Stochastic modeling and statistical calibration with model error and scarce data
COMPUTER METHODS IN APPLIED MECHANICS AND ENGINEERING(2023)
摘要
This paper introduces a procedure to assess the predictive accuracy of stochastic models subject to model error and sparse data. Model error is introduced as uncertainty on the coefficients of appropriate polynomial chaos expansions (PCE). The error associated with finite sample size allows us to conceive of these coefficients as statistics of the data that we describe as random variables whose influence on output quantities of interest is evaluated through the extended polynomial chaos expansion (EPCE). A Bayesian data assimilation scheme is introduced to update these expansions by considering the resulting nested chaos expansion as a hierarchical probabilistic model. Stochastic models of quantities of interest (QoI) are thus constructed and efficiently evaluated. The Metropolis-Hastings Markov chain Monte Carlo procedure is used to sample the posterior. Two illustrative analytical and numerical problems are used to demonstrate the proposed approach. & COPY; 2023 Elsevier B.V. All rights reserved.
更多查看译文
关键词
Model error, Scarce data, Bayesian inference, Extended polynomial chaos expansion, Polynomial chaos coefficients, Uncertainty, quantification
AI 理解论文
溯源树
样例
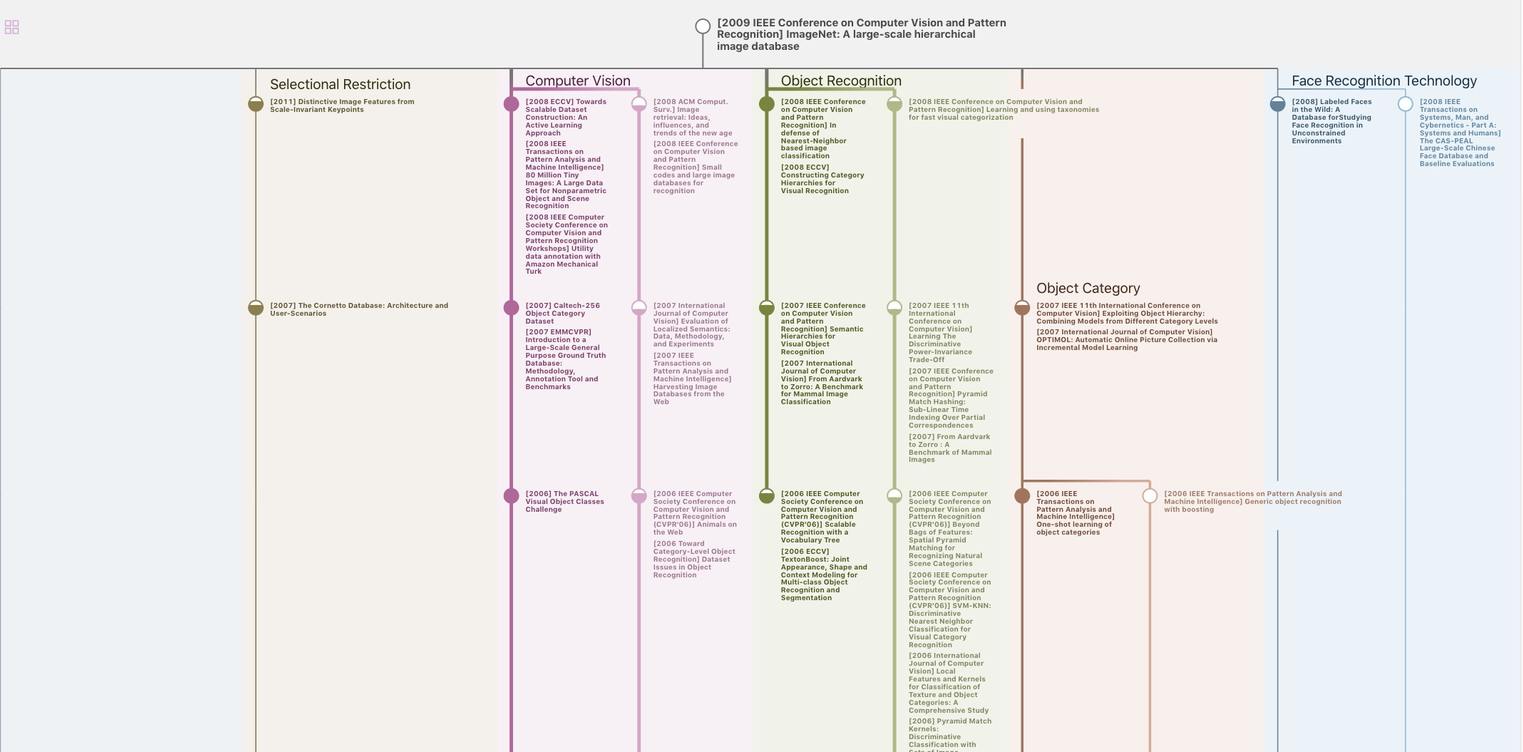
生成溯源树,研究论文发展脉络
Chat Paper
正在生成论文摘要