Choquet regularization for continuous-time reinforcement learning
SIAM JOURNAL ON CONTROL AND OPTIMIZATION(2023)
摘要
We propose Choquet regularizers to measure and manage the level of exploration for reinforcement learning (RL) and reformulate the continuous-time entropy-regularized RL problem of H. Wang, T. Zariphopoulou, and X. Zhou [J. Mach. Learn. Res., 21 (2020), pp. 1--34] in which we replace the differential entropy used for regularization with a Choquet regularizer. We derive the Hamilton-Jacobi-Bellman equation of the problem and solve it explicitly in the linear-quadratic (LQ) case via maximizing statically a mean-variance constrained Choquet regularizer. Under the LQ setting, we derive explicit optimal distributions for several specific Choquet regularizers and conversely identify the Choquet regularizers that generate a number of broadly used exploratory samplers, such as \varepsilon -greedy, exponential, uniform, and Gaussian.
更多查看译文
关键词
reinforcement learning,Choquet integrals,continuous time,exploration,regularizers,quantile,HJB equations,linear-quadratic control
AI 理解论文
溯源树
样例
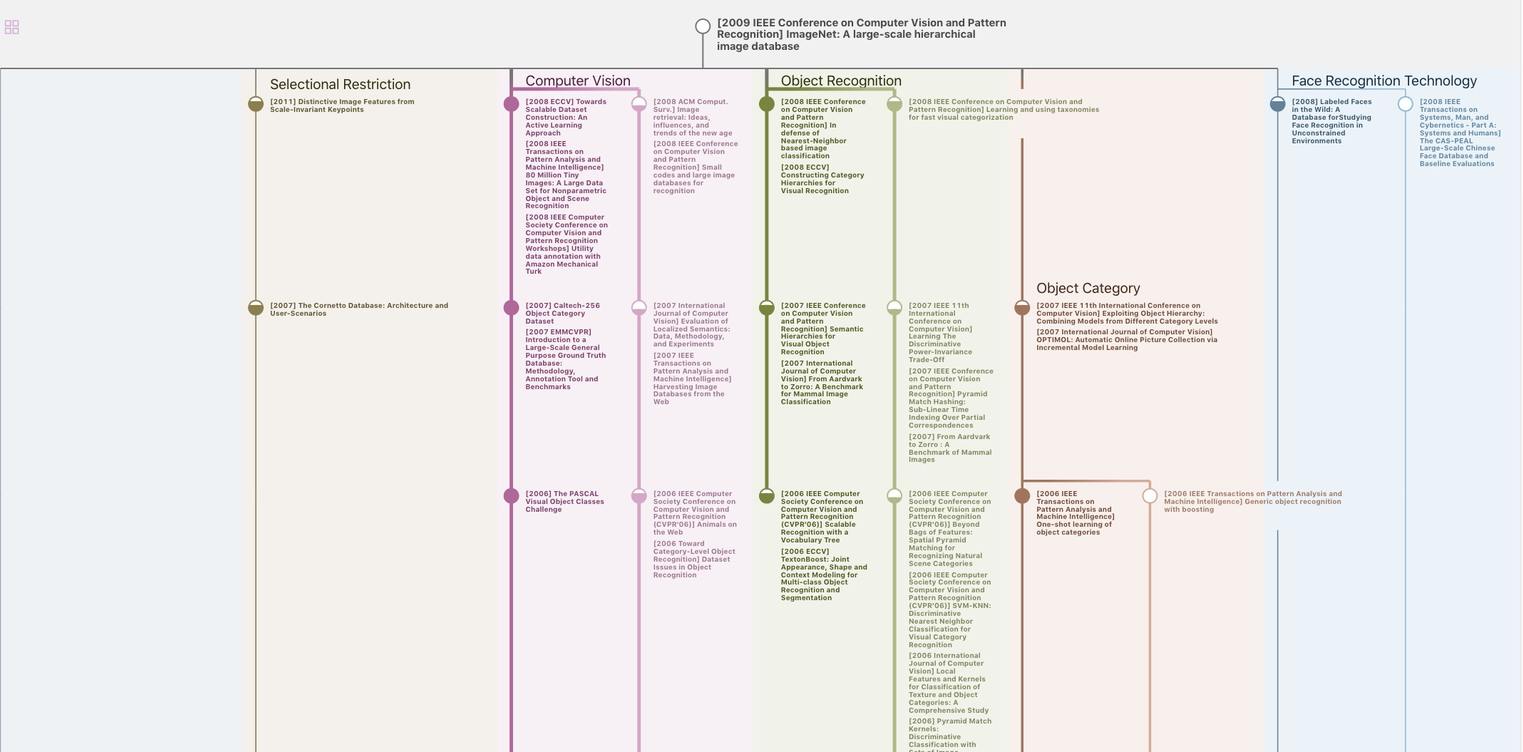
生成溯源树,研究论文发展脉络
Chat Paper
正在生成论文摘要