Domain consensual contrastive learning for few-shot universal domain adaptation
APPLIED INTELLIGENCE(2023)
摘要
Traditional unsupervised domain adaptation (UDA) aims to transfer the learned knowledge from a fully labeled source domain to another unlabeled target domain on the same label set. The strong assumptions of full annotations on the source domain and a closed label set of the two domains might not hold in real-world applications. In this paper, we investigate a practical but challenging domain adaptation scenario, termed few-shot universal domain adaptation (FUniDA), where only a few labeled data are available in the source domain and the label sets of the source and target domains are different. Existing few-shot UDA (FUDA) methods and universal domain adaptation (UniDA) methods cannot address this novel domain adaptation setting well. The FUDA methods would misalign the unknown samples of the target domain and the private samples of the source domain, and the UniDA methods cannot perform well with only a small number of labeled source samples. To address these challenges, we propose a novel domain consensual contrastive learning (DCCL) framework for FUniDA. Specifically, DCCL comprises two major components: 1) in-domain consensual contrastive learning aims to learn discriminative features from few labeled source data, and 2) cluster matching and cross-domain consensual contrastive learning aim to align the features of common samples in the source and target domains while keeping the private samples as private. We conduct extensive experiments on five standard benchmark datasets, including Office-31, Office-Home, VisDA-17, DomainNet, and ImageCLEF-DA. The results demonstrate that the proposed DCCL achieves state-of-the-art performance with remarkable gains.
更多查看译文
关键词
universal domain adaptation,domain adaptation,consensual contrastive learning,few-shot
AI 理解论文
溯源树
样例
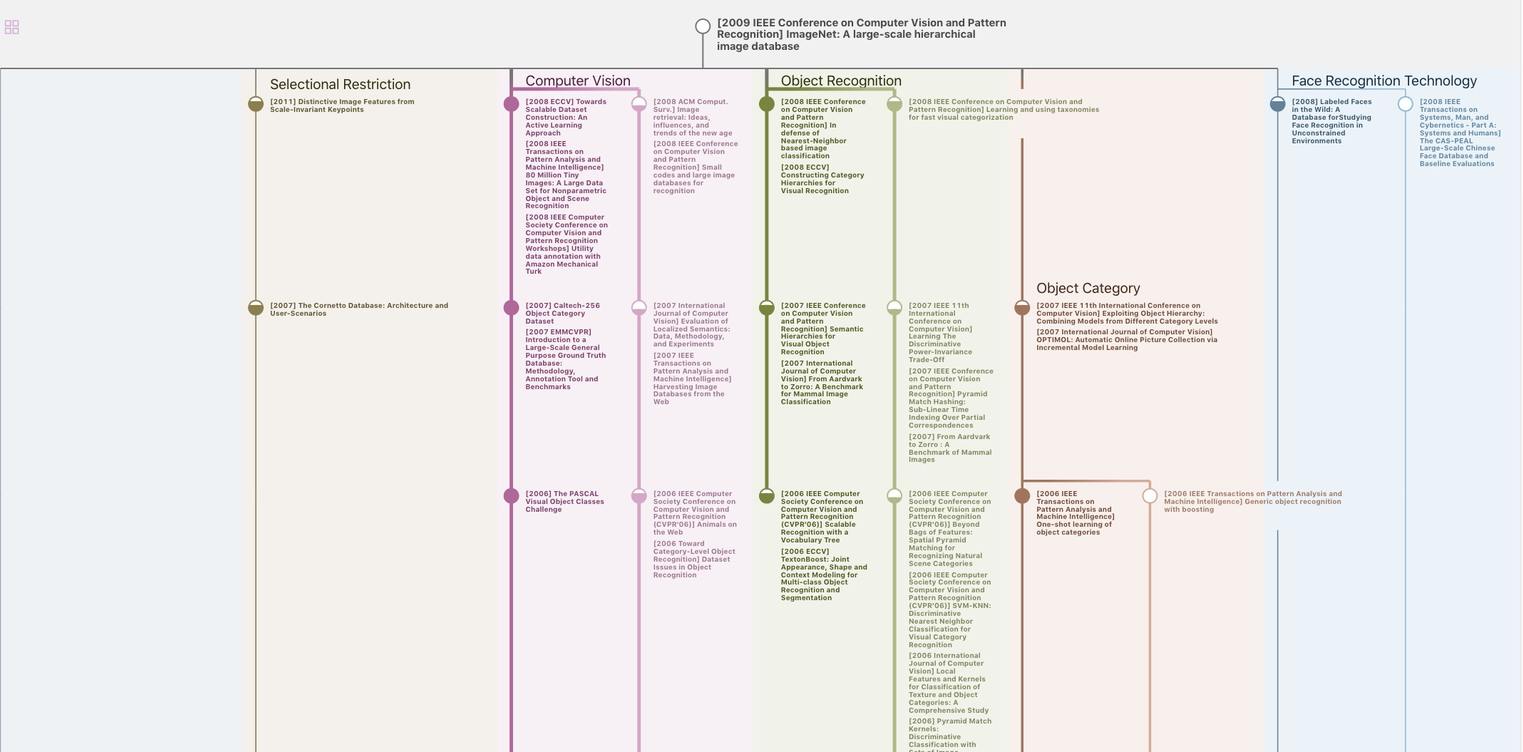
生成溯源树,研究论文发展脉络
Chat Paper
正在生成论文摘要