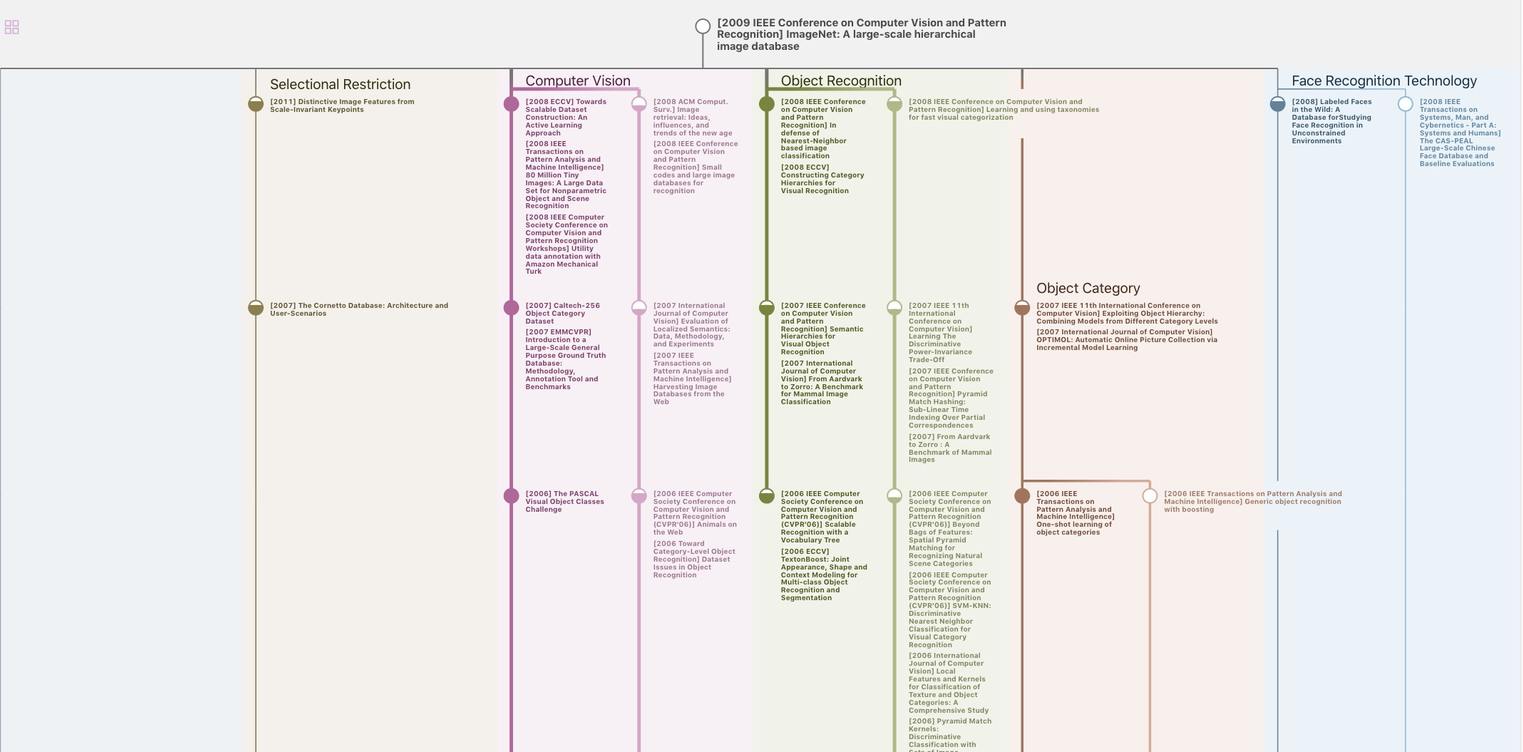
生成溯源树,研究论文发展脉络
Chat Paper
正在生成论文摘要