Landslide susceptibility assessment in Qinzhou based on rough set and semi-supervised support vector machine
EARTH SCIENCE INFORMATICS(2023)
摘要
As selection of landslide-causing indicators and training samples in landslide susceptibility assessment are the key factors in determining a model’s accuracy, the purpose of this research is to improve the accuracy of the landslide susceptibility assessment model by streamlining landslide-causing indicators and expanding training samples. To this end, rough set (RS) theory and genetic reduction method are adopted to reduce the initial 15 landslide-related indicators to 8 indicators highly correlated to landslide occurrence. Then, to tackle the problem of insufficient training samples, a semi-supervised classification method is employed to train the model classifier using labeled data and unlabeled data as mixed samples. Upon these, the landslide susceptibility assessment model of RS-SSVM is set up, with landslide susceptibility grades divided into high, medium, low, and non-prone zones in Qinzhou. Finally, the area under curve (AUC) values are used to compare and validate performances for different models. Analysis and comparison of the results denotes that the RS-SSVM method performed well as indicated by the AUC values of training datasets and verification datasets being 0.9308 and 0.9116. Meanwhile, the AUC values of RS-SSVM and SSVM are 0.9116 and 0.8522, respectively. This indicates that selecting more landslide-causing indicators does not necessarily bring better accuracy; instead, only the key impact indicators should be selected. Furthermore, overlay statistical analysis using historical landslide inventory data and assessment results shows that 71.27% of the historical landslides sites are in the high susceptibility areas accounting for 2.96% of the total area of the study area, which agrees well with the distribution features of historical landslides. Therefore, the proposed RS-SSVM method can improve the spatial cognition of the complex landslide systems aside from being applied to landslide susceptibility assessment in other places with similar regional geo-environmental conditions.
更多查看译文
关键词
Susceptibility assessment,genetic reduction algorithm,Semi-supervised classification,rough set (RS),Support vector machines (SVM)
AI 理解论文
溯源树
样例
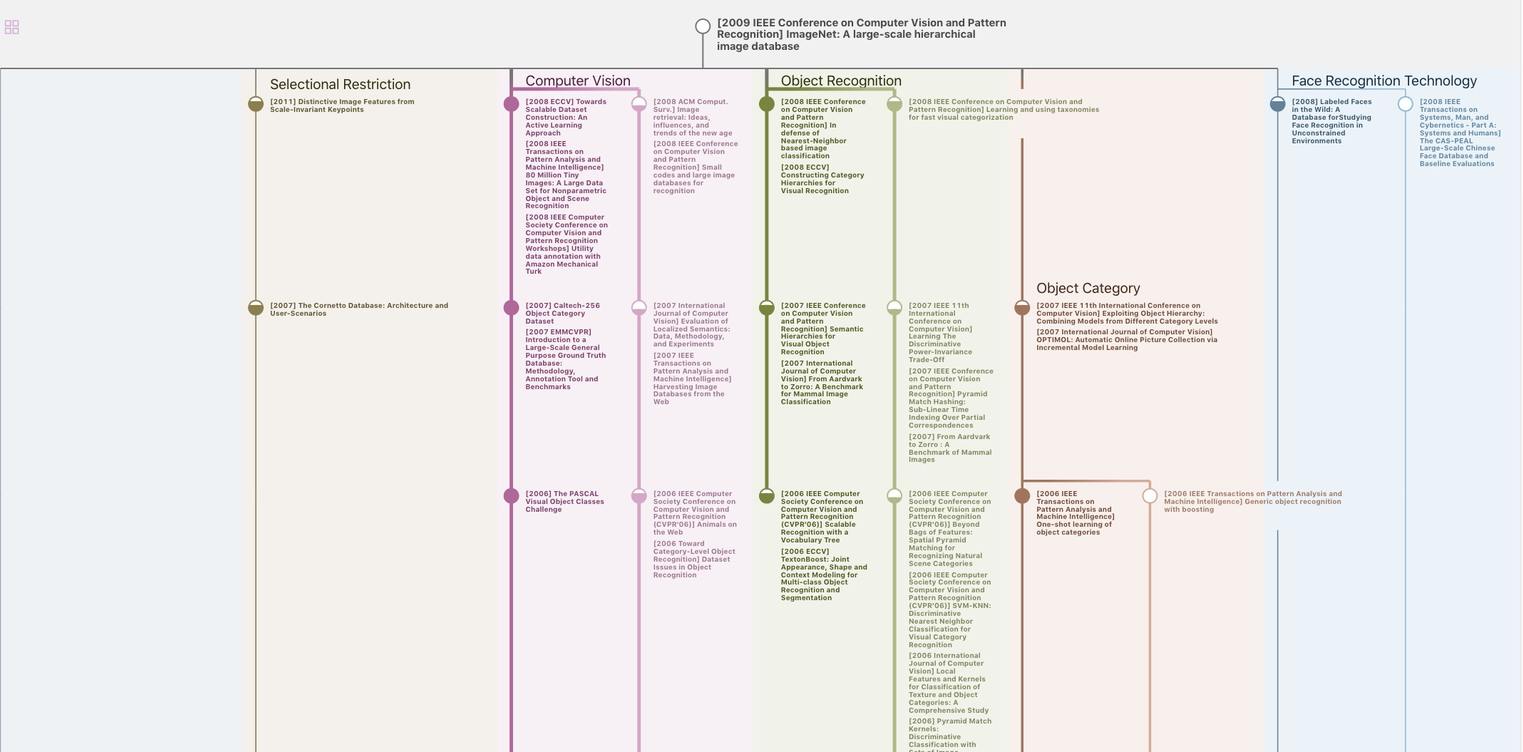
生成溯源树,研究论文发展脉络
Chat Paper
正在生成论文摘要