Science Autonomy and Planetary Missions: ML and Data Science Applied to the ExoMars Mission
2023 IEEE AEROSPACE CONFERENCE(2023)
Abstract
Future planetary science instruments will be capable of producing far more data than can be transmitted back to Earth, potentially leaving valuable scientific data on a planet's surface. Instruments will need to carefully identify the subset of total data to be prioritized for return, as transmission of the full data volume, even after compression, will not be feasible. The concept of science autonomy, where instruments collect measurement data, perform selected science data analyses onboard, and then autonomously act upon those analyses through self-adjustment and tuning of instrument parameters, can be used to identify and produce an optimal and compact data set for return, maximizing the value of each bit returned to Earth. Furthermore, the selection of the next operation(s) to be run following preliminary measurements, without requiring ground-in-the-loop communication, increases mission efficiency and enables successful yet shorter duration missions to hazardous planetary environments. This capability allows missions to prioritize the most compelling or time-critical data, yielding a more efficient and productive scientific investigation overall. In this paper, we present our implementation using different machine learning (ML) techniques (i.e., clustering, classification) for analyzing science data from the Mars Organic Molecule Analyzer (MOMA) instrument onboard the ExoMars rover Rosalind Franklin. MOMA is a dual-source (laser desorption and gas chromatography) mass spectrometer that will search for past or present life on the Martian surface and subsurface through analysis of soil samples. We use data collected from the MOMA flight-like engineering model to develop mass-spectrometry-focused ML techniques. This effort, in preparation for operating on Mars, is aimed at 1) helping the ExoMars science and operations team quickly analyze new data and support them in their decision-making process regarding subsequent operations and, 2) getting a better understanding of the challenges to enable science autonomy in future missions. We also present two significant challenges we faced in this development that are particular to space missions and will be common to most, if not all, robotic planetary missions. First, the lack of sufficient data volume from these unique and highly optimized instruments to train neural networks, and second the lack of sufficient results from the system to fully trust its output. To tackle the first challenge, we analyze the performance of ML algorithms after adding augmented data. We discuss adopting transfer learning techniques to fine-tune a NN trained on large amounts of commercial instrument data so that it can operate on our limited MOMA dataset. For the 'trust' challenge-as it is not always clear what we are looking for in planetary science-we must consider agile ML applications and demonstrate that these will not filter out potentially critical data. We will discuss our concept of a Trust Readiness Level for science autonomy akin to the NASA Technology Readiness Level. This initial project for advanced autonomy illustrates some key first steps of a longer-term objective to enable the spacecraft and instruments themselves to make real-time adjustments during operations as direct human oversight will not be possible for missions going further away in our solar system and beyond.
MoreTranslated text
Key words
commercial instrument data,compact data,compelling time-critical data,data science applied,efficient investigation,ExoMars mission,ExoMars rover Rosalind Franklin,ExoMars science,future planetary science instruments,hazardous planetary environments,highly optimized instruments,instrument parameters,Mars Organic Molecule Analyzer instrument,mass-spectrometry-focused ML techniques,measurement data,mission efficiency,MOMA,optimal data,planetary science-we,potentially critical data,productive scientific investigation,robotic planetary missions,science autonomy,selected science data analyses,shorter duration missions,space missions,sufficient data volume,total data,unique instruments,valuable scientific data
AI Read Science
Must-Reading Tree
Example
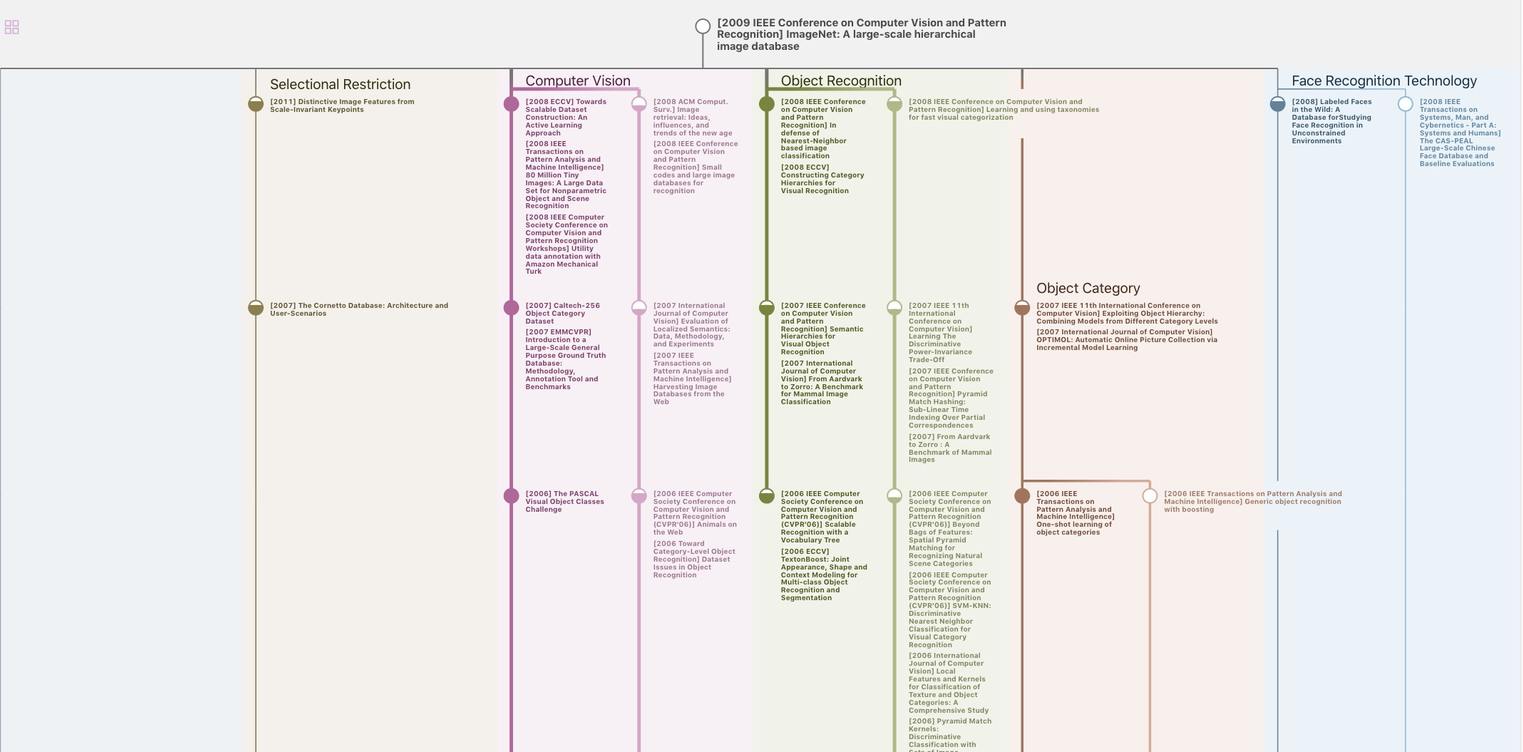
Generate MRT to find the research sequence of this paper
Chat Paper
Summary is being generated by the instructions you defined