Adversarial Tradeoffs in Robust State Estimation
2023 AMERICAN CONTROL CONFERENCE, ACC(2023)
摘要
Adversarially robust training has been shown to reduce the susceptibility of learned models to targeted input data perturbations. However, it has also been observed that such adversarially robust models suffer a degradation in accuracy when applied to unperturbed data sets, leading to a robustness-accuracy tradeoff. Inspired by recent progress in the adversarial machine learning literature which characterize such tradeoffs in simple settings, we develop tools to quantitatively study the performance-robustness tradeoff between nominal and robust state estimation. In particular, we define and analyze a novel adversarially robust Kalman Filtering problem. We show that in contrast to most problem instances in adversarial machine learning, we can precisely derive the adversarial perturbation in the Kalman Filtering setting. We provide an algorithm to find this perturbation given data realizations, and develop upper and lower bounds on the adversarial state estimation error in terms of the standard (non-adversarial) estimation error and the spectral properties of the resulting observer. Through these results, we show a natural connection between a filter's robustness to adversarial perturbation and underlying control theoretic properties of the system being observed, namely the spectral properties of its observability gramian.
更多查看译文
AI 理解论文
溯源树
样例
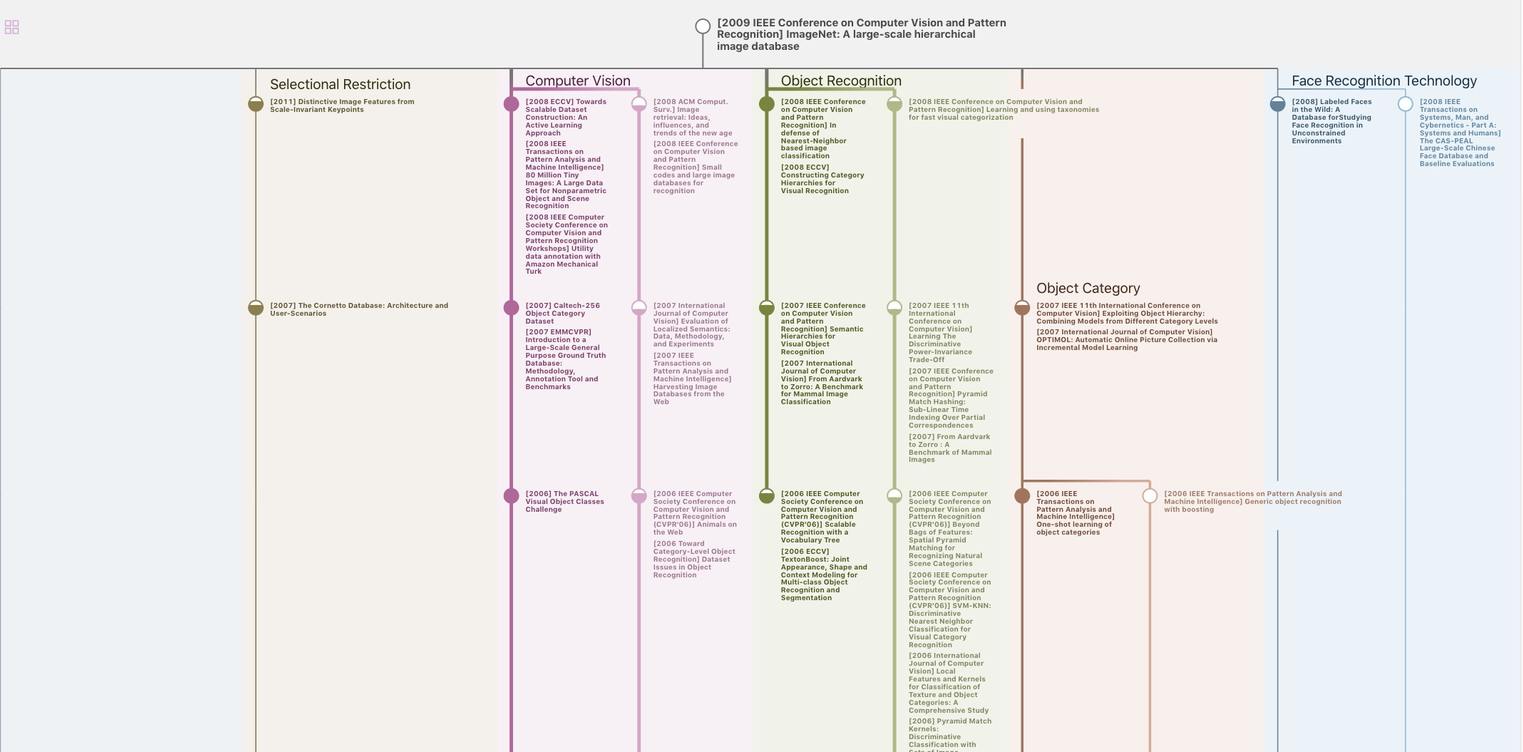
生成溯源树,研究论文发展脉络
Chat Paper
正在生成论文摘要