Semantic Segmentation of Point Cloud With Novel Neural Radiation Field Convolution.
IEEE Geosci. Remote. Sens. Lett.(2023)
摘要
Point cloud semantic segmentation predicts the semantic class of each point, which can help AI machines perceive the real 3-D world. Recently, precision for large-scale point cloud is limited by complex scenarios, data occlusion, and massive data, which remains. This letter proposes neural radiance field convolution (NeRFConv) for large-scale point cloud semantic analysis. First, to conquer some existing methods represent the point cloud local space as the relative position without considering the rotation property of the point cloud. A new spatial direction representation called neural radiance field 7-D is constructed to denote the local point cloud space, which is unaffected by the rotation of the coordinate axes. Then, to be robust against the varying density of the point cloud, an adaptive convolution method is proposed to aggregate the local point cloud features and obtain more expressive local features. Finally, the proposed method achieves state-of-the-art performance on the test dataset. In particular, mIoU of 58.1% and 58.3% were achieved the SensatUrban and SementicKITTI datasets, respectively.
更多查看译文
关键词
point cloud,field,radiation
AI 理解论文
溯源树
样例
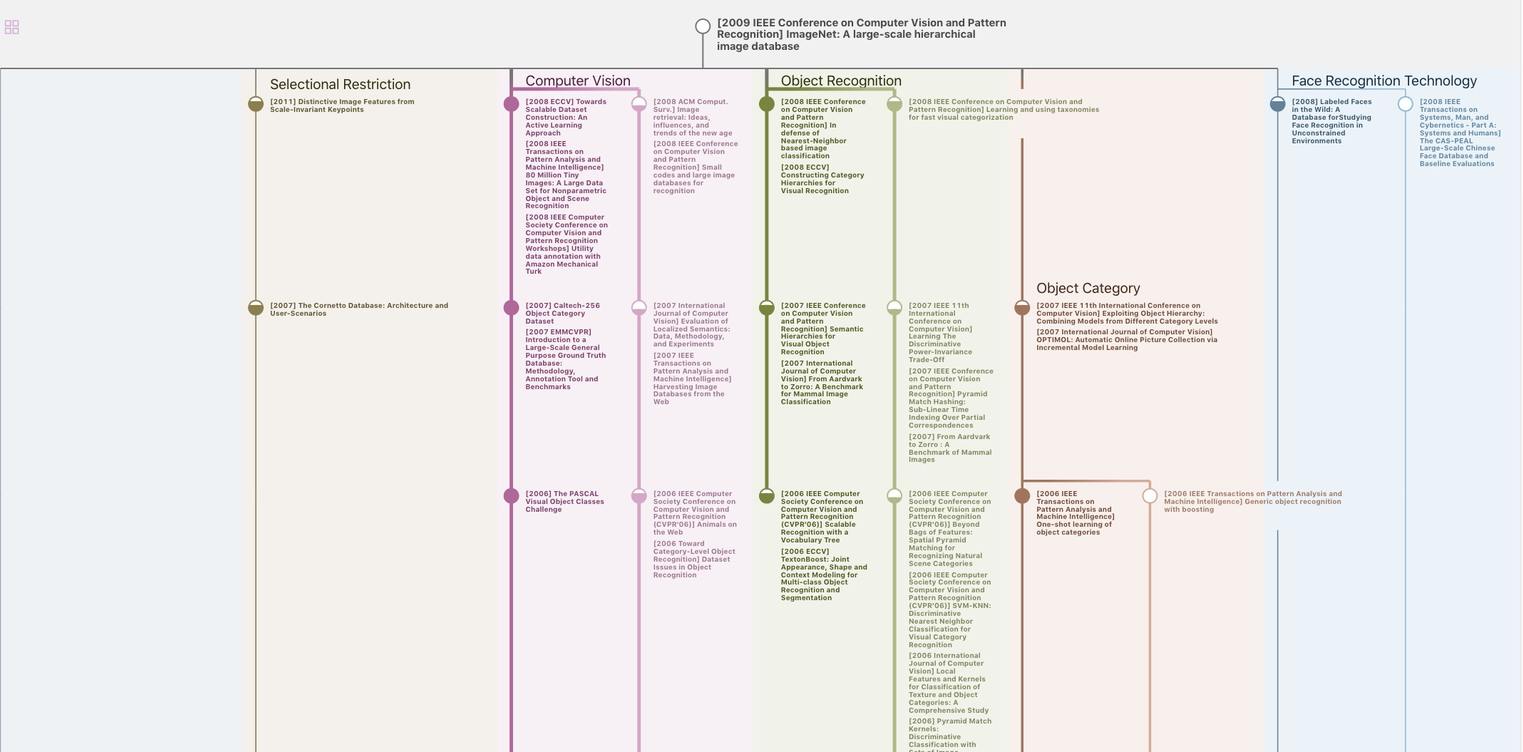
生成溯源树,研究论文发展脉络
Chat Paper
正在生成论文摘要