Revised Empirical Relations Between Earthquake Source and Rupture Parameters by Regression and Machine Learning Algorithms
PURE AND APPLIED GEOPHYSICS(2023)
摘要
In this study, we have developed new empirical relations between various source and rupture parameters such as moment magnitude (M), surface rupture length (SRL), subsurface rupture length (RLD), rupture width (RW), rupture area (RA), and average (AD) and maximum slip (MD), based on an extensive database. The study involves about 476 global earthquakes that occurred between 1857 and 2023, covering a range of magnitudes (≥ 4.5) and faulting styles. The results indicate that relations between M-SRL, M-RLD, M-RW, M-RA, M-AD and M-MD correlate well for all types of faulting compared with previous studies. However, log-linear regression may not account for the nonlinear behaviour of rupture parameters, and these equations are separately used for each fault parameter, which leads to inconsistency in magnitude prediction. Hence, machine learning technique has been used to estimate earthquake magnitudes using various fault parameters simultaneously, which ensures consistency. In this study, we have employed an artificial neural network (ANN) and gradient-boosting machine regression (GBM) and examined their performance and applicability. Our analysis shows that gradient-boosting machine learning estimates earthquake magnitude better than regression equations, but the artificial neural network outperforms both. The result of this study would be beneficial for paleoseismic studies where reliable estimates of earthquake magnitudes and other source parameters are often difficult to estimate.
更多查看译文
关键词
earthquake source,rupture parameters,regression,machine learning
AI 理解论文
溯源树
样例
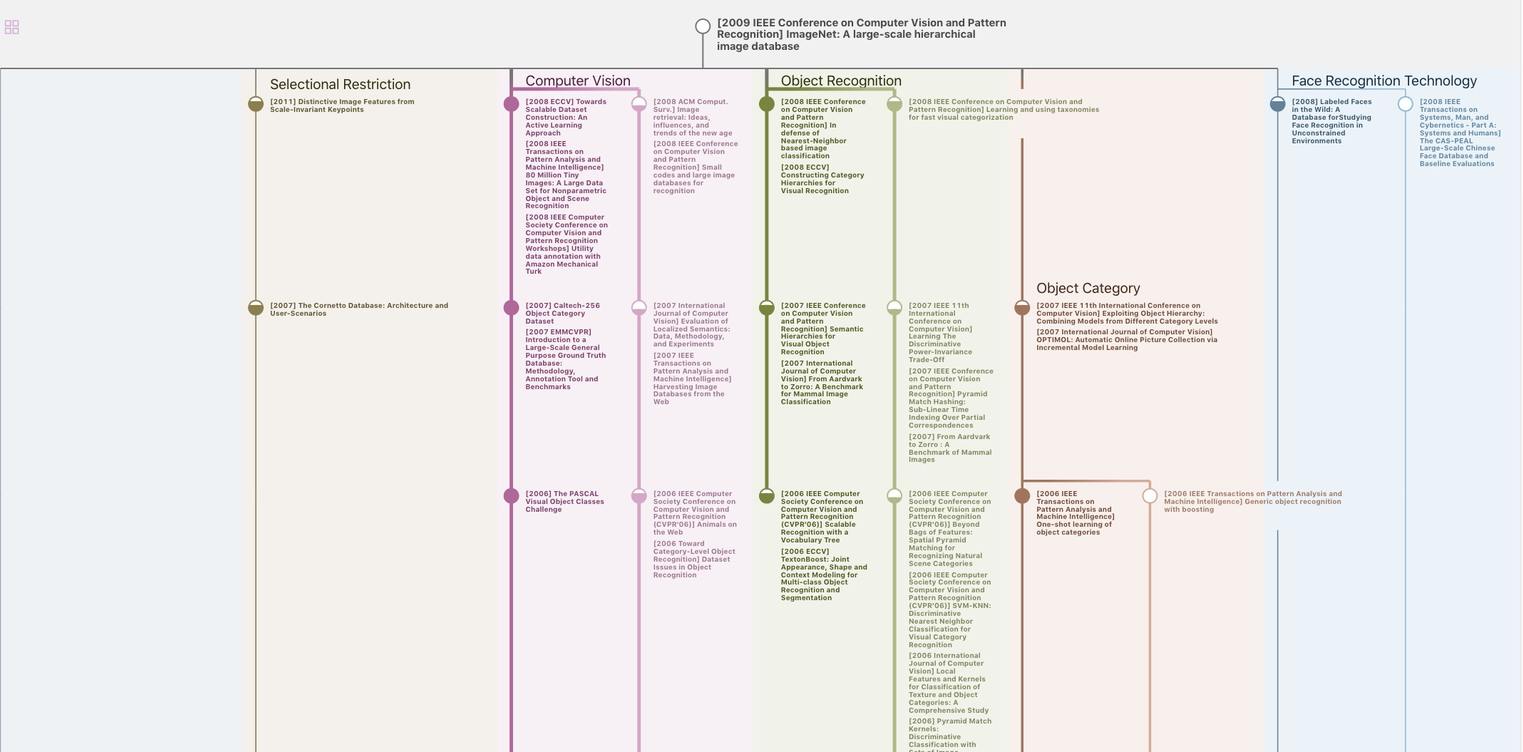
生成溯源树,研究论文发展脉络
Chat Paper
正在生成论文摘要