Window Transformer Convolutional Autoencoder for Hyperspectral Sparse Unmixing.
IEEE Geosci. Remote. Sens. Lett.(2023)
摘要
The availability of spectral library makes hyperspectral sparse unmixing an attractive unmixing scheme, and the powerful feature extraction capability of deep learning meets the requirements of estimating abundances with hundreds of channels in sparse unmixing. However, few related researches have been carried out. In this letter, we propose a window transformer convolutional autoencoder (WiTCAE) to address the sparse unmixing problem. In our method, a well-designed transformer encoder for hyperspectral images (HIS) is applied before convolutional neural network (CNN), aiming at exploring nonlocal information by a new attention mechanism called window-based pixel-level multihead self-attention (WP-MSA). Three consecutive CNN blocks focus on further joint spatial-spectral feature extraction and adjust the number of channels to the number of endmembers contained in the spectral library. Moreover, CNN establishes the connections among windows and smooths out the discontinuities caused by window partition. The decoder is a convolutional layer with a kernel size of 1, and its weights are fixed to a known spectral library. Comparative experiments on both simulated and real datasets confirm the superiority of our proposed network.
更多查看译文
关键词
hyperspectral sparse unmixing,transformer
AI 理解论文
溯源树
样例
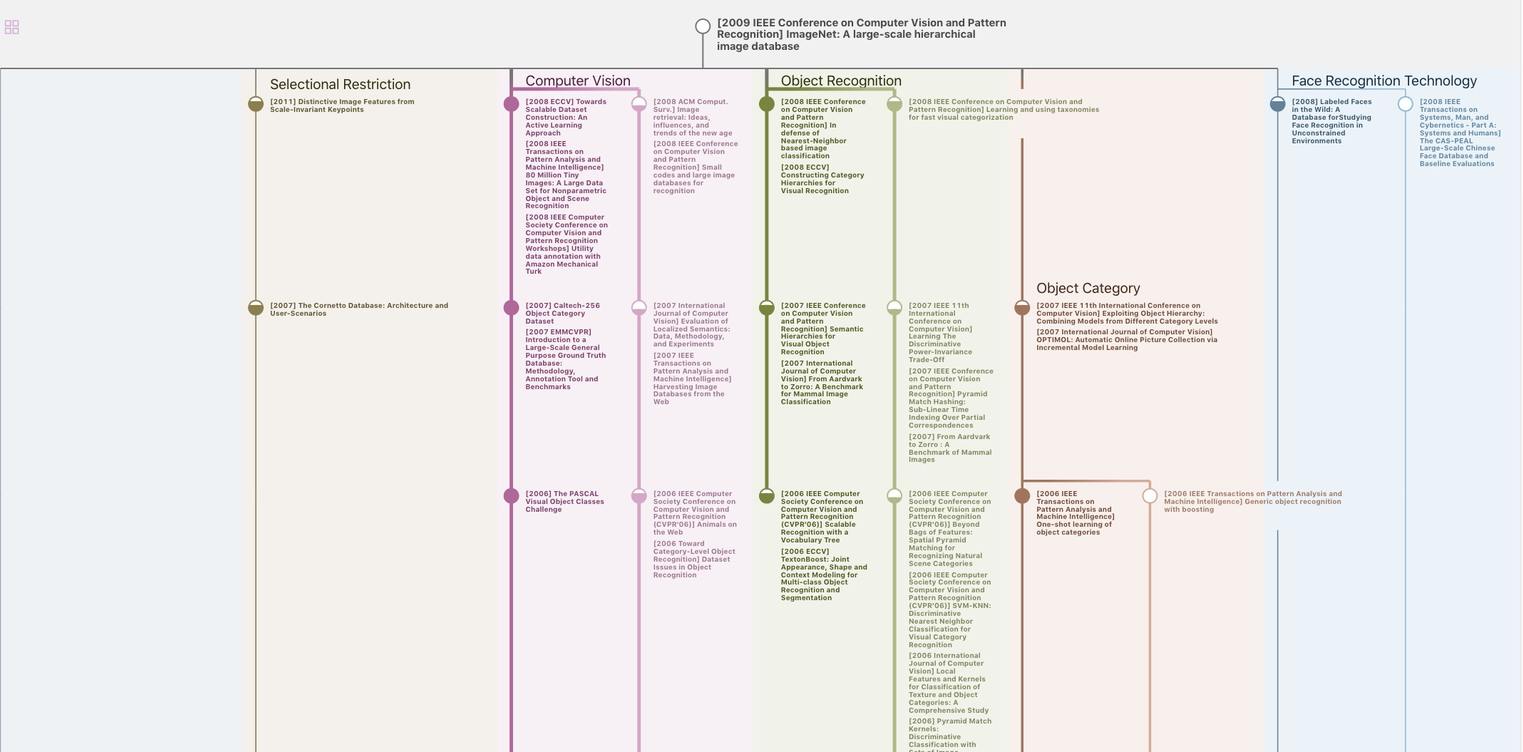
生成溯源树,研究论文发展脉络
Chat Paper
正在生成论文摘要