The validity of a machine learning algorithm in predicting response to immune checkpoint inhibitors in melanoma.
JOURNAL OF CLINICAL ONCOLOGY(2023)
摘要
9523 Background: We previously reported the development of a machine learning algorithm to predict immunotherapy response in patients with advanced melanoma using retrospectively collected pretreatment histologic slides (Johannet et al, CCR 2021). In this study, we tested the validity of the same algorithm in two independent cohorts of melanoma patients enrolled in phase III clinical trials of immune checkpoint inhibitors (ICI), one metastatic, and one adjuvant. Methods: We examined 336 patients enrolled in CheckMate 067(NCT01844505) and CheckMate 238 (NCT02388906). We used deep convolutional neural networks (DCNN) to automatically segment images into three regions of interest: tumor, lymphocytes, and stroma. The algorithm was previously trained and tested to predict the complete and partial response (CR/PR) versus progression of disease (POD) and to predict the probability of progression-free survival (PFS) while on ICI. We tested the performance in the two cohorts; the first (CheckMate 067) included 164 patients with stage III/IV unresectable melanoma who received ipilimumab (n= 49), nivolumab (n = 59), or combination (n= 56) therapy as a first line, then tested whether the algorithm could perform as well in the second cohort (CheckMate 238) which included 172 patients with stage III/IV resectable melanoma who received adjuvant ipilimumab (n = 87) or nivolumab (n = 85) therapy as first line. Results: The segmentation classifier identified tumor within slides with an area under the curve (AUC) of 0.99. A combined model of DCCN and treatment predicted POD with an AUC of 0.72 in metastatic melanoma patients enrolled in Checkmate 067. The DCCN classified patients into high and low risk based on their likelihood of PFS (P <0.0001). However, the same algorithm is not effective in predicting recurrence in patients treated in the adjuvant setting in Checkmate 238 (AUC 0.52). Retraining and testing using only patients treated in the adjuvant setting improved the AUC average to 0.65-0.70. Conclusions: Our results show the reproducibility of our previously developed algorithm in predicting response in patients with advanced metastatic melanoma. The inability of the model to predict recurrence in patients treated in the adjuvant setting might reflect the difference in the endpoint tested (response versus recurrence), which requires further training on larger datasets for this particular endpoint prediction, and/or the limited tumor volume in resected disease below a threshold that machine learning can predict a difference.
更多查看译文
关键词
immune checkpoint inhibitors,melanoma,machine learning
AI 理解论文
溯源树
样例
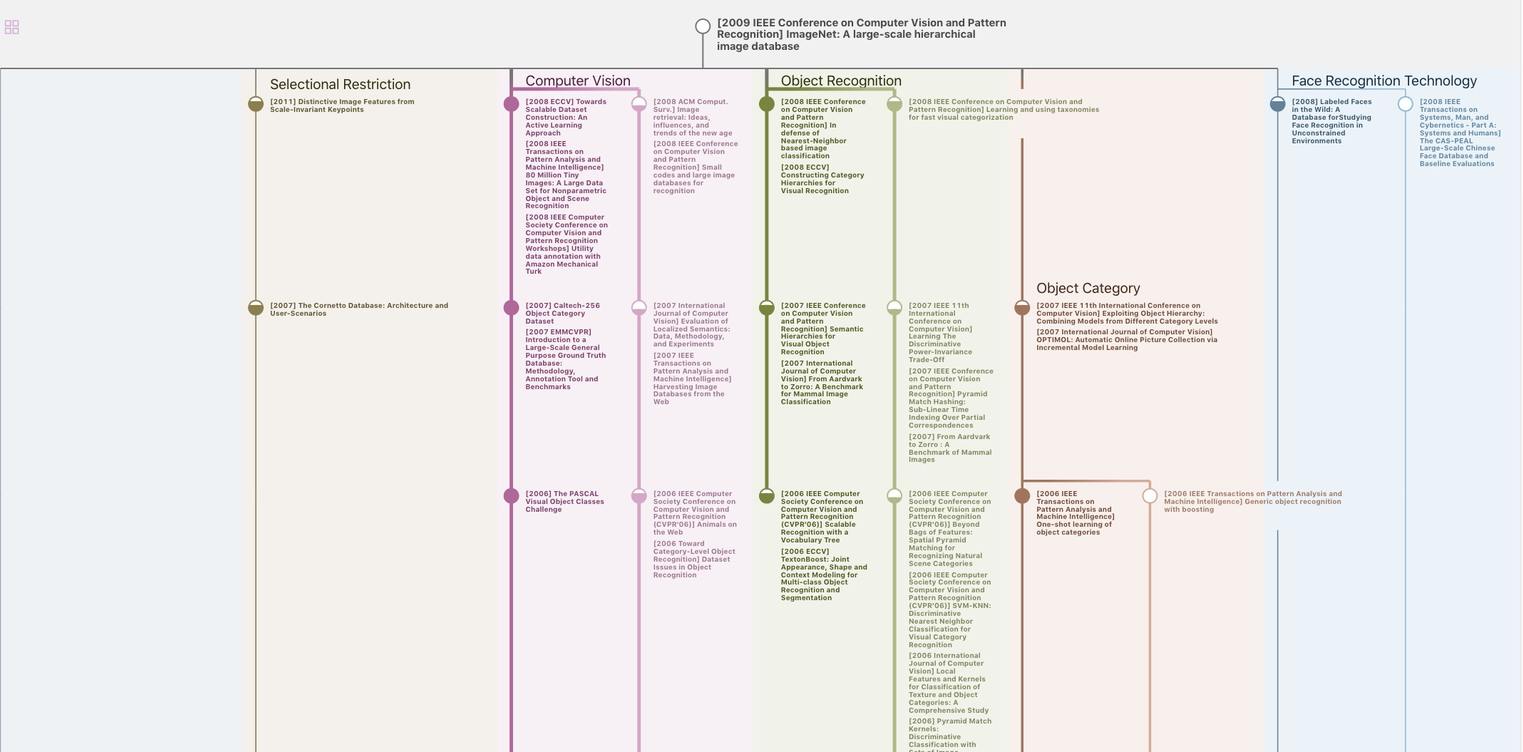
生成溯源树,研究论文发展脉络
Chat Paper
正在生成论文摘要