Generalized Bayes approach to inverse problems with model misspecification
INVERSE PROBLEMS(2023)
Abstract
We propose a general framework for obtaining probabilistic solutions to PDE-based inverse problems. Bayesian methods are attractive for uncertainty quantification but assume knowledge of the likelihood model or data generation process. This assumption is difficult to justify in many inverse problems, where the specification of the data generation process is not obvious. We adopt a Gibbs posterior framework that directly posits a regularized variational problem on the space of probability distributions of the parameter. We propose a novel model comparison framework that evaluates the optimality of a given loss based on its 'predictive performance'. We provide cross-validation procedures to calibrate the regularization parameter of the variational objective and compare multiple loss functions. Some novel theoretical properties of Gibbs posteriors are also presented. We illustrate the utility of our framework via a simulated example, motivated by dispersion-based wave models used to characterize arterial vessels in ultrasound vibrometry.
MoreTranslated text
Key words
parametric inverse problems,Gibbs posterior,uncertainty quantification,waveguide model
AI Read Science
Must-Reading Tree
Example
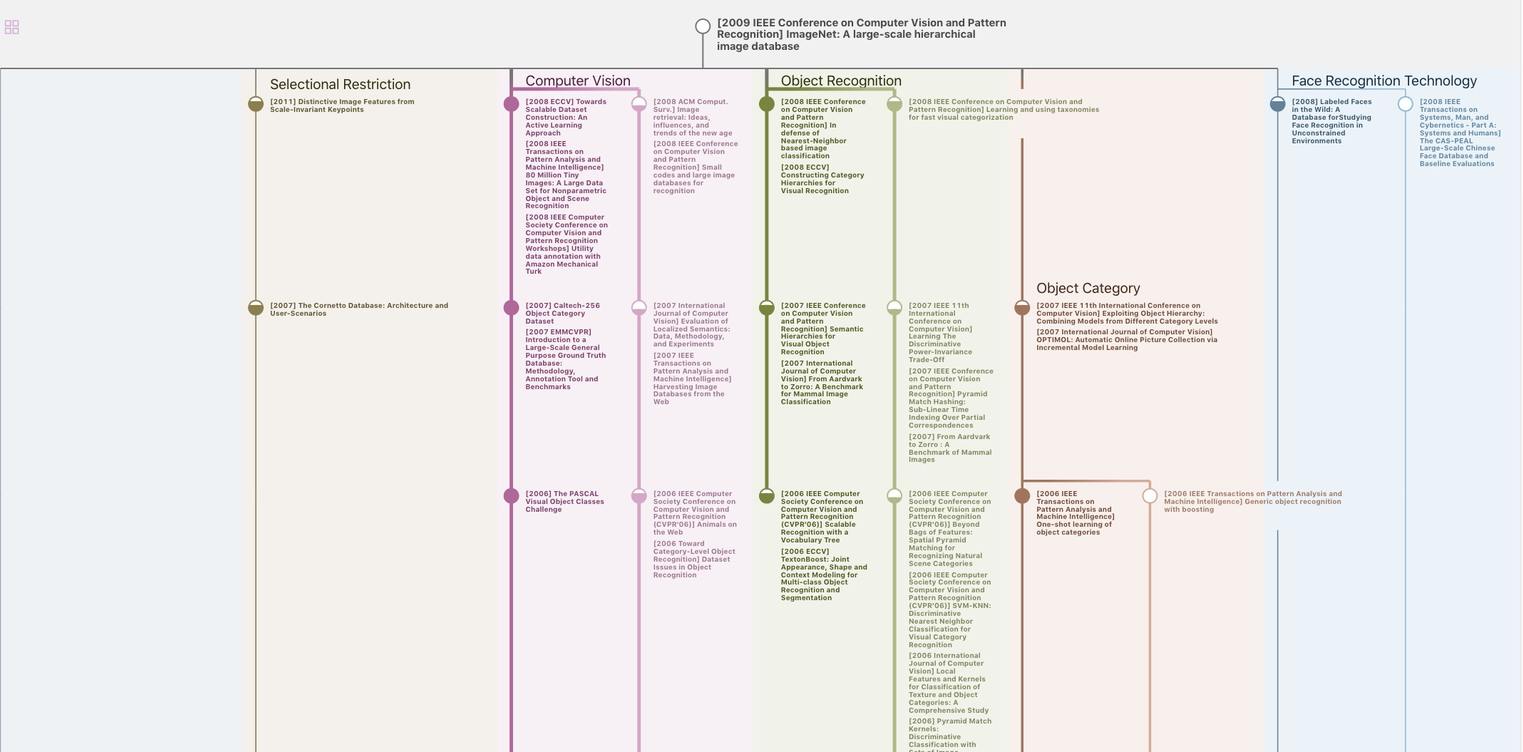
Generate MRT to find the research sequence of this paper
Chat Paper
Summary is being generated by the instructions you defined