Development of natural language processing (NLP) models for extracting key features from unstructured notes to create real-world data (RWD) assets for clinical research at scale
JOURNAL OF CLINICAL ONCOLOGY(2023)
摘要
6607 Background: RWD derived from Electronic Health Records (EHR) has detailed clinical information about patient journeys that can assist in clinical research, trial design, safety assessments etc. However, much of the vital information is locked away in unstructured clinical texts and needs to be converted to structured format to be useful for downstream applications. We demonstrate how this can be achieved at scale with a high degree of accuracy through NLP. Methods: NLP models were developed to extract data for 11 clinical variables from unstructured notes of ~98k lung cancer patients and merged with the structured data into a common data model (Table). These models were a combination of domain knowledge, rule-based models, machine learning models, and deep learning models. The increase in fill rate per variable over structured data only was used to quantify the improvement by NLP. The accuracy of the models was assessed against a manually curated dataset comprising of 752 patients. Results: The NLP models significantly improved the fill rate of key clinical variables and were able to extract the information from clinical notes with high accuracy (Table). For some variables such as NSCLC/SCLC status, surgery, tumor grade and histology, all or most of the data was extracted via NLP. Metastatic status via NLP included distant metastasis, locally advanced disease and no metastasis whereas in the structured data, only data for distant metastasis was present. In the case of Performance Status (PS), even though a significant number of patients had at least 1 PS recorded in the structured data, NLP significantly increased longitudinal capture, thus increasing the density of this variable per patient. Conclusions: NLP models can be developed and used to enrich structured RWD data by extracting information from unstructured documents thus significantly improving the utility of this data for downstream applications. Given the high accuracy of these models and the scale at which they can be run, this can be a good alternative to human curation or can augment human curation enabling the creation of very large-scale datasets for clinical research. [Table: see text]
更多查看译文
关键词
unstructured notes,natural language processing,nlp,clinical research,real-world
AI 理解论文
溯源树
样例
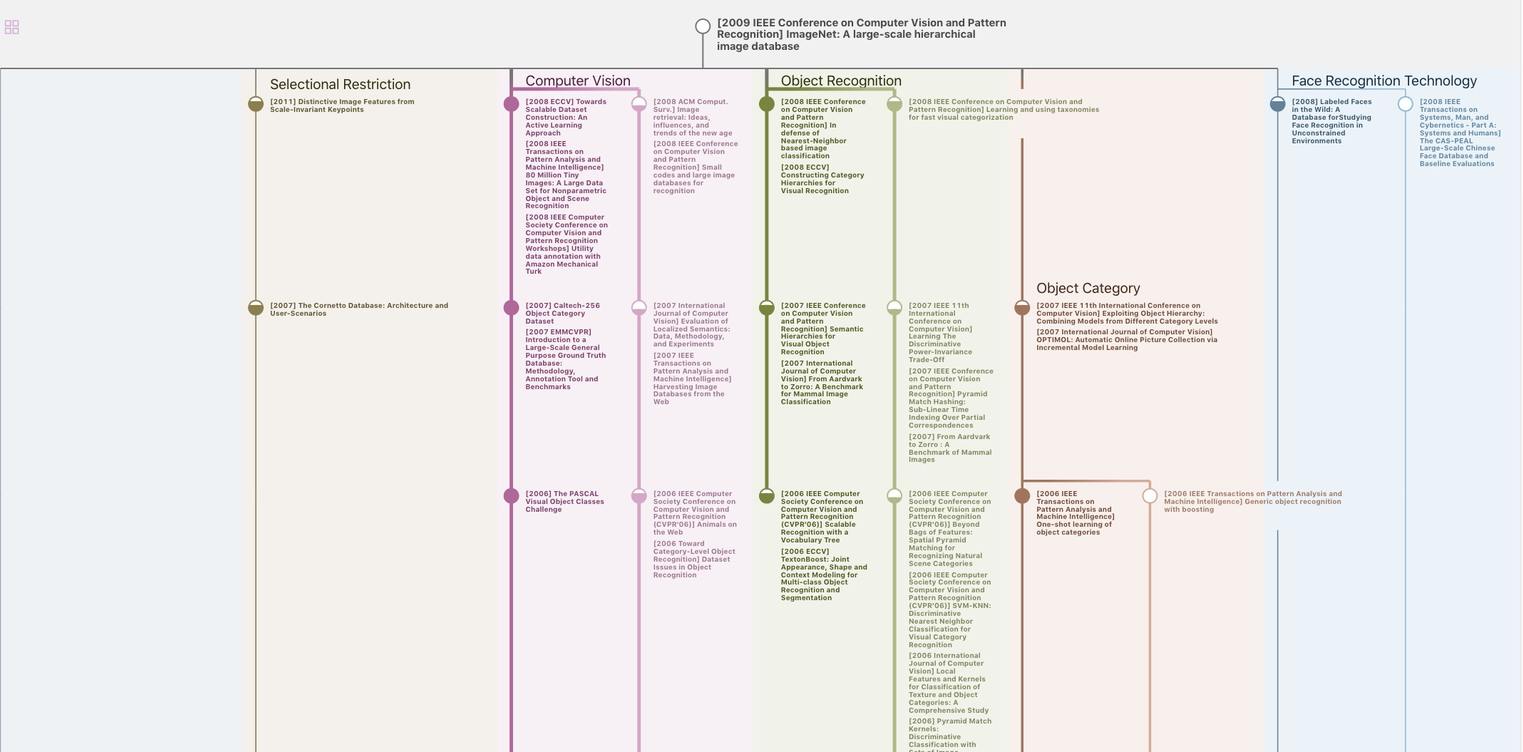
生成溯源树,研究论文发展脉络
Chat Paper
正在生成论文摘要