A hierarchical constraint-based graph neural network for imputing urban area data.
Int. J. Geogr. Inf. Sci.(2023)
摘要
Urban area data are strategically important for public safety, urban management, and planning. Previous research has attempted to estimate the values of unsampled regular areas, while minimal attention has been paid to the values of irregular areas. To address this problem, this study proposes a hierarchical geospatial graph neural network model based on the spatial hierarchical constraints of areas. The model first characterizes spatial relationships between irregular areas at different spatial scales. Then, it aggregates information from neighboring areas with graph neural networks, and finally, it imputes missing values in fine-grained areas under hierarchical relationship constraints. To investigate the performance of the proposed model, we constructed a new dataset consisting of the urban statistical values of irregular areas in New York City. Experiments on the dataset show that the proposed model outperforms state-of-the-art baselines and exhibits robustness. The model is adaptable to numerous geographic applications, including traffic management, public safety, and public resource allocation.
更多查看译文
关键词
graph,neural network,urban,data,constraint-based
AI 理解论文
溯源树
样例
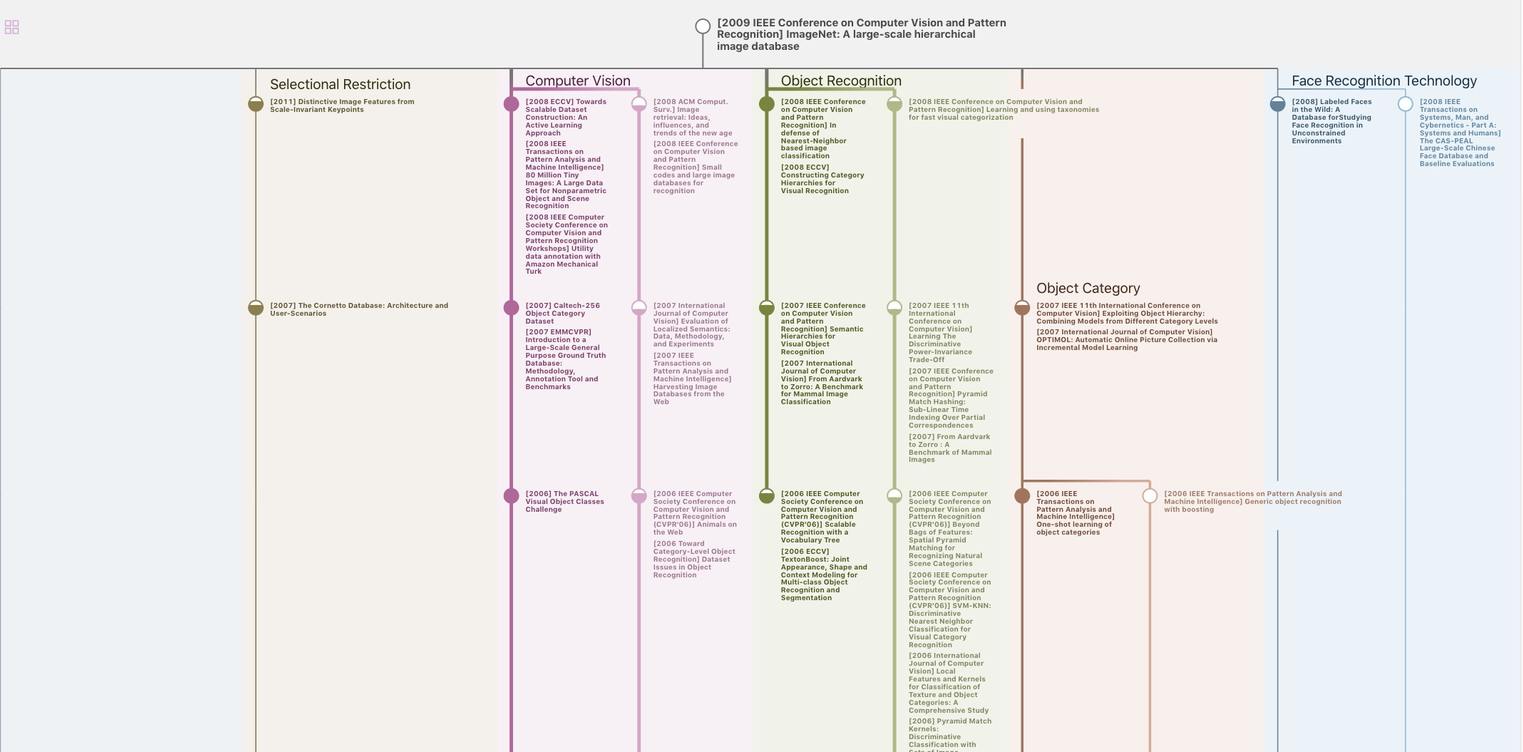
生成溯源树,研究论文发展脉络
Chat Paper
正在生成论文摘要