Drivable Area Detection in Unstructured Environments based on Lightweight Convolutional Neural Network for Autonomous Driving Car
APPLIED SCIENCES-BASEL(2023)
Abstract
Road detection technology is an important part of the automatic driving environment perception system. With the development of technology, the situations that automatic driving needs to consider will become broader and more complex. This paper contributes a lightweight convolutional neural network model, incorporating novel convolution and parallel pooling modules, an improved network activation function, and comprehensive training and verification with multiple datasets. The proposed model achieves high accuracy in detecting drivable areas in complex autonomous driving situations while significantly improving real-time performance. In addition, we collect data in the field and create small datasets as reference datasets for testing algorithms. This paper designs relevant experimental scenarios based on the datasets and experimental platforms and conducts simulations and real-world vehicle experiments to verify the effectiveness and stability of the algorithm models and technical solutions. The method achieves an MIoU of 90.19 and a single batch time of 340 ms with a batch size of 8, which substantially reduces the runtime relative to a typical deep network structure like ResNet50.
MoreTranslated text
Key words
image segmentation,drivable area detection,autonomous driving car
AI Read Science
Must-Reading Tree
Example
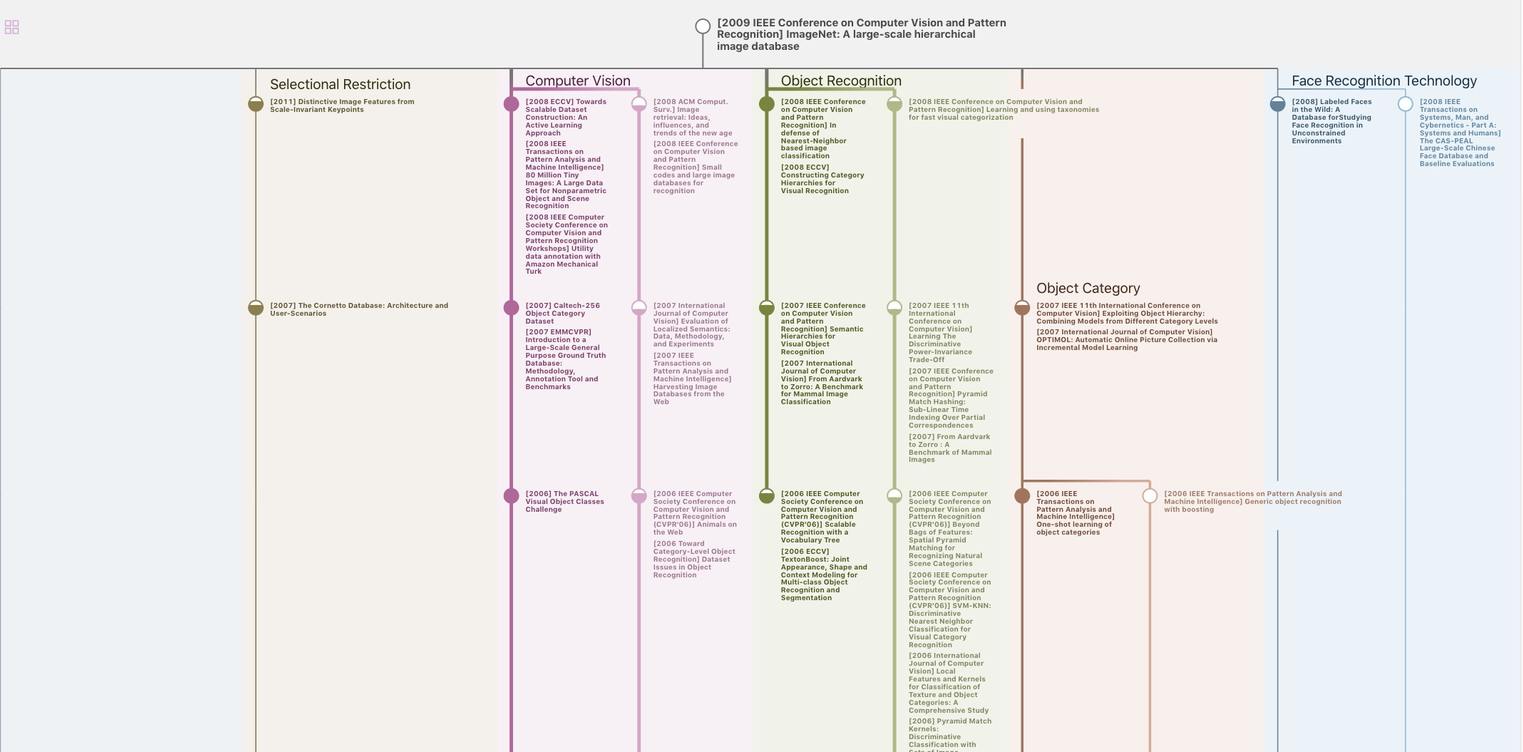
Generate MRT to find the research sequence of this paper
Chat Paper
Summary is being generated by the instructions you defined