Methodology Development of a Free-Flight Parameter Estimation Technique Using Physics-Informed Neural Networks
2023 IEEE AEROSPACE CONFERENCE(2023)
摘要
Unstable free-flight rigid body motion, consisting of 3D translational motion and large angular rates about all axes and orientations outside of typical flight envelopes, is a complex dynamical system with significant challenges in modeling and simulation. Current methods in predicting this type of flight rely on aerodynamic models dependent on large databases consisting of non-linear aerodynamic stability coefficients determined primarily through computational simulations and a combination of static and dynamic wind-tunnel tests. Due to the complex nature of this type of unstable free-flight motion, experimental flight data is needed to study the full dynamics and is well suited to aircraft system identification and parameter estimation, which has greatly affected how non-linear aerodynamic models are developed. While these methods have been proven effective in a wide array of flight conditions, significant research efforts are still going into improving these methods in extreme flight conditions similar to the fully unstable free-flight motion de-scribed. Similarly, the development of Physics-Informed Neural Networks (PINNs) has significantly changed scientific machine learning by introducing physical knowledge of a system directly into the neural network training. In this work, we develop three methods of using PINNs to extract aerodynamic parameters from flight data. First, Determinant PINNs consist of a trajectory network and a parameter estimation module which can be used to estimate parameters that vary due to a known or estimated functional form caused by changes in explanatory variables. Secondly, Non-Determinant PINNs consisting of a trajectory network and a parameter estimation network can be used to estimate parameters that vary due to a set of explanatory variables with no known form. Thirdly, a Modified Non-Determinant PINN is developed to demonstrate the use of a parameter estimation network independent of a trajectory network while still implementing physical constraints into the training process. Each method is introduced with simulated case studies based on 2D projectile motion. Then an F-16 non-linear 6-DOF simulation case study is used to demonstrate the potential application of PINNs as an aircraft parameter estimation and modeling tool.
更多查看译文
关键词
2D projectile motion,3D translational motion,aerodynamic parameters,aircraft parameter estimation,aircraft system identification,complex dynamical system,complex nature,computational simulations,Determinant PINN,dynamic wind-tunnel tests,experimental flight data,extreme flight conditions,free-flight motion de-scribed,free-flight parameter estimation technique,known estimated functional form,methodology development,modeling tool,modified nondeterminant PINN,neural network training,nonlinear aerodynamic models,nonlinear aerodynamic stability coefficients,parameter estimation module,parameter estimation network,physical constraints,physical knowledge,Physics-Informed Neural Networks,significant research efforts,simulated case studies,simulation case study,static wind-tunnel tests,trajectory network,typical flight envelopes,unstable free-flight motion,unstable free-flight rigid body motion
AI 理解论文
溯源树
样例
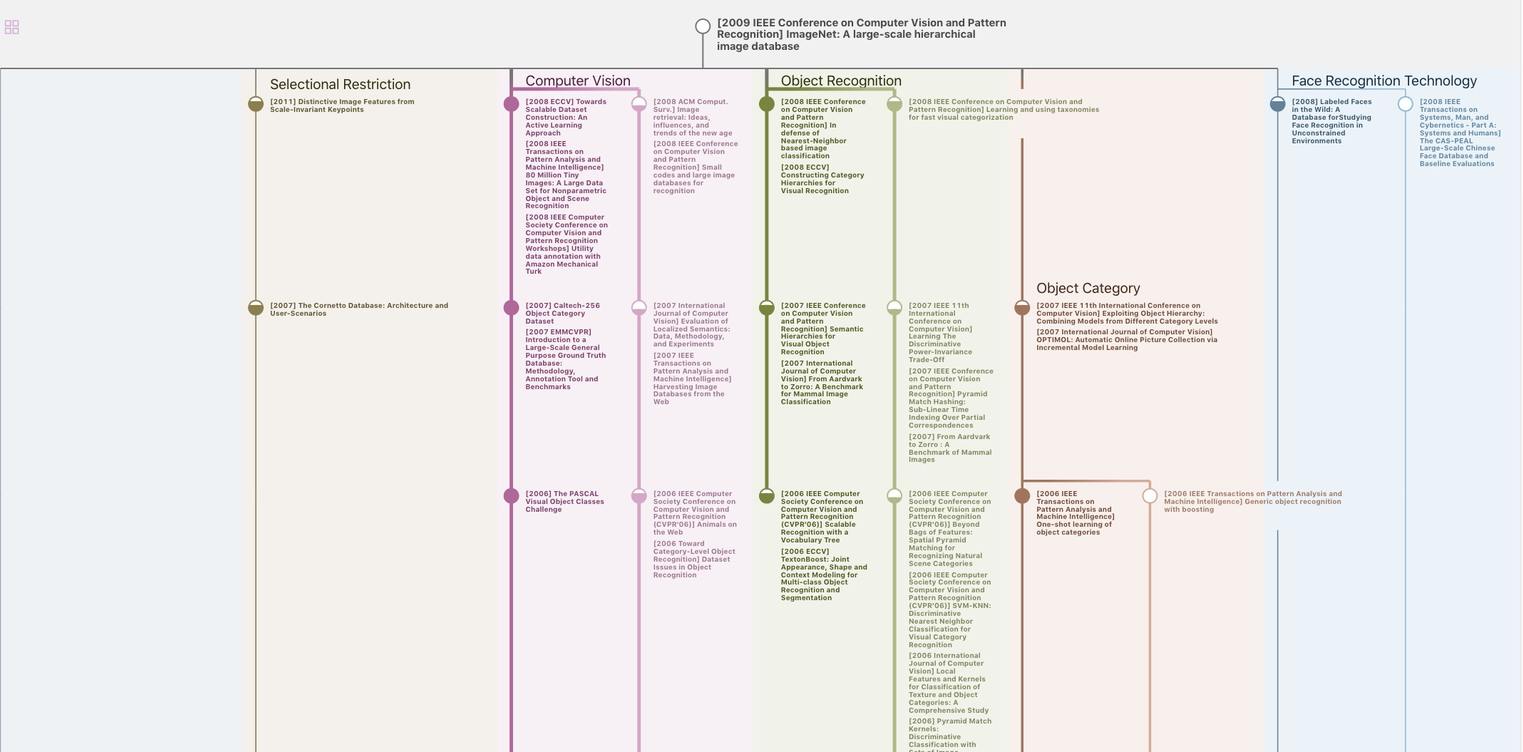
生成溯源树,研究论文发展脉络
Chat Paper
正在生成论文摘要