Exponential Information Bottleneck Theory Against Intra-Attribute Variations for Pedestrian Attribute Recognition.
IEEE Trans. Inf. Forensics Secur.(2023)
摘要
Multi-label pedestrian attribute recognition (PAR) involves assigning multiple attributes to pedestrian images captured by video surveillance cameras. Despite its importance, learning robust attribute-related features for PAR remains a challenge due to the large intra-attribute variations in the image space. These variations, which stem from changes in pedestrian poses, illumination conditions, and background noise, make extracted attribute-related features susceptible to irrelevant information or noise interference. Existing PAR methods rely on body prior extractors or attention mechanisms to locate attribute-correlation regions for extracting robust features. However, these methods may not be robust to intra-attribute variations, which limits their effectiveness. To address this challenge, we propose a novel and flexible PAR framework that leverages the exponential information bottleneck (ExpIB) approach. Our ExpIB-Net uses mutual information compression as the main penalty during the early stage of training, thereby eliminating irrelevant information. As training progresses, the mutual information penalty weakens and the Binary Cross-Entropy Loss (BCELoss) contributes to improving the PAR recognition accuracy. Our method can also be integrated into an attention module to form the AttExpIB-Net, which better handles intra-attribute variations for better performance. Additionally, our model-agnostic ExpIB approach is plug-and-play, requiring no additional computational overhead during inference. Experiments on several challenging PAR datasets show that our method outperforms state-of-the-art approaches.
更多查看译文
关键词
Feature extraction, Pedestrians, Mutual information, Body regions, Training, Task analysis, Semantics, Pedestrian attribute recognition, intra-attribute variations, exponential information bottleneck
AI 理解论文
溯源树
样例
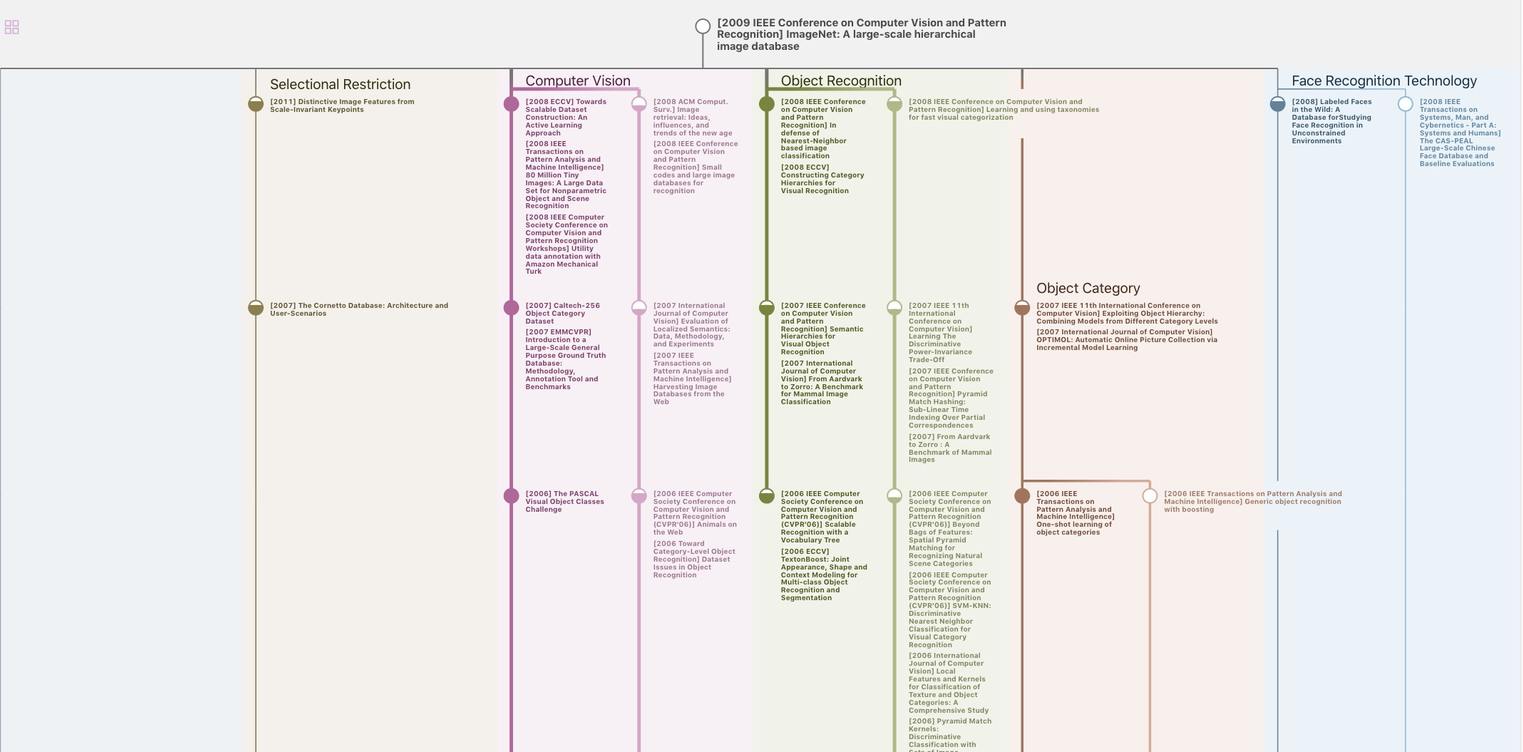
生成溯源树,研究论文发展脉络
Chat Paper
正在生成论文摘要