A Fault Diagnosis Method With Bitask-Based Time- and Frequency-Domain Feature Learning
IEEE TRANSACTIONS ON INSTRUMENTATION AND MEASUREMENT(2023)
摘要
Deep-learning-based methods used for fault diagnosis show remarkable performance, and these methods primarily learn features based on the time or frequency domain. Generally, time- and frequency-domain features are important for identifying faults. They can reflect the type and severity of faults, especially, frequency-domain features can reveal highly distinct patterns related to fault types. Thus, learning time- and frequency-domain features helps obtain comprehensive fault information and realize high accuracy for fault diagnosis. In addition, multitask learning (MTL) can learn features from many related tasks simultaneously. Therefore, in this study, MTL is employed for learning time- and frequency-domain features from two tasks (learning features from the time-domain and the frequency-domain). A fault diagnosis method using a bitask-based time- and frequency-domain feature learning network (TF-FLN) is proposed. The TF-FLN learns features through pretraining, and then the features are fed into a fault diagnosis network that is fine-tuned using labeled data. In particular, in the TF-FLN, task-specific loss functions are applied according to the characteristics of the two tasks. Moreover, the weight for each loss function is optimized automatically using a loss function optimizer. Compared with existing methods, the proposed method realizes a higher accuracy of 99.86% on the gearbox dataset and of 99.37% on the Paderborn University bearing dataset. Furthermore, experimental results demonstrate the effectiveness of MTL and the low computation cost of the proposed method.
更多查看译文
关键词
fault diagnosis method,frequency domain feature learning,fault diagnosis,bitask-based
AI 理解论文
溯源树
样例
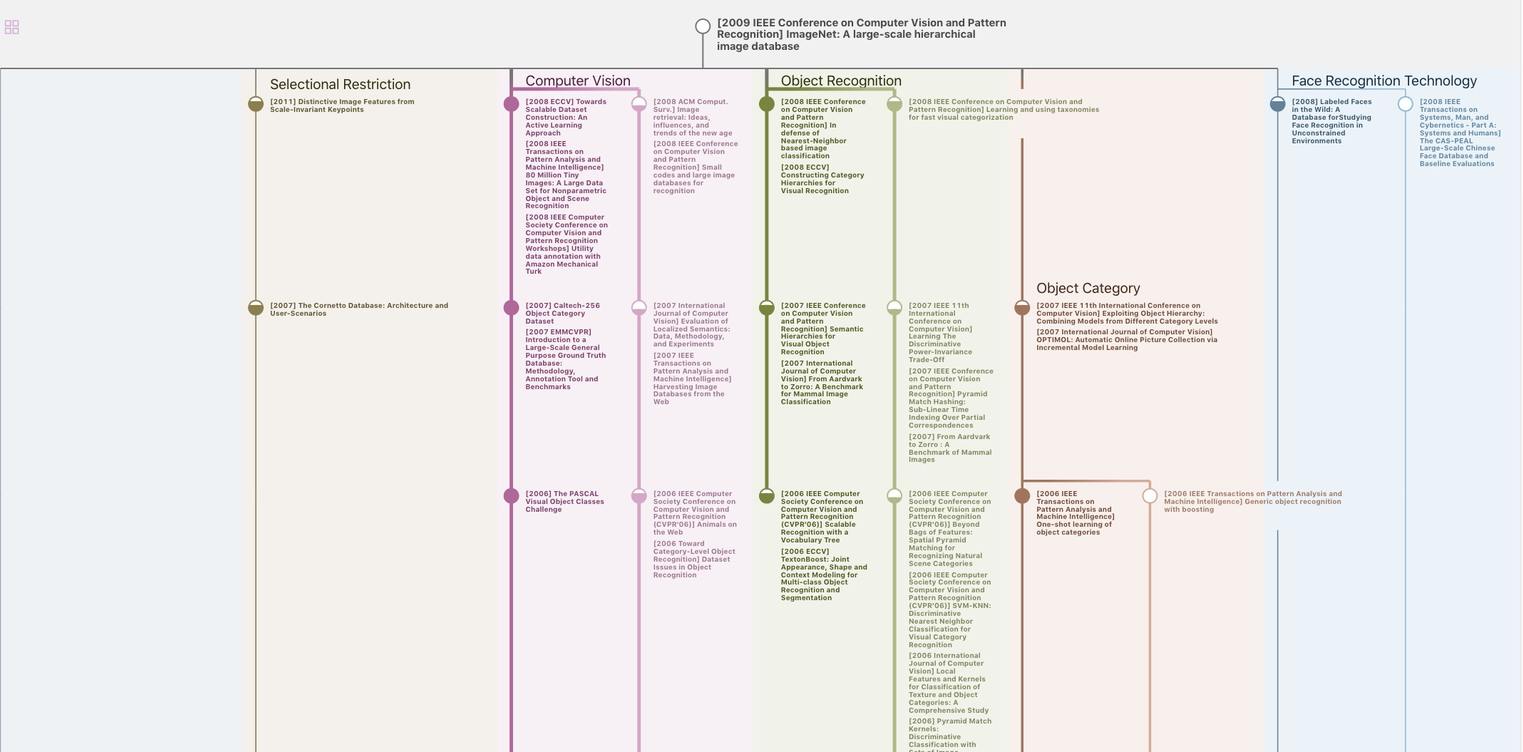
生成溯源树,研究论文发展脉络
Chat Paper
正在生成论文摘要