PGA-Net: Polynomial Global Attention Network With Mean Curvature Loss for Lane Detection
IEEE TRANSACTIONS ON INTELLIGENT TRANSPORTATION SYSTEMS(2023)
摘要
Lane detection is an important task in the field of automatic driving. Since lane lines usually have complex topologies and exist in various complex scenes (e.g., damaged lanes, severe occlusion, etc.), lane detection remains challenging. In this work, we propose a Polynomial Global Attention Network (PGA-Net) for lane detection, which is an end-to-end model for mining global road information and predicting lanes shape parameter formulas simultaneously. We model lane shape with cubic polynomial function and use the transformer-based DETR model to introduce the context information of lanes and roads to better regress the lane parameters. For polynomial curve modeling, we propose Mean Curvature Loss (MCL) to constrain the curvature of the predicted lanes, thereby enhancing the quality of curve lanes prediction. In addition, we design an improved supervision strategy to eliminate information bias between our parametric prediction methods and the labeling methods of lane datasets. Our method achieves state-of-the-art performance on two popular benchmarks (TuSimple and LLAMAS) and a most challenging benchmark (CULane), while exhibiting accelerated speed (>140fps on 3090 GPU, 28.9% improvement in average) and lightweight model size (<3M, an averaged 83.7% reduction).
更多查看译文
关键词
Lane detection,polynomial regression,global attention,aggregate contextual information,curvature loss
AI 理解论文
溯源树
样例
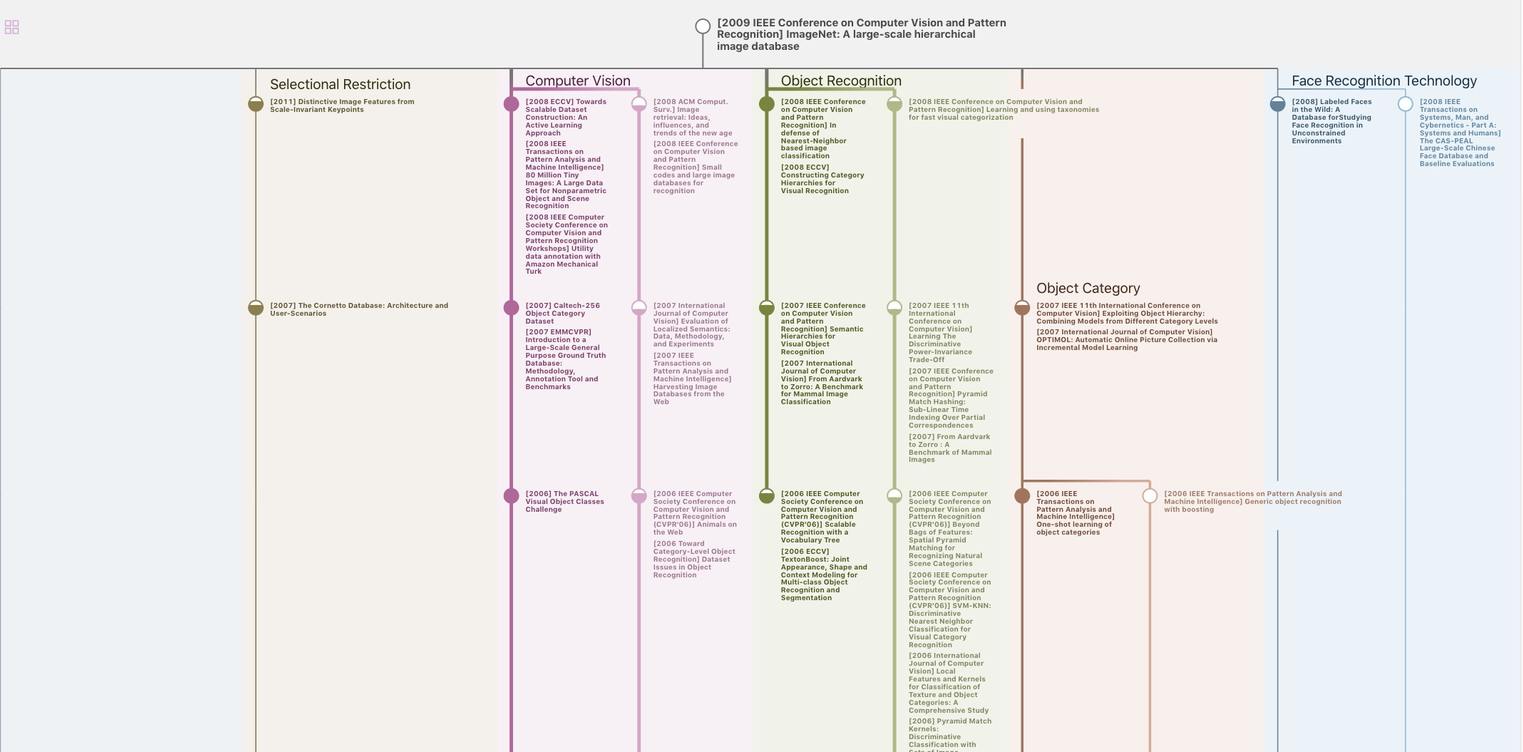
生成溯源树,研究论文发展脉络
Chat Paper
正在生成论文摘要