Cluster-based data relabelling for classification
INFORMATION SCIENCES(2023)
摘要
Linear classifiers are generally simpler and more explainable than their nonlinear variants. They can achieve satisfactory classification performance on linearly separable data, but not on nonlinear data. So, linear classifiers need extending, typically by modification of their algorithms, resulting in their nonlinear variants. In this paper we present one general method, cluster-based data relabelling (CBDR), that allows linear classifiers to work effectively on nonlinear data. CBDR partitions the data set into several non-overlapping class-specific clusters and relabels data by the clusters. A linear classifier can then be applied to the relabelled data to seek cluster-based linear decision boundaries instead of class-based decision boundaries. Extensive experimentation has demonstrated that CBDR can significantly enhance the classification performance of linear classifiers, and even outperform their nonlinear variants. Further experimentation has demonstrated that CBDR can also improve the classification performance of nonlinear classifiers. Most significant outperformance was observed on imbalanced data in both cases.
更多查看译文
关键词
Classification,Classifier,Cluster-based data relabelling,Linear discriminant analysis classifier,Support vector machine,Multilayer perceptron,Naive Bayes classifier,Decision tree,Machine learning,Pattern recognition
AI 理解论文
溯源树
样例
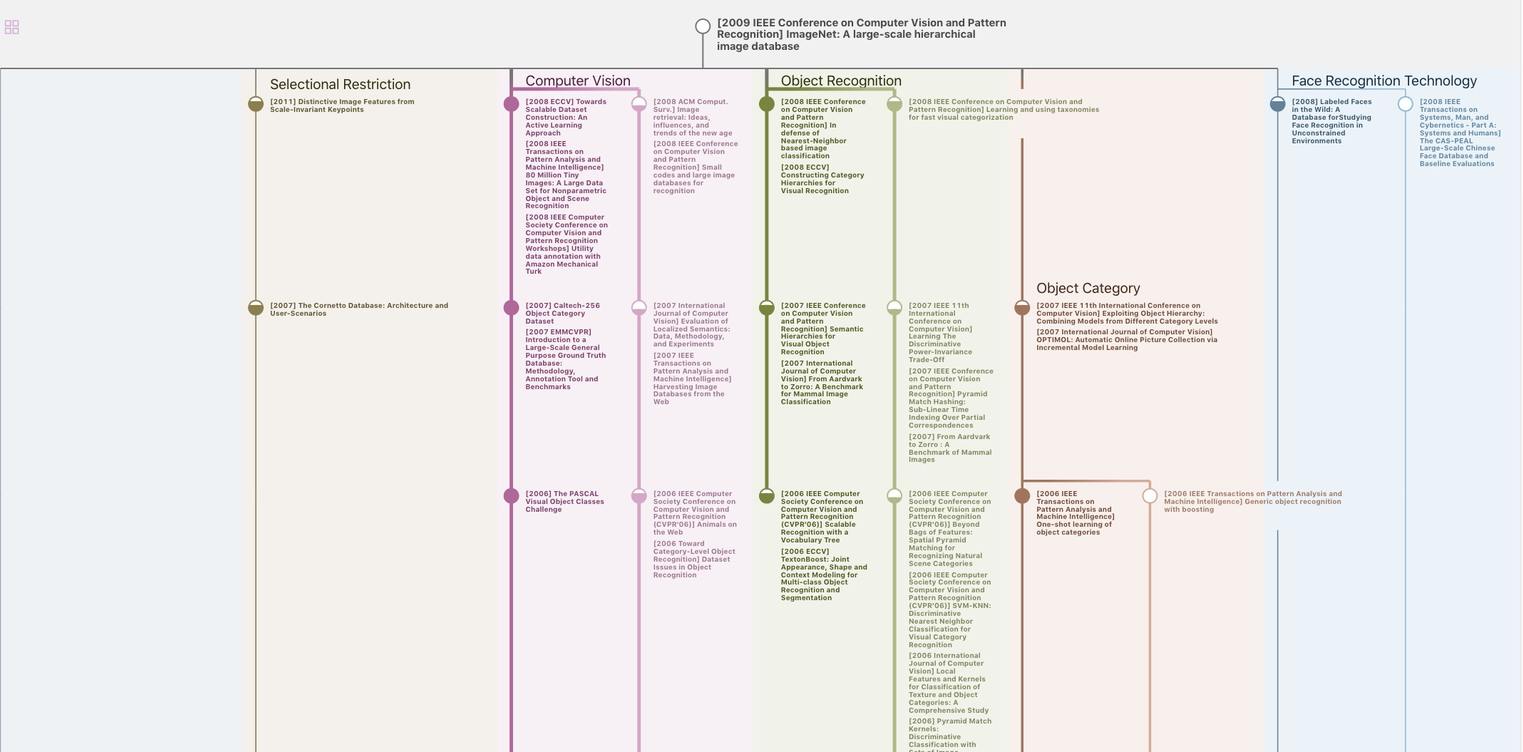
生成溯源树,研究论文发展脉络
Chat Paper
正在生成论文摘要