Predicting phases and hardness of high entropy alloys based on machine learning
INTERMETALLICS(2023)
摘要
The mechanical properties of high entropy alloys are highly dependent on phase structure. Therefore, accurate prediction of the phase structure is a guide to composition selection and performance enhancement, and it saves both experimental and financial expenditure. In this study, seven classification models were selected to predict the phase structure of high-entropy alloys and six regression models were selected to predict the hardness, and it was found that the XGB model performed best in both classification and regression predictions, where in the prediction phase structure, the XGB model has an error rate of 4.5% in the 10% prediction set, 11.6% in the 20% prediction set and 6.25% in the 30% prediction set. In predicting the hardness, the RMSE and MAE evaluation indexes of the XGB model are 52.66, 26.49. The δ (atomic radius difference) feature parameter having the strongest contribution to the phase formation in the high-entropy alloys. In addition, valence electron concentration(VEC), y/n BCC (The presence or absence of BCC) and y/n lm (The presence or absence of other phases) were key features affecting the hardness. Predictive analysis of two high-entropy alloys, AlCoCrFeNi and AlCoCrFeNiTi0.5, and a characterization effect map based on the Shapley additive explanations (SHAP) framework were developed to provide decision support for hardness assessment.
更多查看译文
关键词
Machine learning,High-entropy alloy,Phase structure,Hardness,SHAP framework
AI 理解论文
溯源树
样例
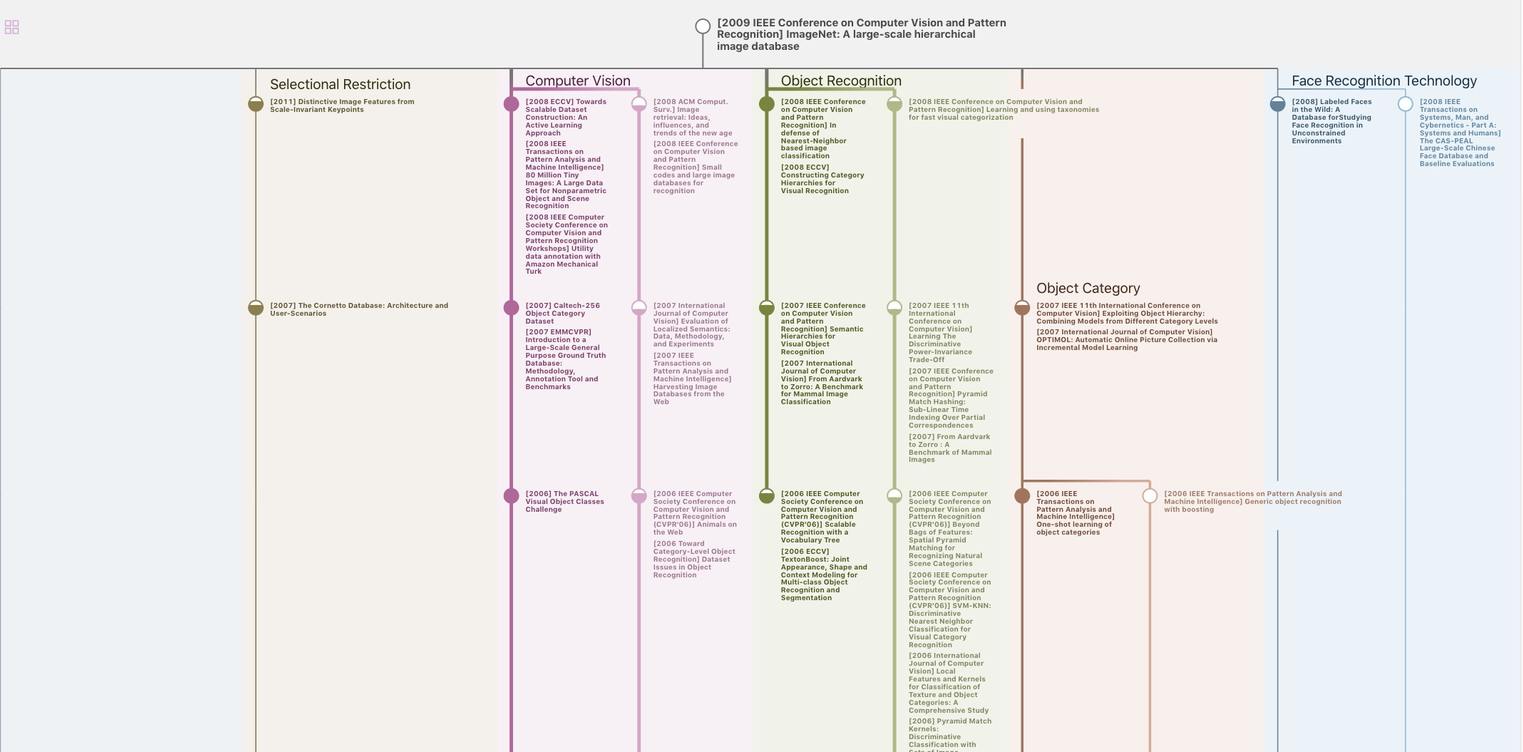
生成溯源树,研究论文发展脉络
Chat Paper
正在生成论文摘要