Improved Sliding Mode Control for a Robotic Manipulator With Input Deadzone and Deferred Constraint
IEEE TRANSACTIONS ON SYSTEMS MAN CYBERNETICS-SYSTEMS(2023)
摘要
In this article, neural network (NN)-based sliding mode control schemes are proposed for an n-link robotic manipulator with system uncertainties, input deadzone, and external perturbations. A novel error-shifting function is proposed to release initial conditions. NNs are employed to approximate the unknown parameters of both system uncertainties and input deadzone. To update the sliding mode scheme, two advanced sliding mode surfaces with error-shifting function and barrier function are proposed to reduce the dependency of prior information and to realize a finite time convergence result, collectively. It should be pointed out that the proposed methods do not require initial states to satisfy the prescribed constraint caused by the barrier function and can be applied under unknown initial conditions. Furthermore, finite-time convergence for both tracking errors and NN weights is guaranteed. The effectiveness of the proposed schemes is demonstrated by simulation and experiments on the KINOVA robot.
更多查看译文
关键词
Adaptive sliding mode control (SMC), barrier Lyapunov function (BLF), deferred performance constraints, neural networks (NNs), robotic manipulator
AI 理解论文
溯源树
样例
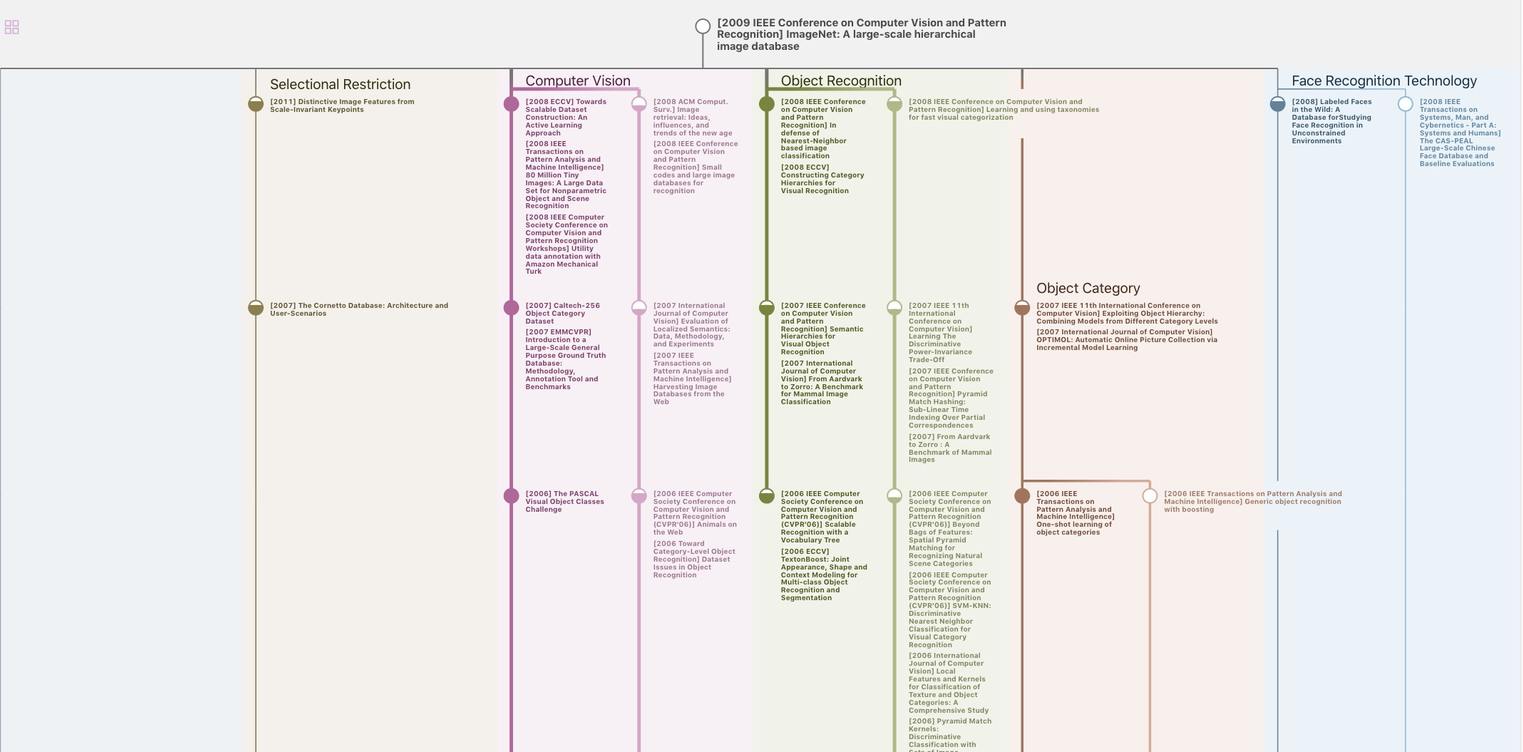
生成溯源树,研究论文发展脉络
Chat Paper
正在生成论文摘要