Functional order-reduced Gaussian Processes based machine-learning emulators for probabilistic constitutive modelling
COMPOSITES PART A-APPLIED SCIENCE AND MANUFACTURING(2023)
摘要
Machine learning methods have been extensively explored for constitutive relation that is essential in material and structural analyses. However, most existing approaches rely on neural networks, which lack interpretability and treat stress–strain data as discrete values, disregarding their inherent continuous nature. Therefore, this paper proposes novel functional order-reduced Gaussian Processes emulators, which are more interpretable by leveraging Bayesian theory and account for the uncertainty arising from microstructural homogenisation, providing the non-parametric probabilistic and continuous constitutive modelling of composite microstructure undergoing fracture/failure. Its most salient point is the capability of predicting the continuous and probabilistic stress–strain function only using limited (i.e., 400) samples, where the uncertain data is high-dimensional in large-scale composite (up to 250,000). An illustrative example demonstrates that the emulator accurately captures the probabilistic constitutive relation, providing insights into the maximum stress and strain values. Notably, the results highlight the significant variation in maximum stress due to fibre uncertainty. Moreover, the example showcases that as the fibre volume fraction increases from 0.4 to 0.6, the maximum stress tends to increase, while the maximum strain decreases, namely, more fibre results in higher strength and stiffness.
更多查看译文
关键词
Machine learning for probabilistic constitutive modelling,Functional Gaussian Process regression,Carbon fibre composite,Statistical properties/methods
AI 理解论文
溯源树
样例
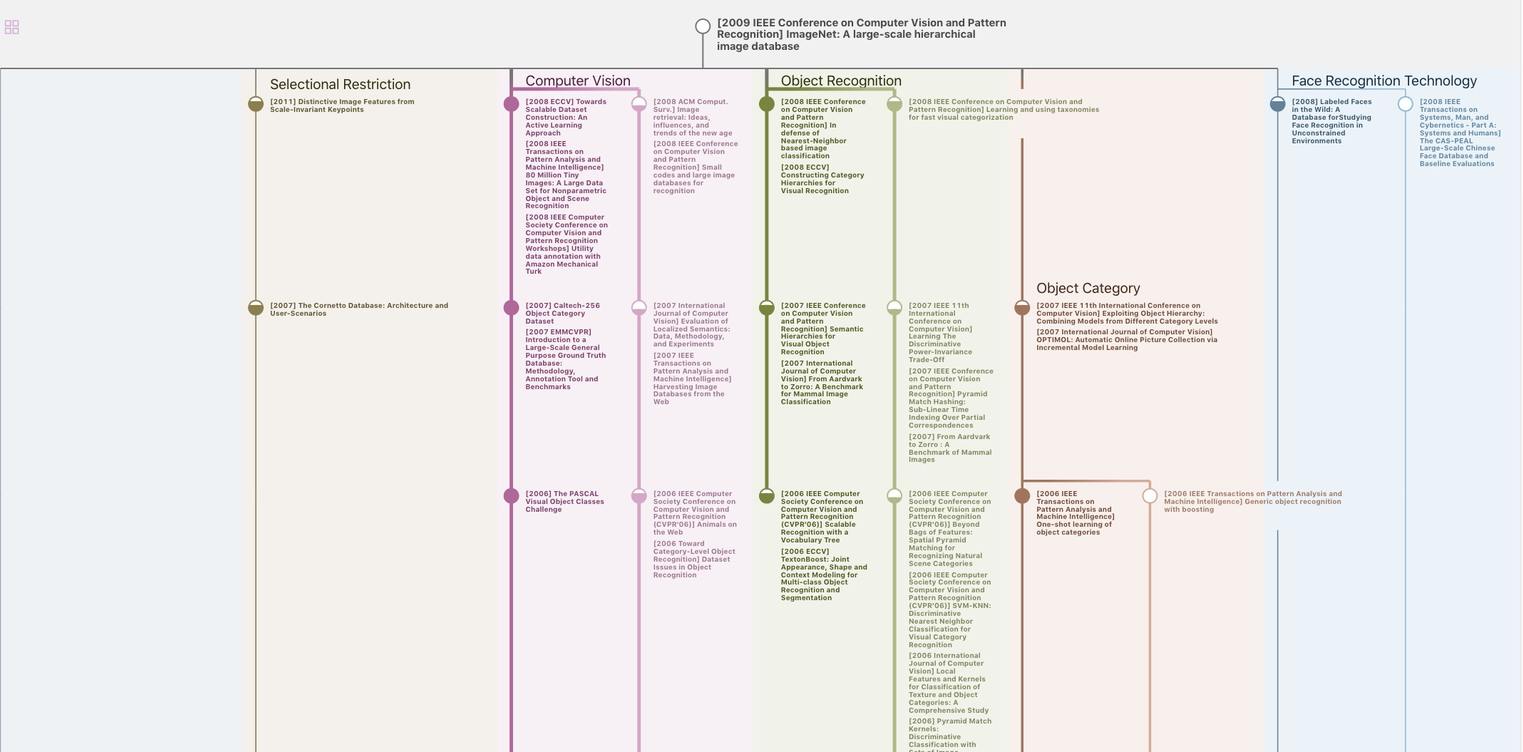
生成溯源树,研究论文发展脉络
Chat Paper
正在生成论文摘要