Traffic Modeling Based on Data-Driven Method for Simulation Test of Autonomous Driving
IEEE TRANSACTIONS ON INTELLIGENT TRANSPORTATION SYSTEMS(2023)
摘要
Traffic modeling is vitally important to the simulation test of autonomous driving, as on-road testing is not only tedious, inefficient, costly, and often unsafe. The fidelity of the traffic model is thus the key to achieving effective and efficient simulation test results. However, high-fidelity traffic modeling remains a challenge due to the complexity of vehicle movement and the dynamic spatiotemporal interactions among vehicles. In this paper, we propose a novel system-based traffic modeling approach that considers traffic as a whole, or a collection of all vehicles' movement and their interactions involved simultaneously. In addition, a long short-term memory (LSTM) encoding-decoding framework with a multi-head self-attention mechanism is proposed to capture the temporal dependency of vehicle motion and represent the vehicle-vehicle interaction. Furthermore, some traffic data are collected and used for model training purposes. A metric representing one of the traffic attributes, as the criterion for data screening, is proposed to describe the traffic complexity and disorder, or chaos. Experimental results demonstrate that the proposed model has achieved better performance compared with others from the literature.
更多查看译文
关键词
Simulation test,data-driven,traffic modeling,long short-term memory,self-attention
AI 理解论文
溯源树
样例
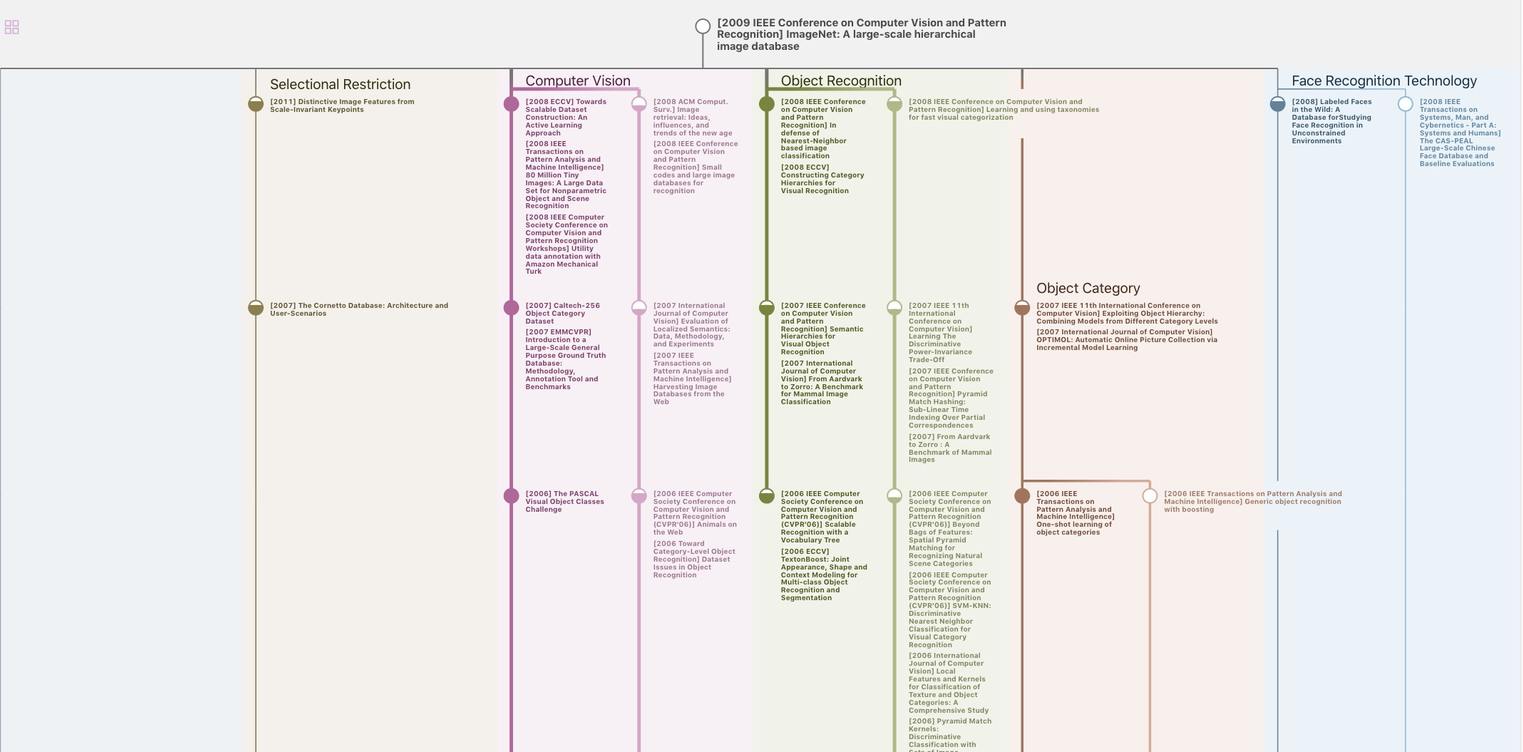
生成溯源树,研究论文发展脉络
Chat Paper
正在生成论文摘要