Class Incremental Learning based on Identically Distributed Parallel One-Class Classifiers.
Neurocomputing(2023)
摘要
Class incremental learning requires models to learn new-class knowledge without forgetting old-class in-formation. As a natural solution, the parallel one-class framework (POC) has attracted extensive attention. However, POC is prone to suffer the problem of lacking comparability between classifiers due to their inconsistent output distributions. To address this drawback, we propose an incremental learning method based on Identically Distributed Parallel One-class Classifiers (IDPOC). The core of IDPOC is a novel one-class classifier with Gaussian distributed output, referred to as Deep-SVD2D. Deep-SVD2D encourages the distribution of sample representations to follow the standard multivariate Gaussian. Consequently, the distance between the representation and its class center will approximately follow a chi-square distribution with some freedom degree. IDPOC further eliminates the freedom degree to ensure the output of all classifiers to follow an identical distribution, thus enhancing the comparability between different classifiers. We evaluate IDPOC on four popular benchmarks: MNIST, CIFAR10, CIFAR100, and Tiny-ImageNet. The experimental results show that IDPOC achieves state-of-the-art performance, e.g., it outperforms the best baseline by 1.6% and 2.8% on two large-scale benchmarks of CIFAR100 and Tiny-ImageNet, respectively 1.
更多查看译文
关键词
Incremental learning,Continual learning,Lifelong learning,One-class learning,Image classification
AI 理解论文
溯源树
样例
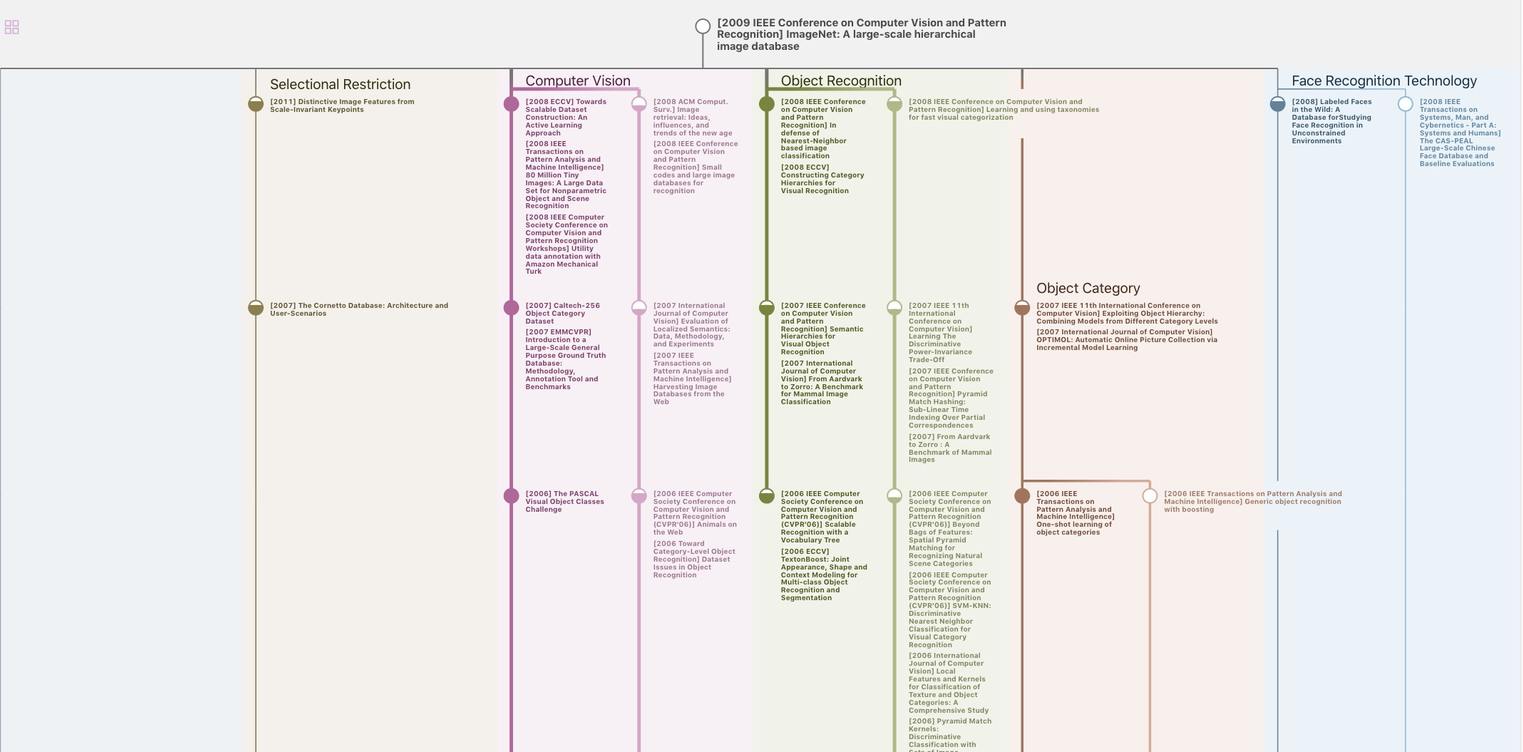
生成溯源树,研究论文发展脉络
Chat Paper
正在生成论文摘要