An optimized backpropagation neural network models for the prediction of nanomaterials concentration for purification industrial wastewater
ENGINEERING APPLICATIONS OF ARTIFICIAL INTELLIGENCE(2023)
摘要
In this paper, an optimized backpropagation neural network (BPNN) prediction models have been proposed to estimate the concentration of titanium dioxide (TiO2) nanomaterials required for the removal of Acid Black 24 dye, a type of textile dye, from industrial wastewater. The research gap addressed in this study lies in the investigation of various optimization algorithms for BPNN models and their profound impact on the accuracy of nanomaterial concentration predictions, thus contributing to the advancement of nanotechnology-based wastewater treatment research and its practical applications in industrial settings. Although several studies have predicted the amount of nanomaterial needed to degrade the dyes in industrial wastewater using BPNN, few have investigated the effects of optimized BPNN and how greatly the amount of degradation can vary. Therefore, six different optimization algorithms, including genetic algorithms (GAs), ant colony optimization (ACO), particle swarm optimization (PSO), evaluation strategy (ES), biogeography-based optimization (BBO), and population-based incremental learning algorithm (PBIL), are applied to optimize and evaluate the BPNN models. Experimental data obtained from chemical laboratory experiments are used to train and test the models, with different experiments conducted for various factors like TiO2, H2SO4, NaIO4, K2S2O8, dye, and silver nitrate. The performance of the optimized BPNN models is assessed using metrics such as mean squared error (MSE), root means squared error (RMSE), and R-squared (R2). The results demonstrate the effectiveness of the proposed models, with ACO yielding the best outcomes across all models. The R2 values for the TiO2, H2SO4, NaIO4, and K2S2O8 models after optimization indicate perfect predictions, whereas the R2 values before optimization ranged from 0.888 to 0.984. PSO performs well for most models except for K2S2O8, GA improves most models except for the silver nitrate model, ES enhances only the Dye model, and PBIL does not affect the TiO2 model. Overall, the optimized BPNN models exhibit impressive accuracy in estimating the TiO2 concentration required for removing Acid Black 24 dye from industrial wastewater.
更多查看译文
关键词
Machine learning,Nanotechnology,Water population,Industrial water degradation,Textile dyes,Optimized BPNN,ACO,PSO,GA,PBILL,ES,BBO
AI 理解论文
溯源树
样例
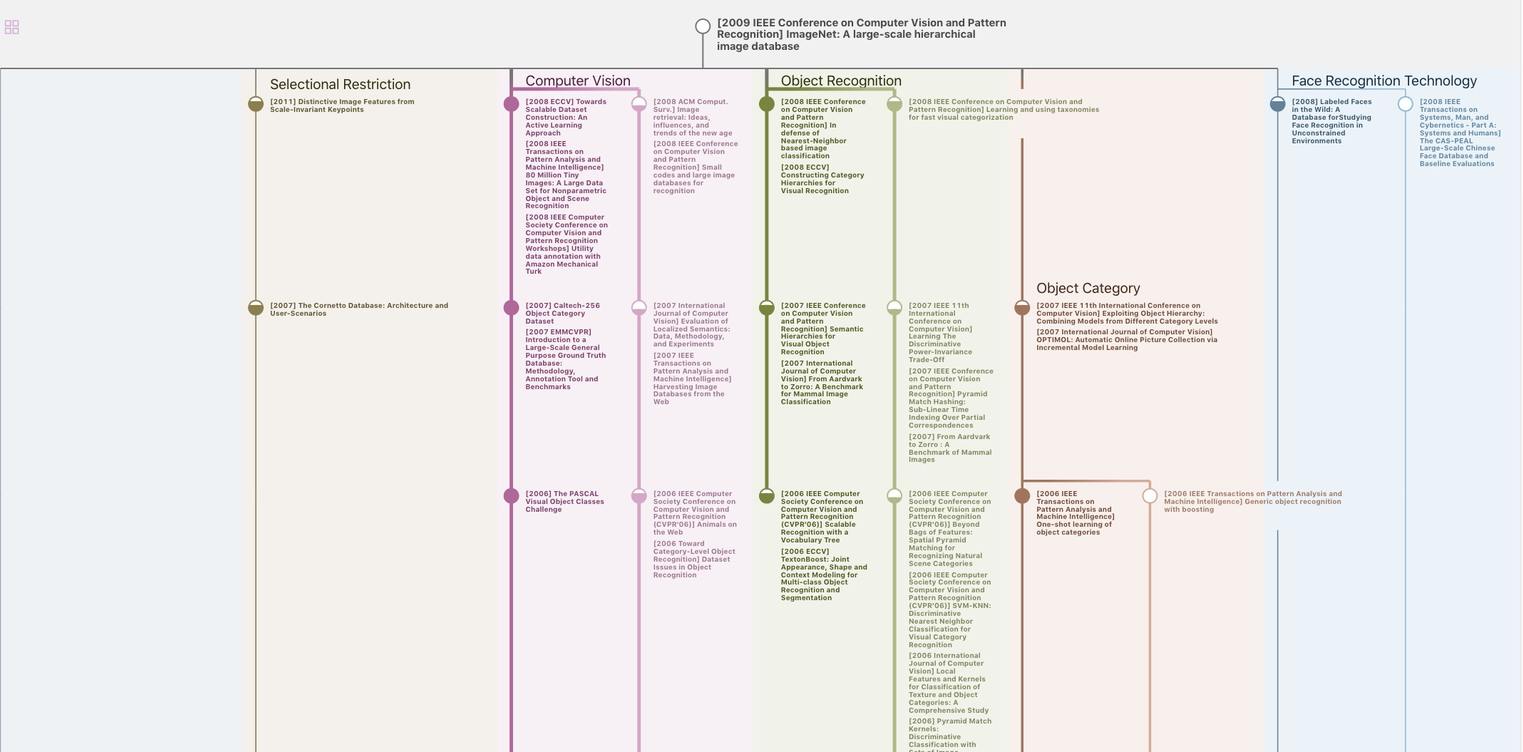
生成溯源树,研究论文发展脉络
Chat Paper
正在生成论文摘要