In silico approaches to patient selection: Credentialing elraglusib as a novel treatment in metastatic melanoma resistant to checkpoint inhibitors.
JOURNAL OF CLINICAL ONCOLOGY(2023)
摘要
3079 Background: Elraglusib (9-ING-41), a novel inhibitor of GSK-3β, has been evaluated in >230 patients (pts) with advanced malignancies including metastatic melanoma in the 1801 phase 1/2 trial (NCT03678883). Pts with melanoma treated with single agent elraglusib demonstrated clinical benefit including a durable complete response despite multiple prior lines of therapy including checkpoint inhibitors (CPI). Methods: We collected data from numerous -omic modalities in the 1801 trial to characterize markers of elraglusib response with bioinformatics and machine learning (ML). Genomic panels were collected on 105 pts. The most frequent tumor types included colorectal cancer (n=35), pancreas (n=31), lung (n=15) and metastatic melanoma (n=12). Pts were treated with elraglusib as a single agent or in combination with chemotherapy. Pathway based features were generated by quantifying the percent of each Reactome pathway that was mutated. These pathway features were iteratively evaluated for their performance in shallow neural networks predicting patient RECIST with each model using 5-15 randomly selected features at a time. Publicly available genomic data from an anti-PD-1 trial in patients with metastatic melanoma (Hugo et al., 2017) was transformed into pathway-based features and input into the final model to predict elraglusib response in this population. Results: Our final pathway based genomic model for elraglusib response performed with a training accuracy of 93% and a test accuracy of 88% on the 1801 patients. We then tested the model on metastatic melanoma patients who were treated with anti-PD-1 therapy. We found that patients from Hugo et al. were predicted to respond to elraglusib (22/26). In addition, most patients with tumors resistant to anti-PD-1 therapy were also predicted to respond to elraglusib (10/12). Finally, we tested the model on metastatic melanoma patients treated with elraglusib in the 1801 study that had failed CPI and for whom -omic data was available. The model correctly identified 5/5 patients that demonstrated clinical benefit (1 CR, ongoing>1400 days; 4 prolonged OS of 107, 256, 357, and 556 days). Conclusions: We applied robust in-silico modeling developed using 1801 trial data to develop a rationale for the development of elraglusib in the treatment of metastatic melanoma including patients that failed or were refractory to anti-PD-1 treatment. This model correctly identified metastatic melanoma patients in 1801 with good clinical outcomes that had failed prior CPI treatment. Further, we present this in silico approach as a robust way to repurpose prior clinical study genomics and ML modeling for population enrichment during the clinical development of novel drugs. Clinical trial information: NCT03678883 .
更多查看译文
关键词
metastatic melanoma,credentialing elraglusib,patient selection,silico approaches
AI 理解论文
溯源树
样例
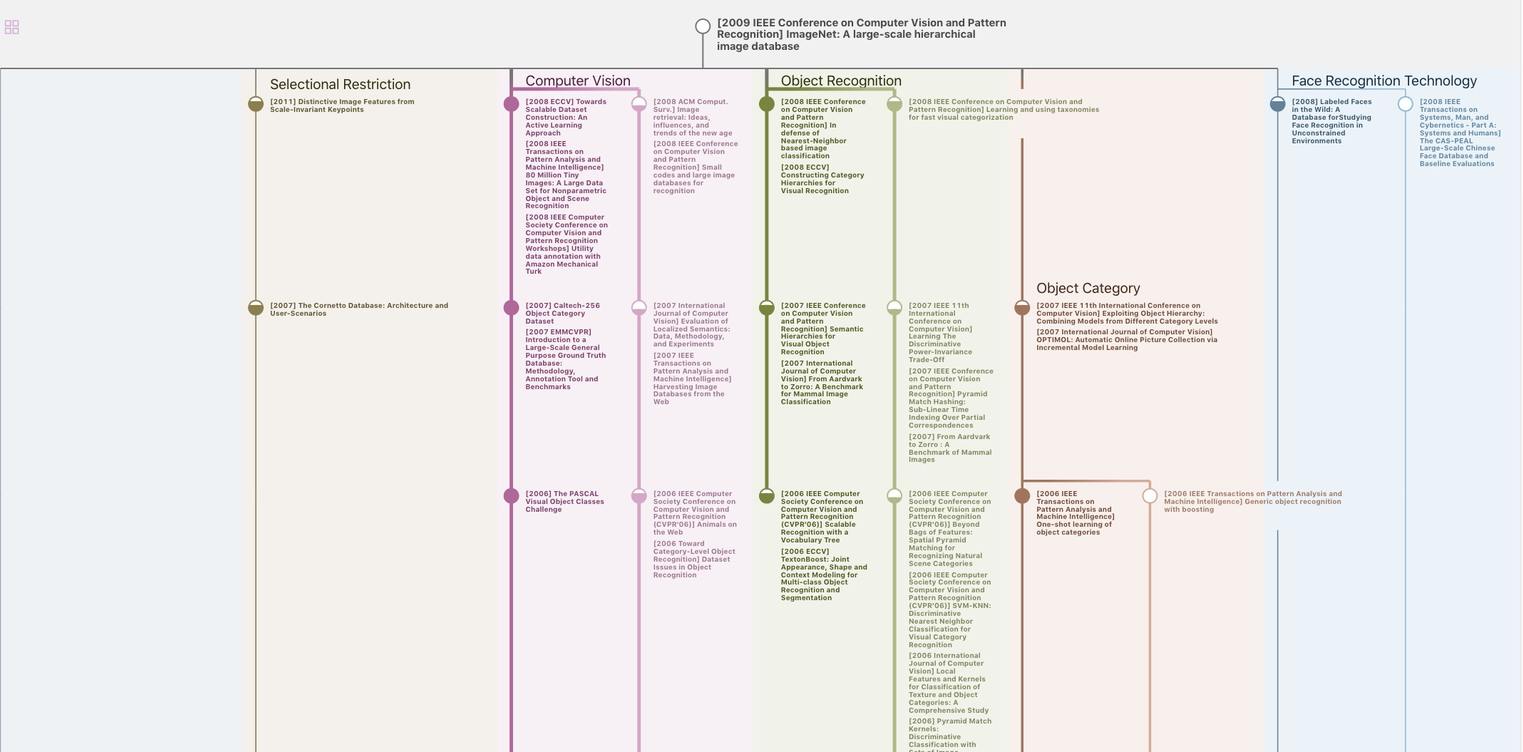
生成溯源树,研究论文发展脉络
Chat Paper
正在生成论文摘要