Unleashing the Power of Graph Spectral Sparsification for Power Grid Analysis via Incomplete Cholesky Factorization.
IEEE Trans. Comput. Aided Des. Integr. Circuits Syst.(2023)
摘要
Graph spectral sparsification-based preconditioning technique has shown promising results for power grid analysis. However, the conventional methods converge slowly for high-accuracy requirement. In this work, we propose an efficient approach to address this issue. Instead of using the Cholesky factorization, we employ the incomplete Cholesky factorization to factorize the spectral sparsifier. We also propose a concept of graph spectral pattern, which can further reduce the preconditioned conjugate gradient (PCG) iterations using less number of nonzeros. Experiments show that under 10(-6) relative tolerance, our proposed preconditioning technique achieves 1.17x speedup compared to AMGPCG in average; compared to the conventional spectral sparsification-based preconditioning techniques, our proposed approach achieves up to 8.53x speedup of the factorization, 8.74x speedup of the PCG iteration, and 5.6x speedup of the total time. Moreover, the speedup of the total time continues to enlarge for higher-accuracy requirement, e.g., 10(-12). Finally, but not least, our method is compatible with existing graph spectral sparsification algorithms for power grid analysis.
更多查看译文
关键词
Power grids, Laplace equations, Convergence, Iterative methods, Symmetric matrices, Fans, Runtime, Incomplete Cholesky (IC) factorization, power grid analysis, preconditioned conjugate gradient (PCG) iterative solver, spectral graph sparsification
AI 理解论文
溯源树
样例
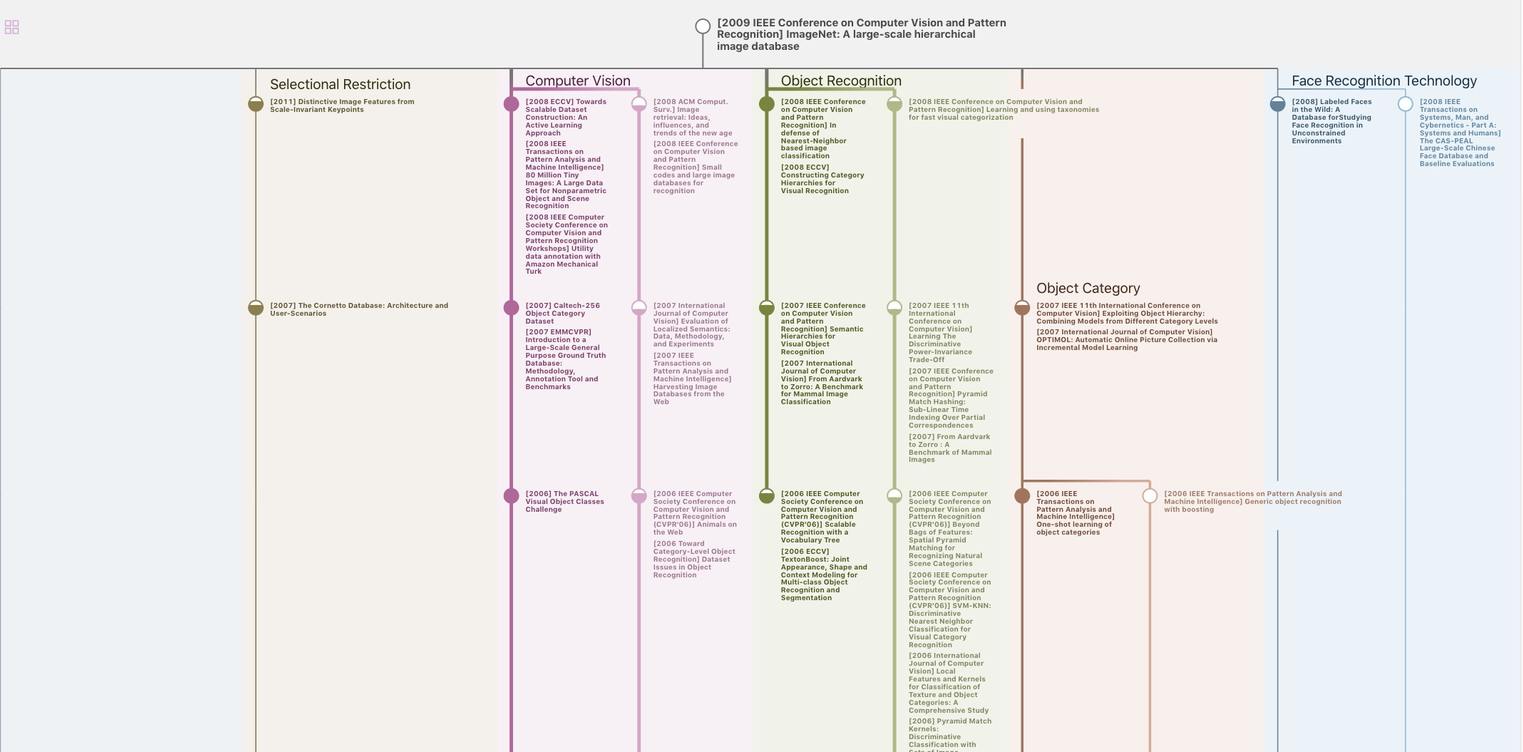
生成溯源树,研究论文发展脉络
Chat Paper
正在生成论文摘要