Fully automated prostate cancer identification using multiplex fluorescence immunohistochemistry to assess prognosis markers on a single tissue section
JOURNAL OF CLINICAL ONCOLOGY(2023)
摘要
e17118 Background: Most prostate cancers diagnosed are low to intermediate risk and face the decision on whether active surveillance or active treatment represents the optimal treatment option. To incorporate a reproduceable parameter in treatment planning, prognostic marker assessment using multi-gene panels has been frequently proposed. However, the fluctuating tumor purity can reduce the predictive value of such tests. A more accurate prognostication would be the quantification of prognosis markers exclusively on cancer cells. Methods: To enable automated prognosis marker quantification, a framework for automated prostate cancer detection that comprises three different artificial intelligence analysis steps and an algorithm for cell-distance analysis have been developed and validated using 8 marker BLEACH&STAIN mfIHC (i.e., panCK, p63, PSA, PSMA, INSM1, AR, Ki-67, CD56) in a tissue microarray and prostate biopsy cohort of 10’234 prostate cancers. Results: The approach for automated prostate cancer detection mimics the way pathologists use for identification of cancer cells and identified epithelial cells (CKpan positive) that were non-adjacent to basal cells (p63 positive). Validation experiments revealed that the optimal distance between p63 + basal cells and benign panCK + cells was 23 µm to exclude benign glands from the prognosis marker analysis. A distance of 23µm as threshold for automated cancer detection showed a 39% higher accuracy compared to the cutoff value 5 µm. To compare this automated mfIHC-based prognosis marker assessment with the frequently used bright field IHC-based QuPath software package, and the manual prostate identification by pathologists, a cohort of 30 biopsies from routine clinical practice was assessed. An excellent agreement between the mfIHC-based automated cancer detection and manual cancer identification by pathologists was found (intraclass correlation [ICC]: 0.94 [95% CI 0.87 – 0.97]), while the QuPath-based model showed a 20 % deviation from the gold standard (manual assessment by pathologists). Accordingly, the automated mfIHC-based approach was used to search for a prognosis marker panel for routine clinical practice. 5 out of 6 analyzed prognosis markers (PSA, PSMA, INSM1, AR, Ki-67) were significantly liked to biochemical recurrence in univariate analysis (p ≤ 0.021) and 2 of 6 parameters (AR, Ki-67) were independent in multivariate analysis (each p≤ 0.0078). Several prognosis scores of different marker combinations were found that showed strong prognostic relevance in univariate analysis (p < 0.001) and were independent from Gleason groups, pT, pN, serum PSA and R-Status (p = 0.001). Conclusions: Automated prostate cancer identification enables fully automated prognosis marker assessment in routine clinical practice using deep learning and mfIHC.
更多查看译文
关键词
prostate cancer identification,prostate cancer,multiplex fluorescence immunohistochemistry,prognosis markers
AI 理解论文
溯源树
样例
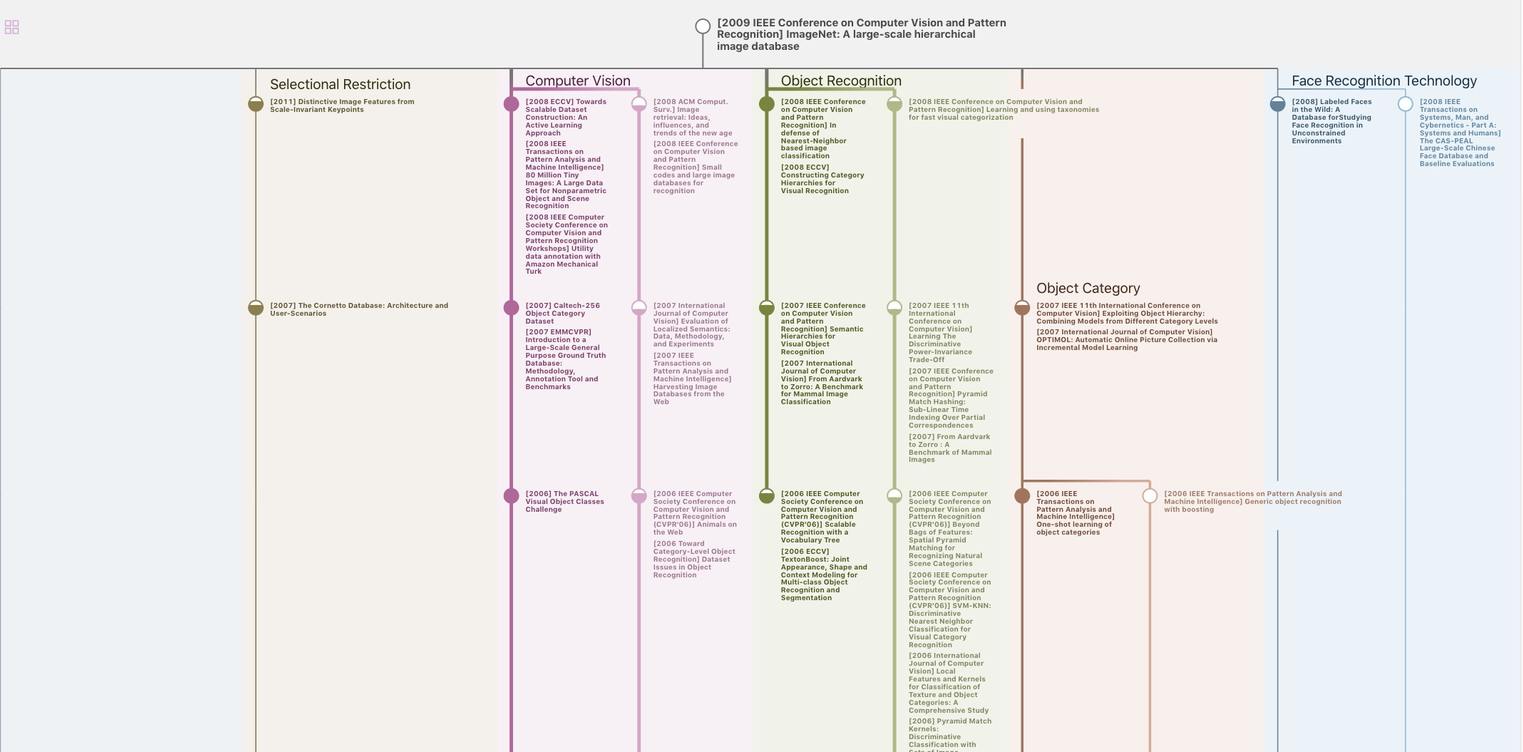
生成溯源树,研究论文发展脉络
Chat Paper
正在生成论文摘要