Graph attention U-Net to fuse multi-sensor signals for long-tailed distribution fault diagnosis
ENGINEERING APPLICATIONS OF ARTIFICIAL INTELLIGENCE(2023)
摘要
Due to the occasionality and unsustainable characteristics of mechanical faults, the collected data in real scenario is normally imbalanced that subsequently bottlenecks the appropriate fault recognition. In the actual operation of the equipment, there often exists many fault types, but the sample size of each fault is usually extremely unbalanced, which case is similar to the issue of long-tailed distribution. However, most existing approaches dealing with the long-tail problem seldomly utilize sensor structure information to reduce the imbalance impact and the commonly employed cost-sensitive losses fail to meet the comprehensively and complexity of the problem. To address these shortcomings, here we intend to construct a sensor network from the multi-sensor of the system and propose the graph attention U-Net (GATU-Net) to model the sensor network for the fault diagnosis featured with long-tailed distribution. Our proposed algorithm employs graph attention networks for the message passing and feature mining of structural relations among those sensors, and obtains favorable graph embeddings through the U-Nets architecture. In addition, a weighted shrinkage loss is proposed to allow the model to penalize the attention to low-valued samples and increase the attention to high-valued samples. Two experimental case studies unveil that GATU-Net is capable of achieving great performance for fault diagnosis with long-tailed distribution.
更多查看译文
关键词
Graph neural networks,Multi-sensor networks,Fault diagnosis,Long-tailed distribution
AI 理解论文
溯源树
样例
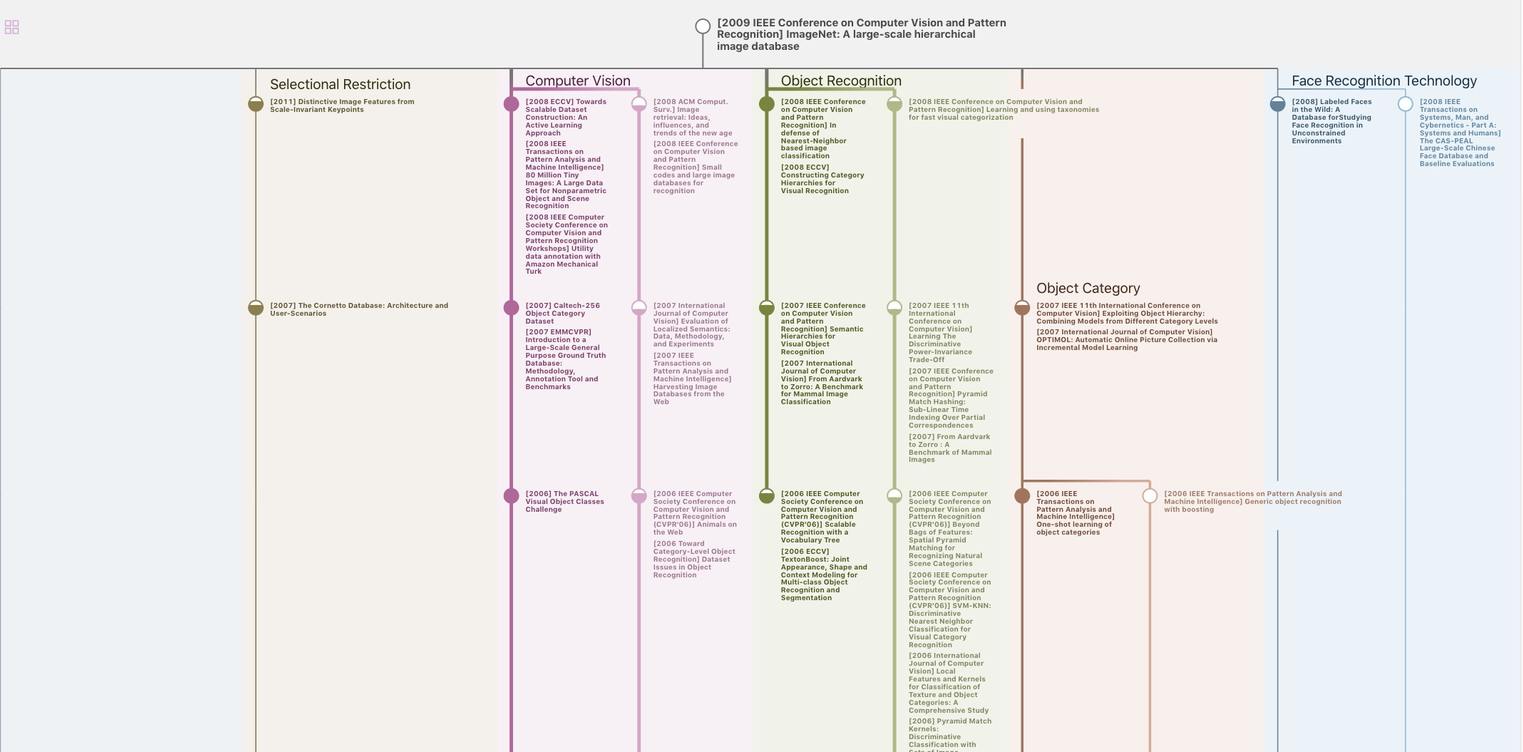
生成溯源树,研究论文发展脉络
Chat Paper
正在生成论文摘要